An investigation of the exposure effect of recommender systems in hospitality
Decision Analytics Journal(2024)
摘要
This study aims to investigate the role of a recommendation system (RS) in personalizing the guest journey in the hospitality industry and its impact on decision making, particularly the exposure effect. The study has two main objectives: (a) to quantify the additional value of using a RS based on an empirical dataset and (b) to explore how to leverage the exposure effect based on a simulated dataset to increase the number of services chosen by guests. The study compares machine learning models and naive strategies using several evaluation metrics to evaluate the added value. The study uses an empirical case study containing over 33,000 reservations to compare the effectiveness of different methods. We show that the eXtreme Gradient Boosting (XGBoost) model outperforms other approaches based on accuracy (0.905), AUC (0.908), and simulated revenue. The study also employs a reinforcement learning approach, as an extension of a RS, to quantify the exposure effect and leverage it to increase the number of services chosen by guests. The approach is found to improve the number of services chosen by 7.60% compared to a RS without this extension in a simulation environment.
更多查看译文
关键词
Recommender systems,Machine learning,Exposure effect,Hospitality,Reinforcement learning
AI 理解论文
溯源树
样例
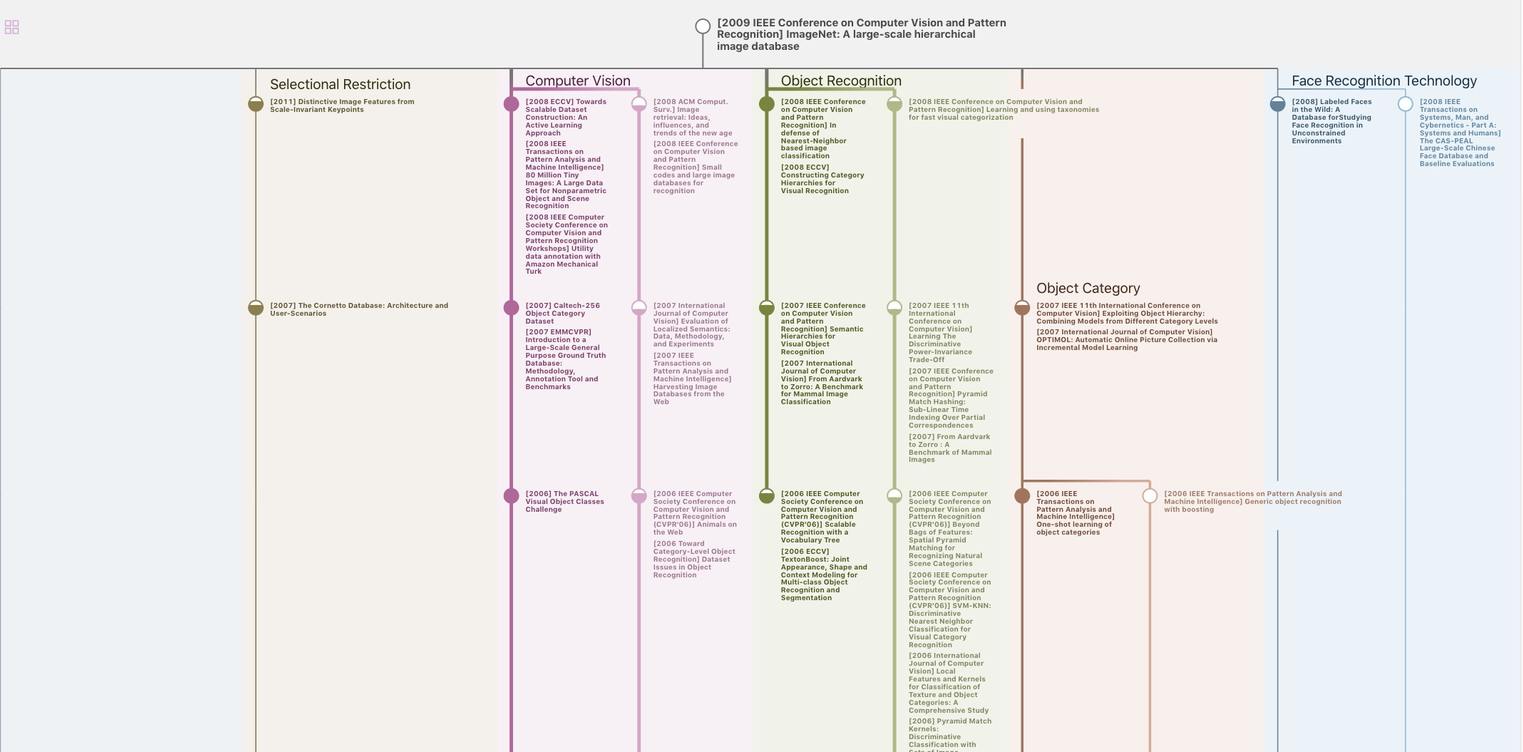
生成溯源树,研究论文发展脉络
Chat Paper
正在生成论文摘要