HSNet: Crowd counting via hierarchical scale calibration and spatial attention
ENGINEERING APPLICATIONS OF ARTIFICIAL INTELLIGENCE(2024)
摘要
Crowd counting has made great progress in recent years, however, problems such as sharp scale variation and background noise still seriously affect counting accuracy. To address the above two deep-rooted challenges, we purposefully propose a novel and robust network called Hierarchical Scale Calibration and Spatial Attention Network (HSNet). HSNet is composed of two key components: the Scale Diversity Enhancer (SDE) and the Spatial Position Focuser (SPF). Additionally, we adopt the pyramid vision Transformer (Twins-SVT) as backbone, which addresses the fact that CNNs are inherently limited by the local attention-receiving fields and unable to model long-term dependencies. Specifically, the SDE adopts a cross-layer strategy to extract the multi-scale features at different levels of the network and concatenates them gradually, which can enrich the scale diversity and alleviate the limitations arising from scale variation. In addition, the foreground (head region) is the most vital marker for crowd counting, the SPF embeds location information into channel attention to precisely enhance the focus on head regions, which significantly mitigates the negative effects of background noise. Intuitively, SPF mitigates the misestimation for background regions. Extensive experiments on four frequently used crowd counting datasets indicate that HSNet has superior performance in counting accuracy compared with the other state -of -the -art methods.
更多查看译文
关键词
Crowd counting,Scale diversity enhancer,Spatial position focuser,Scale variation,Background noise
AI 理解论文
溯源树
样例
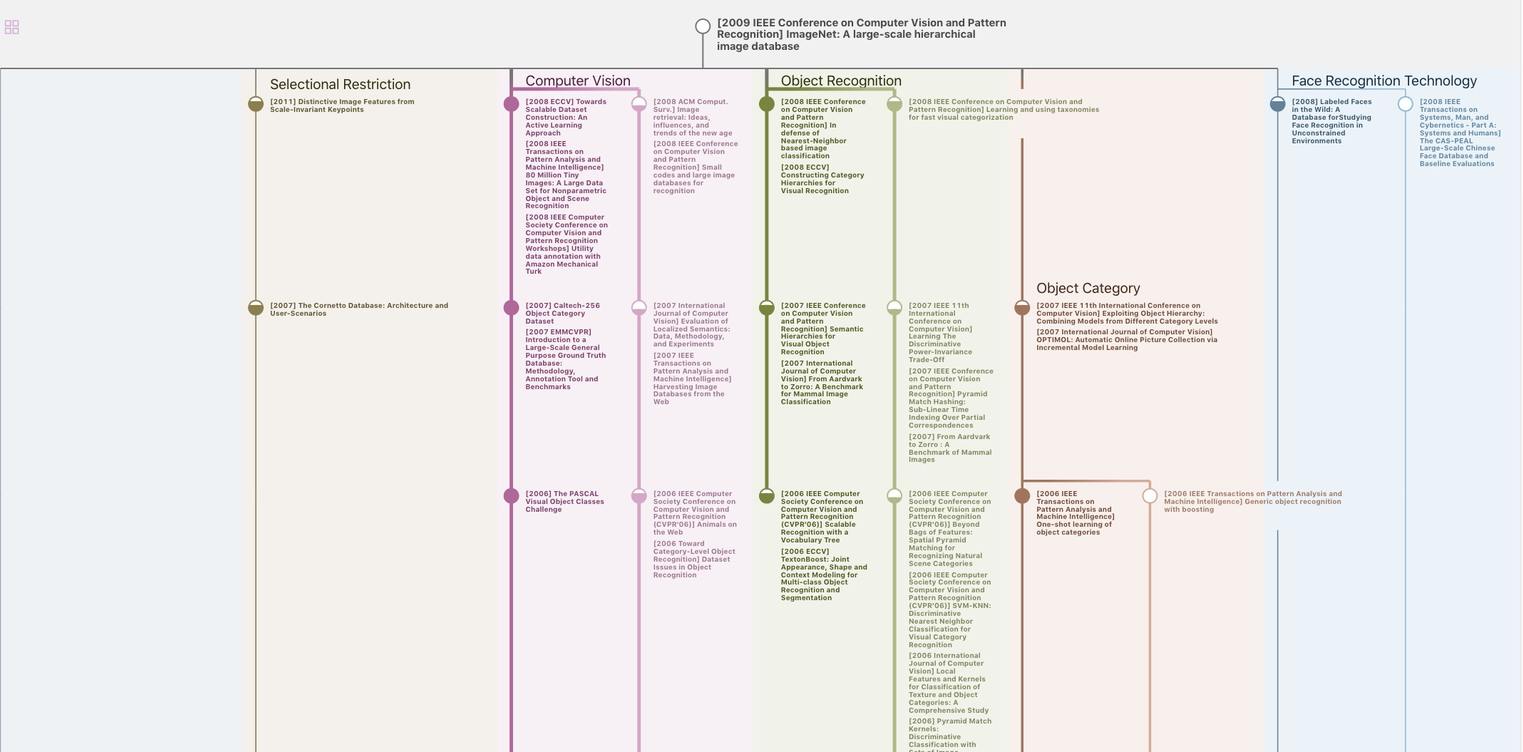
生成溯源树,研究论文发展脉络
Chat Paper
正在生成论文摘要