Relevance variable selection variational auto-encoder network for quality-related nonlinear process monitoring
APPLIED SOFT COMPUTING(2024)
摘要
Quality -related process monitoring is essential for revealing changes in product quality and ensuring industrial safety. Therefore, it is crucial to distinguish enough quality -related features within the data. To learn the nonlinear characteristics and obtain quality -related features in process data, a novel process monitoring method named relevance variable selection variational auto -encoder (RVS-VAE) is proposed. Firstly, to enhance the strong quality -related variables and learn the time correlation, the normalized original data is weighted by mutual information and augmented into data matrices. Secondly, the RVS strategy is proposed to select the most quality -related variables from the latent features. These quality -related features are further utilized to monitor the process. What is more, when a fault is detected, relevance variable relative contribution (RVRC) method is presented to locate the fault variables. Finally, performance of the proposed RVS-VAE method is evaluated through comparative experiments on tennessee eastman (TE) process.
更多查看译文
关键词
Variable selection,Fault variable localization,Quality-related,Process monitoring,Nonlinear
AI 理解论文
溯源树
样例
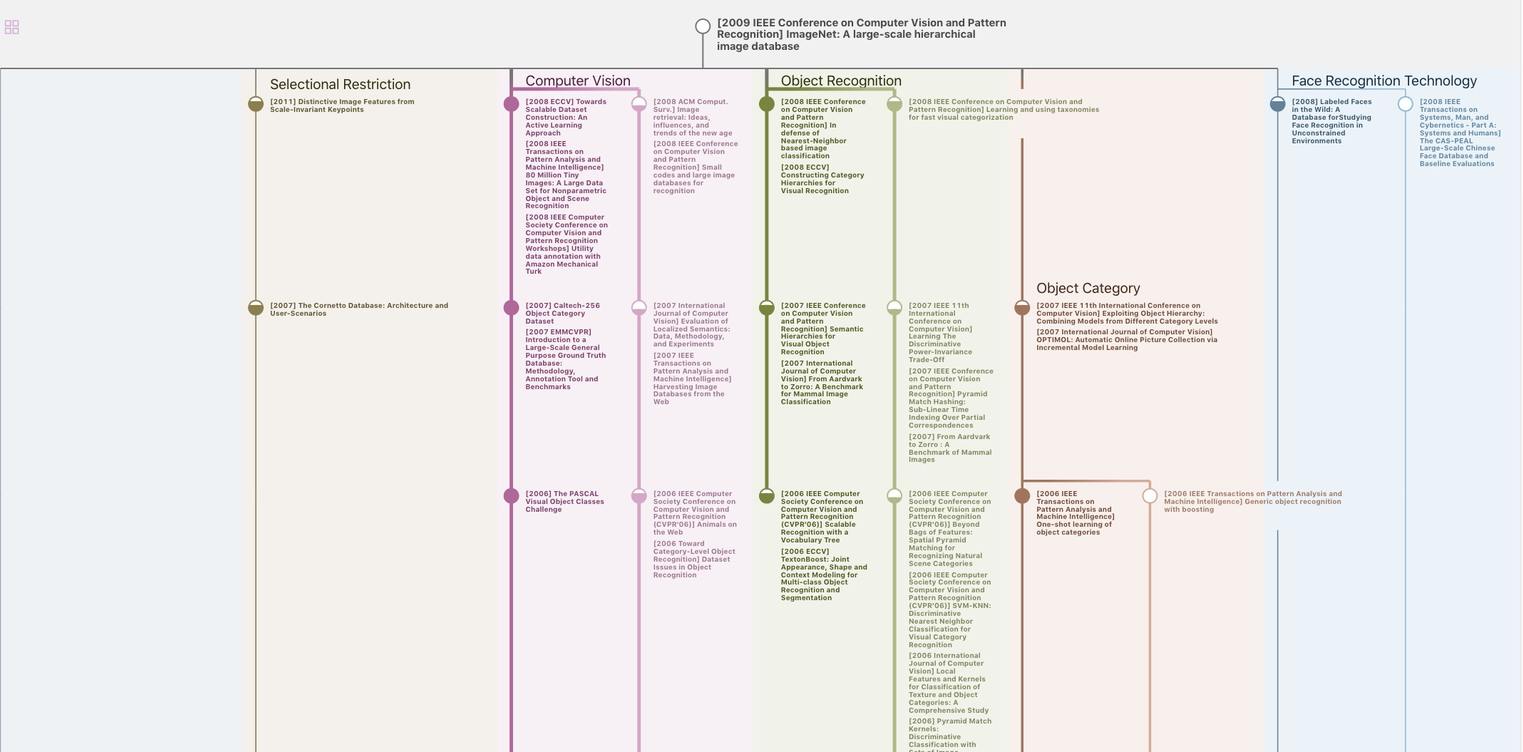
生成溯源树,研究论文发展脉络
Chat Paper
正在生成论文摘要