Machine learning-based prediction of outdoor thermal comfort: Combining Bayesian optimization and the SHAP model
Building and Environment(2024)
摘要
Rising global temperatures have resulted in urban heat waves in recent years, endangering residents' health and even their lives. As a result, accurate outdoor thermal comfort prediction is critical. The goal of this research is to develop highly accurate and interpretable machine learning models of outdoor thermal comfort. We created a summer outdoor thermal comfort dataset for cold regions using microclimate parameter measurements and questionnaires, and divided it into two datasets: without and with shading. The prediction performance of nine machine learning models was compared, as well as their prediction performance following Bayesian optimization. Finally, the SHAP model was used to explain the important features of the best machine learning models and their impact on thermal comfort. The results show that after partitioning the dataset by shading, the accuracy of the best machine learning prediction models for Thermal sensation vote (TSV), Thermal acceptable vote (TA), and Thermal comfort vote (TCV) in the unshaded space improves by 9.2%, 9.31%, and 6.16%, respectively, while the prediction accuracy in the shaded space remains basically unchanged. Extreme gradient boosting (XGBoost), Light Gradient Boosting Machine (LightGBM), and categorical boosting (CatBoost) outperform other methods. After Bayesian optimization (BO), the machine learning models' TSV, TA, and TCV prediction accuracies improved by 6.83%, 4.05%, and 2.55%, respectively. CatBoost model with Bayesian optimization (CatBoost + BO) was the best model in most cases. TSV, TA, and TCV predictions were heavily influenced by microclimate parameters, with mean radiant temperature being the most important in unshaded spaces and air temperature being critical in shaded spaces. Furthermore, age, body mass index, and emotional state all had a significant impact on TA and TCV. This research will contribute to improving the quality of life of urban residents by providing a scientific foundation for the design and planning of urban open space.
更多查看译文
关键词
Outdoor thermal comfort,Urban open space,Machine learning,Bayesian optimization,SHapley additive exPlanations
AI 理解论文
溯源树
样例
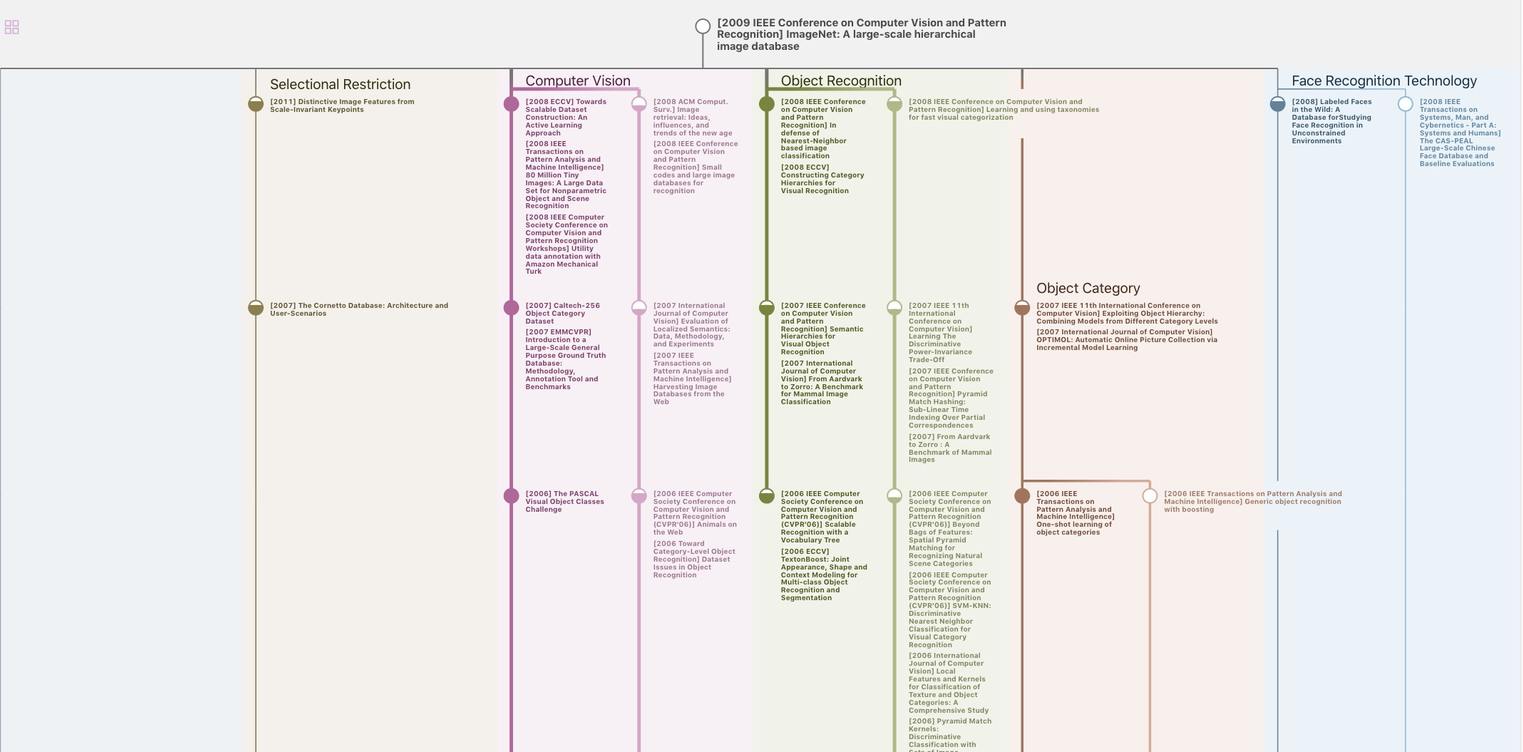
生成溯源树,研究论文发展脉络
Chat Paper
正在生成论文摘要