Gate recurrent unit neural network based high-precision feedforward control for piezoelectric nanopositioning stage
Engineering Applications of Artificial Intelligence(2024)
摘要
Piezoelectric actuators (PEAs) have been widely employed in various high-precision motion scenarios. However, the intrinsic nonlinear characteristics of PEA severely degrade its static accuracy and dynamic tracking performance. Describing these nonlinearities accurately in actual motion systems is nearly impossible, making the realization of precise predictive tracking control very challenging. Neural networks are widely recognized for their strong ability to approximate nonlinearity, making them suitable for control methods in PEA systems. Nonetheless, solely relying on neural networks makes it difficult to predict and compensate for the nonlinear characteristics. Notably, simple linear feedforward compensators can effectively suppress the nonlinearities, achieving satisfactory performance with slightly residual nonlinearities. Based on the above knowledge, this paper proposes a predictive high-precision tracking control based on Gate Recurrent Unit neural network (GRU-NN) and model feedforward compensation methods. Different from other NN-based methods, the proposed approach incorporates a linear model to concentrate the neural network’s efforts on fitting the nonlinear characteristics. Overall, the proposed method has achieved the following advantages compared to other methods: (1) The training requirements of the GRU-NN method have been significantly reduced with a limited dataset and shallow network structure, which enhances the applicability and generalization of the NN-based algorithm. (2) The employment of a linear model feedforward compensator remarkably suppresses the nonlinearities of piezoelectric actuators, and it improves the prediction accuracy of the entire algorithm from around 90% to about 98%. The enhanced prediction accuracy ultimately resulted in improved tracking performance. (3) Compared with most existing control algorithms, the proposed method is hysteresis-model-free with solid generalization ability, and it avoids the sophistication of constructing the nonlinearity of PEA systems. Various comparative experiments are conducted to reveal the effectiveness of the proposed method, and it demonstrates a similar and even better high-precision tracking performance as the benchmark method (iterative learning control, ILC) with solid generalization and applicability capability on PEAs.
更多查看译文
关键词
Piezoelectric actuator,High tracking precision,Hysteresis-model-free,Gated recurrent unit neural network,Feedforword control
AI 理解论文
溯源树
样例
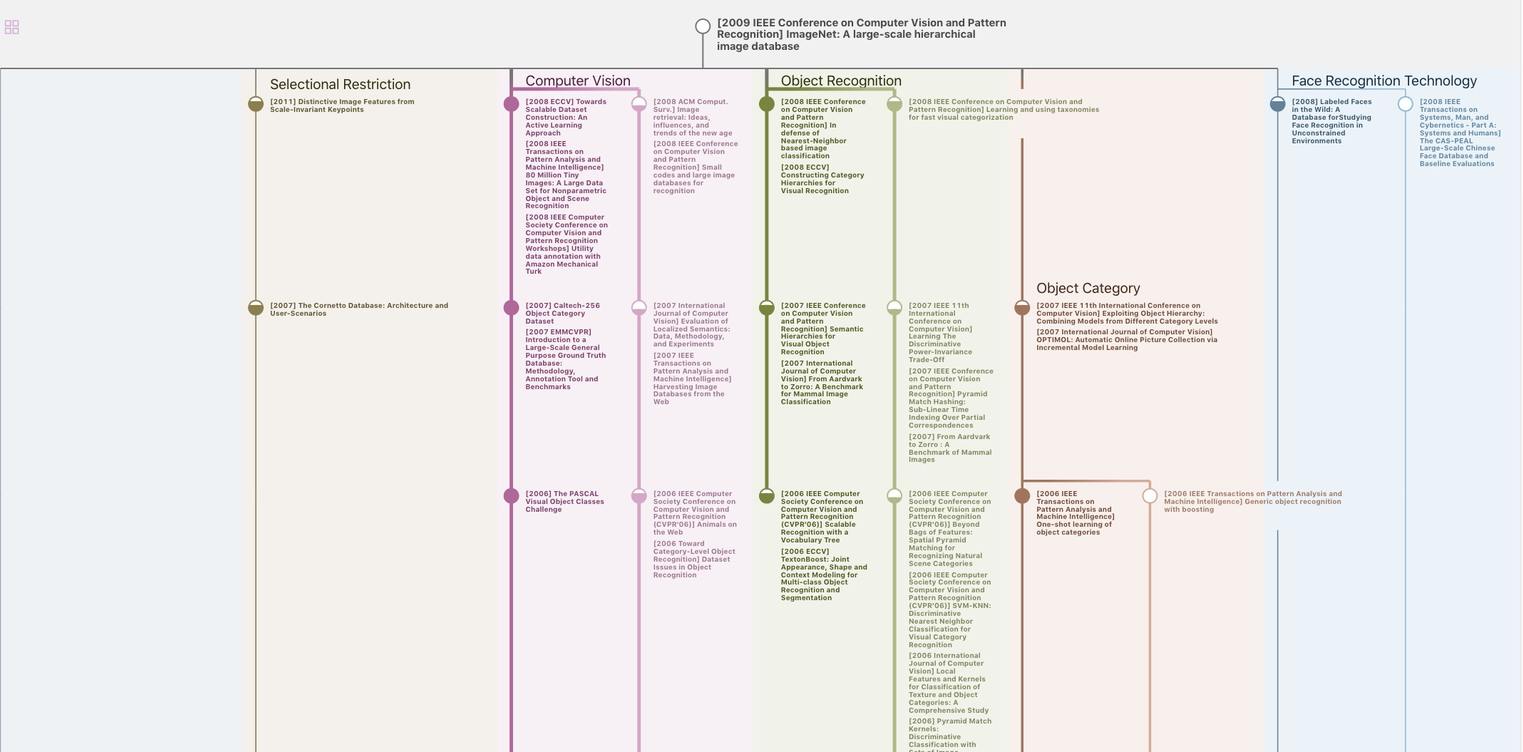
生成溯源树,研究论文发展脉络
Chat Paper
正在生成论文摘要