LEFMIFS: Label enhancement and fuzzy mutual information for robust multilabel feature selection
Engineering Applications of Artificial Intelligence(2024)
摘要
Feature selection is one of the quite important preprocessing steps in multilabel learning. However, multilabel feature selection is facing big challenges due to high-dimensional and noise-contaminated data. In addition, most existing multilabel feature selection methods mainly center around the assumption of uniform label distribution. Meanwhile, the difference in significance of the labels associated with the sample is ubiquitous in many real-world applications. Motivated by these, this paper proposes a robust multilabel feature selection algorithm in which label enhancement is embedded into feature selection. Firstly, based on exploring dual-space identifying information, the data distribution of natural neighbors is further discussed, and the original logical label is enhanced to the label distribution form. Furthermore, a robust multilabel β-precision fuzzy rough sets model (MLβPFRS) is constructed. Combining the algebraic view with the information view in MLβPFRS defines a novel multilabel fuzzy entropy, and some properties and relationships are discussed. What’s more, an objective evaluation function is designed to estimate the quality of the features. Finally, a label enhancement and fuzzy mutual information-based robust multilabel feature selection algorithm is proposed. The extensive experiments performed on thirteen publicly available datasets demonstrate that compared with some advanced algorithms, LEFMIFS is not only for obtaining the feature subset with better classification performance but also for maintaining good robustness.
更多查看译文
关键词
Fuzzy rough sets,Multilabel feature selection,Fuzzy mutual information,Label enhancement,Robustness
AI 理解论文
溯源树
样例
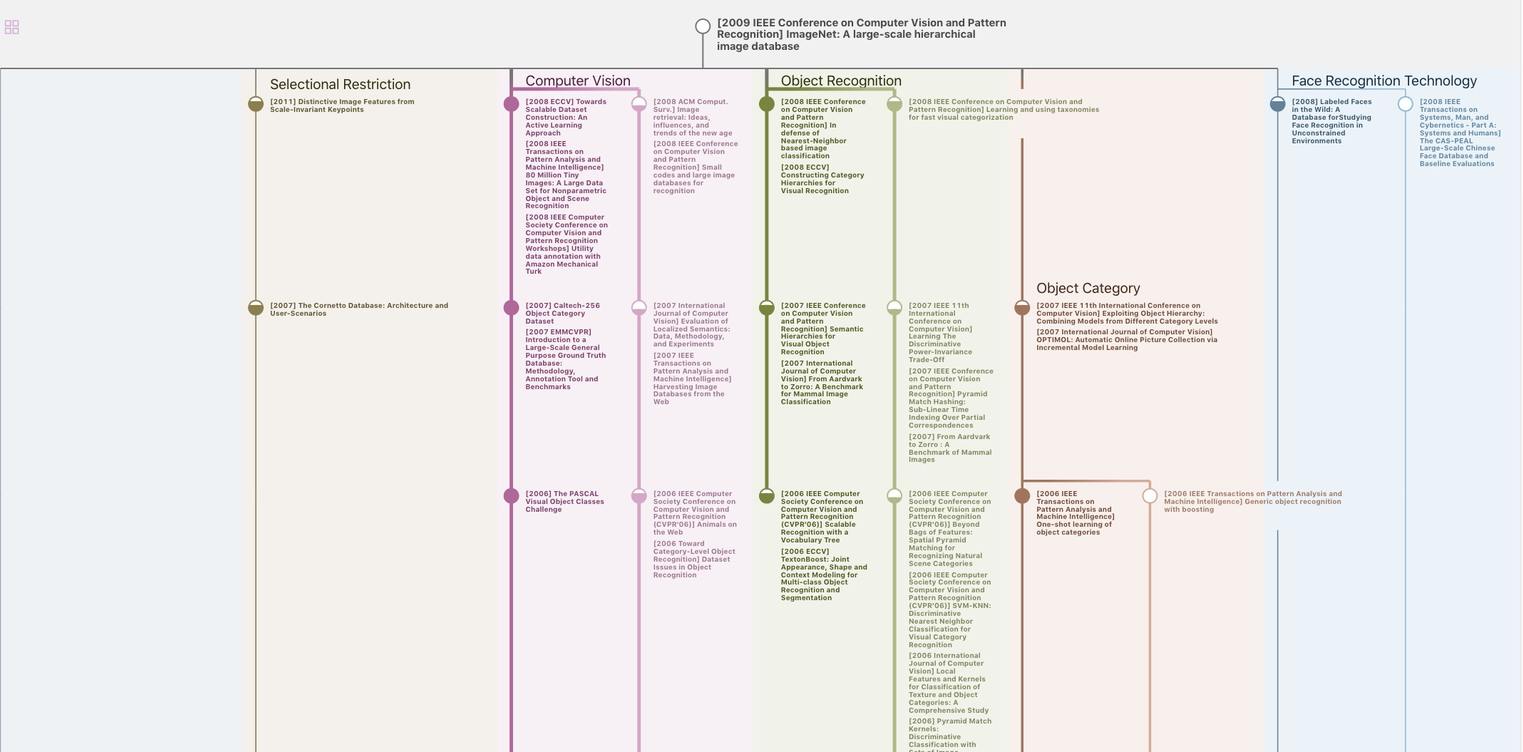
生成溯源树,研究论文发展脉络
Chat Paper
正在生成论文摘要