An empirical wavelet transform-based approach for motion artifact removal in electroencephalogram signals
Decision Analytics Journal(2024)
摘要
Motion artifacts reduce the quality of information in the electroencephalogram (EEG) signals. In this study, we have developed an effective approach to mitigate the motion artifacts in EEG signals by using empirical wavelet transform (EWT) technique. Firstly, we decompose EEG signals into narrowband signals called intrinsic mode functions (IMFs). These IMFs are further processed to suppress the artifacts. In our first approach, principal component analysis (PCA) is employed to suppress the noise from these decomposed IMFs. In the second approach, the IMFs with noisy components are identified using the variance measure, which are then removed to obtain the artifact-suppressed EEG signal. Our experiments are conducted on a publicly available Physionet dataset of EEG signals to demonstrate the effectiveness of our approach in suppressing motion artifacts. More importantly, the IMF-variance-based approach has provided significantly better performance than the EWT-PCA based approach. Also, the IMF-variance based approach is computationally more efficient than the EWT-PCA based approach. Our proposed IMF-variance based approach achieved an average signal to noise ratio (ΔSNR) of 28.26 dB and surpassed the existing methods developed for motion artifact removal.
更多查看译文
关键词
Empirical wavelet transform (EWT),Motion artifact,Intrinsic mode function (IMF),Electroencephalogram (EEG),Principal component analysis,Noise removal
AI 理解论文
溯源树
样例
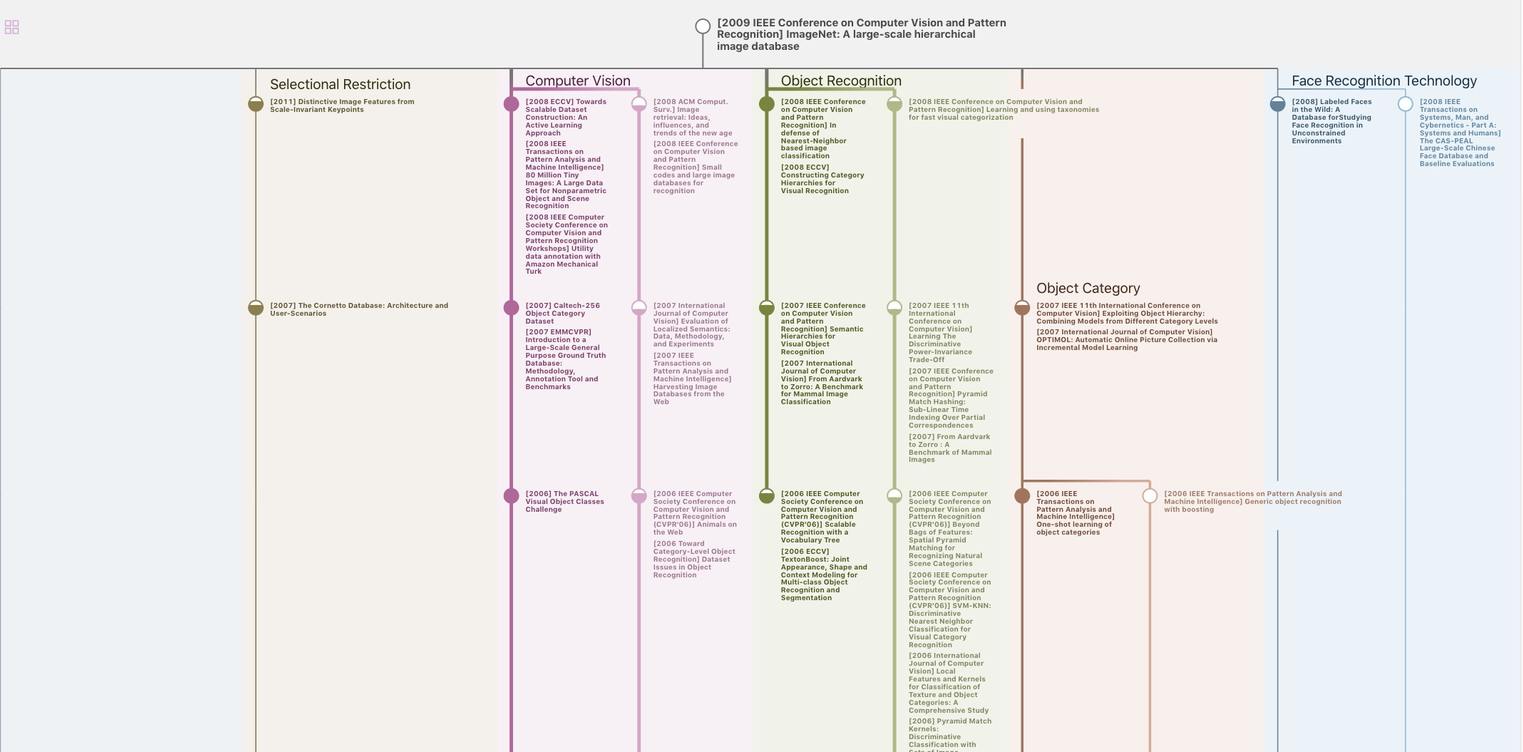
生成溯源树,研究论文发展脉络
Chat Paper
正在生成论文摘要