Robust multi-modal pedestrian detection using deep convolutional neural network with ensemble learning model
Expert Systems with Applications(2024)
摘要
In the realm of video surveillance systems, pedestrian detection (PD) is a crucial task that enables the identification and tracking of individuals, crowd monitoring, and people counting. However, accurate PD is a challenging task due to variations in the appearance and pose of pedestrians. With the advent of deep learning (DL) techniques, particularly convolutional neural networks (CNNs), computer vision (CV) methods for object classification and detection have been revolutionized. Effective PD depends on supervised learning, which requires large labeled datasets. This paper, we present pedestrian detection (PD) using a Robust Multi-modal Pedestrian Detection using a Deep Convolutional Neural Network with an Ensemble Learning (RMPD-DCNN-EL) model. This model effects computer vision and ensemble learning to accomplish precise pedestrian detection. The SimAM EfficientNet model with a stochastic gradient descent (SGD) optimizer is used for feature extraction, while ensemble classification is performed using three DL models are Nested Long Short-Term Memory (NLSTM), deep belief networks (DBNs), and extreme learning machine (ELM). To evaluate the effectiveness of tettapproach, we use the INRIA dataset, and the experimental results prove the greater performance of RMPD-DCNN-EL over other DL methods. The proposed method enables robust pedestrian detection despite modifications in arrival and pose, making it an operative solution for video surveillance systems in smart transport environments. Experimental results show that the detection accuracy is 99.30% of the proposed algorithm is considerably higher than that of the traditional convolutional neural network algorithm.
更多查看译文
关键词
Pedestrian detection,Deep learning,Ensemble learning,Video surveillance,Computer vision
AI 理解论文
溯源树
样例
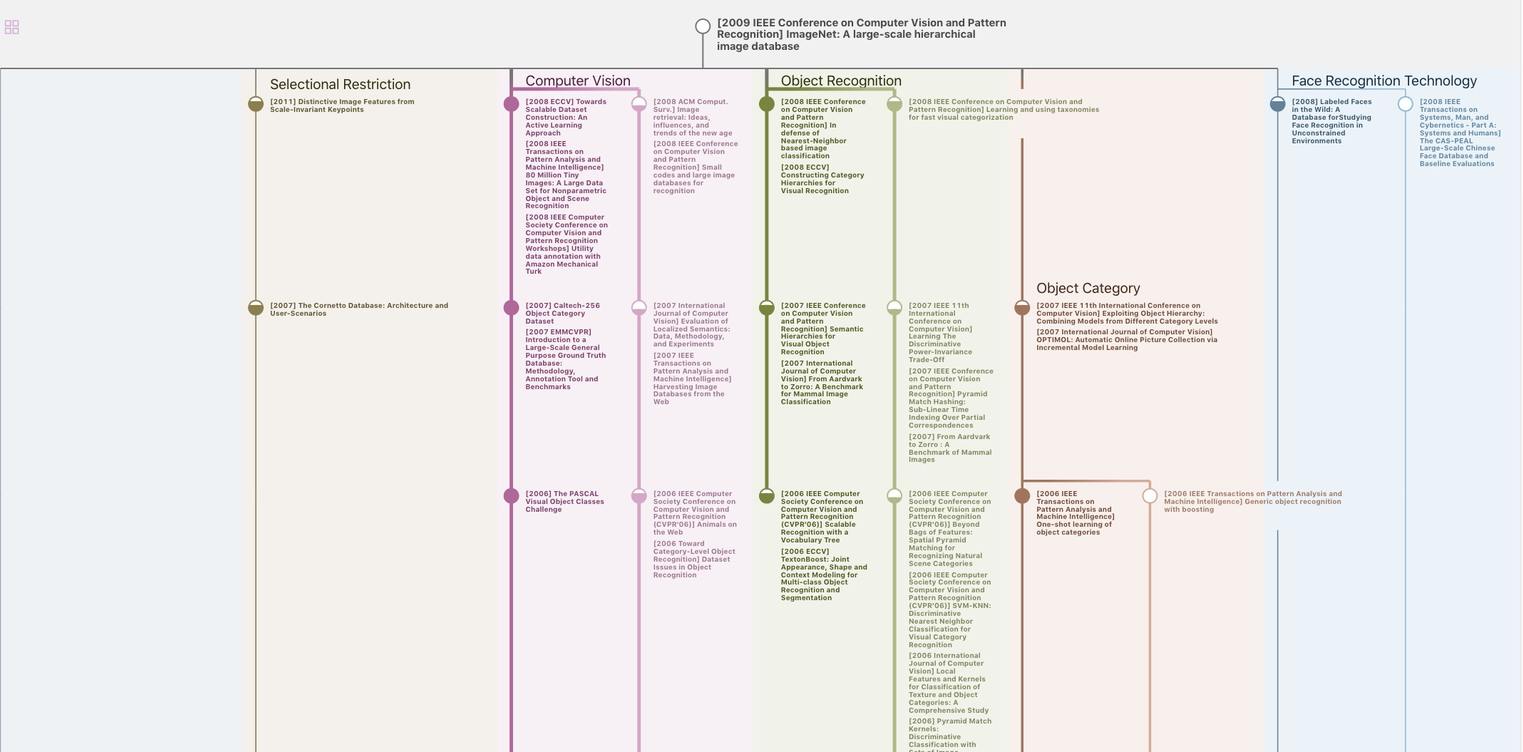
生成溯源树,研究论文发展脉络
Chat Paper
正在生成论文摘要