TABDeep: A two-level action branch architecture-based deep reinforcement learning for distributed sub-tree scheduling of online multicast sessions in EON
Computer Networks(2024)
摘要
The surge in demand for multimedia applications has brought a great pressure on network bandwidth resources. In order to improve the efficiency of bandwidth resource usage and ensure the transmission quality of multicast sessions, it is very urgent to effectively schedule online multicast sessions. To do so, in this paper, we study the distributed sub-tree scheduling problem of online multicast sessions in elastic optical network (EON). First, we model the problem as a Markov Decision Process (MDP) by defining state, action and reward. Second, a two-level action branch architecture-based deep reinforcement learning (TABDeep) algorithm is proposed to realize the destination node grouping, source node selection, route establishment and spectrum assignment of multicast sessions. Finally, to test the superiority of TABDeep, we construct a series of simulation experiments to compare TABDeep with a link-aware distributed steiner sub-tree benchmark algorithm (LA-DSST). The experimental results confirm that TABDeep performs better than LA-DSST in reducing the blocking probability of the session.
更多查看译文
关键词
Deep reinforcement learning,Distributed Sub-tree Scheduling,Elastic optical network
AI 理解论文
溯源树
样例
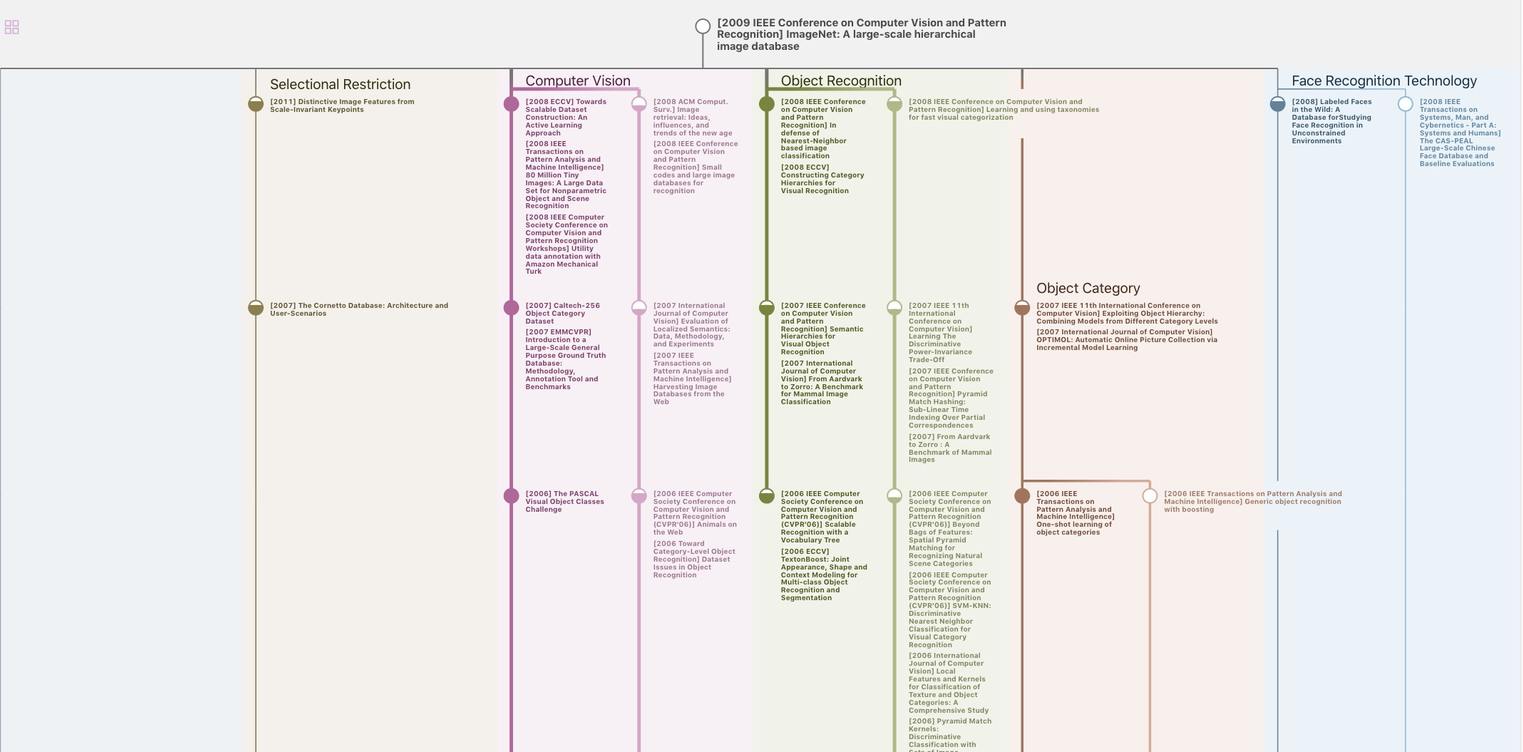
生成溯源树,研究论文发展脉络
Chat Paper
正在生成论文摘要