Quality assessment of view synthesis based on unsupervised quality-aware pre-training
APPLIED SOFT COMPUTING(2024)
摘要
The current view synthesis quality metrics mainly rely on hand-crafted features, which have clear physical meanings but fail to comprehensively describe the complex distortion characteristics in synthesized images. With the rapid advancement of deep models, convolutional neural networks hold great promise for learning complex distortion representations. The exceptional performance of deep learning is closely tied to the availability of abundant labeled training data. However, manual annotation of labels for quality assessment tasks is arduous, which impede the application of deep learning in this area. With this motivation, this paper presents a no -reference quality assessment model for view synthesis based on unsuperviseD quality -Aware Pre -Training (DAPT). Specifically, a two -stream network with spatial destruction sensitivity and adaptive heterogeneous awareness branches is firstly designed, and then the two branch networks are pre -trained unsupervised to fully extract the quality -aware feature representations. Finally, a multi -layer perceptron is utilized to generate quality scores based on the spatial domain destruction and structural damage information. Notably, to better align the quality -aware features learned through unsupervised pre -training in the source domain with those of the target domain, we introduce a domain adaptive module in the adaptive heterogeneous awareness branch. Extensive experiments demonstrate that the proposed DAPT model outperforms the state -of -the -arts.
更多查看译文
关键词
View synthesis,Quality assessment,Unsupervised pre-training,Domain adaptive
AI 理解论文
溯源树
样例
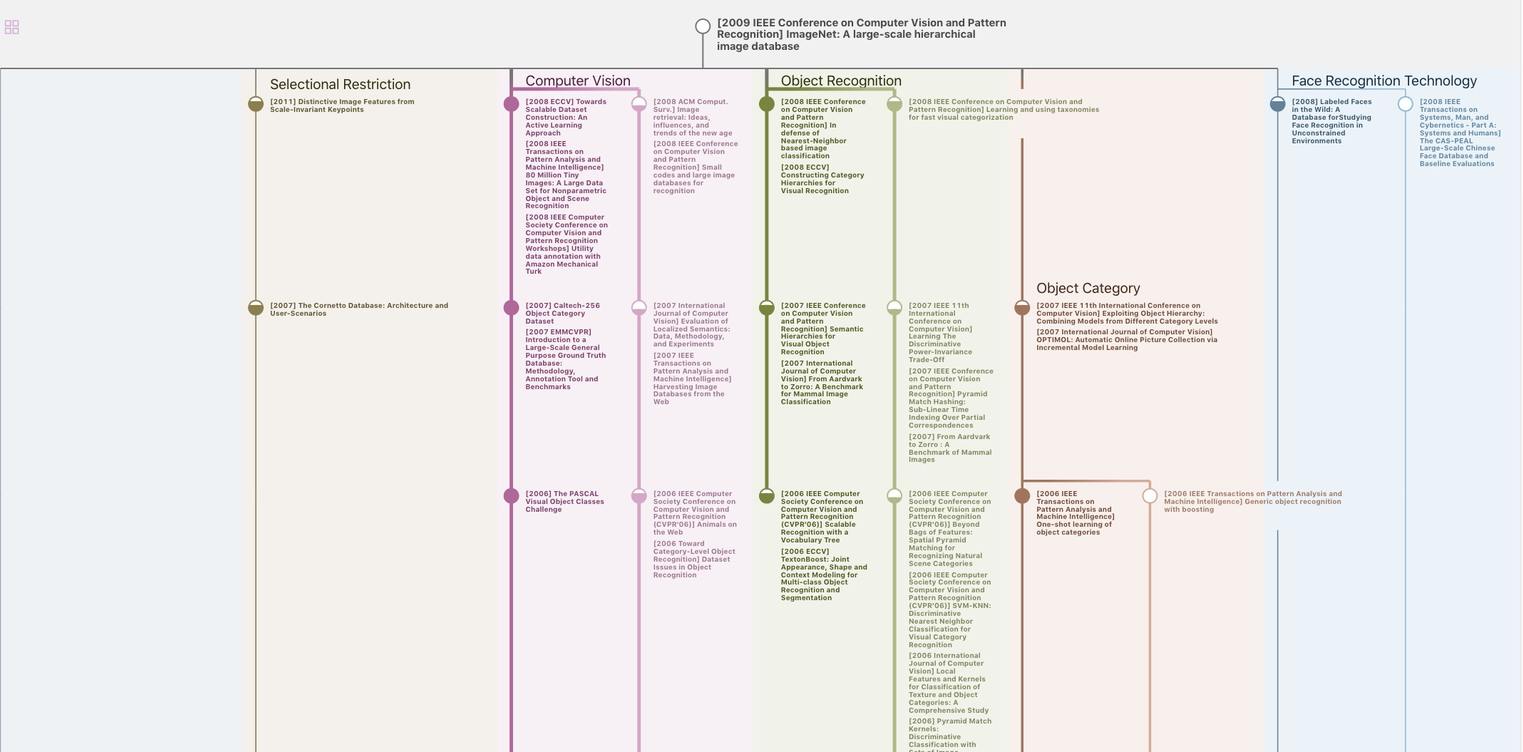
生成溯源树,研究论文发展脉络
Chat Paper
正在生成论文摘要