Deep transfer learning enables battery state of charge and state of health estimation
ENERGY(2024)
摘要
In the realm of lithium-ion battery state estimation, traditional data driven approaches face challenges in accurately estimating state of charge and state of health throughout the battery's life cycle under dynamic working condition, and there is still a lack of research on models that can fulfill these requirements simultaneously. To address these issues, this study proposes an adaptive convolutional gated recurrent unit with Kalman filter for state of charge estimation throughtout battery's full life cycle, leveraging transfer learning and deep learning techniques. Additionally, an adaptive convolutional gated recurrent unit with average post-processor is developed to estimate the battery state of health under dynamic working conditions, using voltage, current, temperature, state of charge, and accumulated discharge capacity as input features. Furthermore, a joint adaptive deep transfer learning model is proposed for simultaneously state of charge and state of health estimation through battery's full life cycle under dynamic working conditions. Experimental results validate the feasibility, accuracy, and robustness of the proposed models.
更多查看译文
关键词
Lithium-ion battery,State of charge,State of health,Joint estimation,Deep learning,Transfer learning
AI 理解论文
溯源树
样例
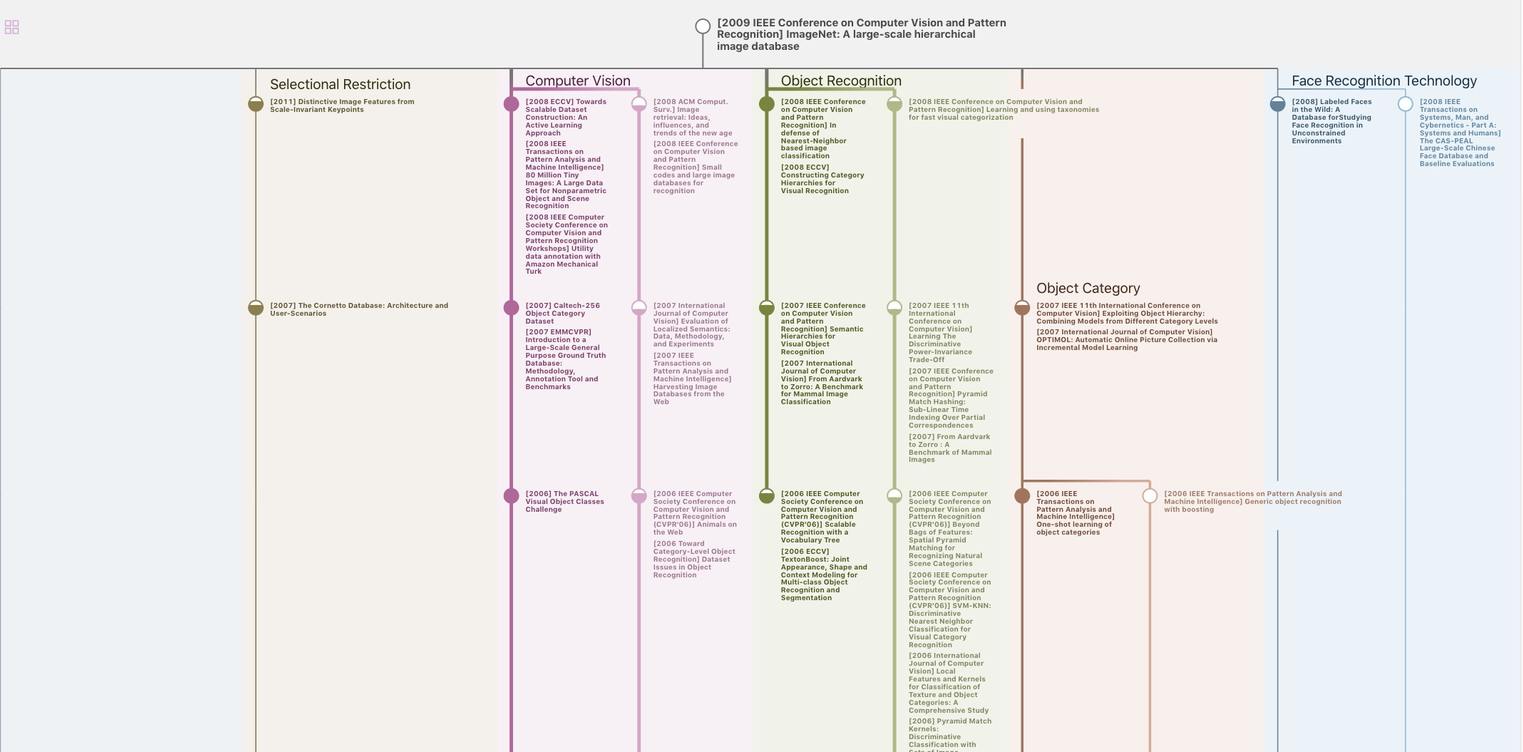
生成溯源树,研究论文发展脉络
Chat Paper
正在生成论文摘要