Unsupervised feature selection using chronological fitting with Shapley Additive explanation (SHAP) for industrial time-series anomaly detection
Applied Soft Computing(2024)
摘要
With the development of the industrial Internet of Things (IIOT), an amount of industrial multivariate time series (IMTS) data has been collected by various sensors. IMTS data anomaly detection plays an important role in industrial process monitoring and operation condition identification. Many real-world IMTS datasets usually have a large number of redundant features, which may lead to deviation of the anomaly detection model and misunderstanding of potential feature correlation. Hence, it is essential to select features that have critical and effective effects on anomalies and operation conditions. In this paper, a feature selection that introduces knowledge-based target variables into inner feature selectors (IFSs) is proposed as a wrapper method, referred to as ILSFS. The IFS is constructed by integrating a timing fitter, which is established based on the long and short-term memory (LSTM) network, with the Shapley Additive explanation (SHAP) assisted to eliminate unimportant features. The experiments were conducted on two datasets: the vertical roller mill dataset (VMD) of a slag milling factory in Jiangsu Province and the public server machine dataset (SMD). Compared with None feature selection, SVM-RFE, LGBM -Boruta, Genetic-based methods, and other SHAP-assisted feature selection methods such as XGBSVFIR and ShapHT+, the experiment results highlighted the proposed methodology ILSFS improves the best F1 score of each the above baselines by 0.2799, 0.2208, 0.2647, 0.1882, 0.3775, 0.2765 for the VMD and 0.1475, 0.0586, 0.0567, 0.0638, 0.0519, 0.2250 for the SMD on the downstream anomaly detection. The best precision of the ILSFS for the VMD and the SMD was 0.8224 and 0.9499, respectively, while its best recall was 0.7353 and 0.4902, respectively.
更多查看译文
关键词
Industrial multivariate time-series data,Unsupervised feature selection,LSTM,SHAP,Anomaly detection
AI 理解论文
溯源树
样例
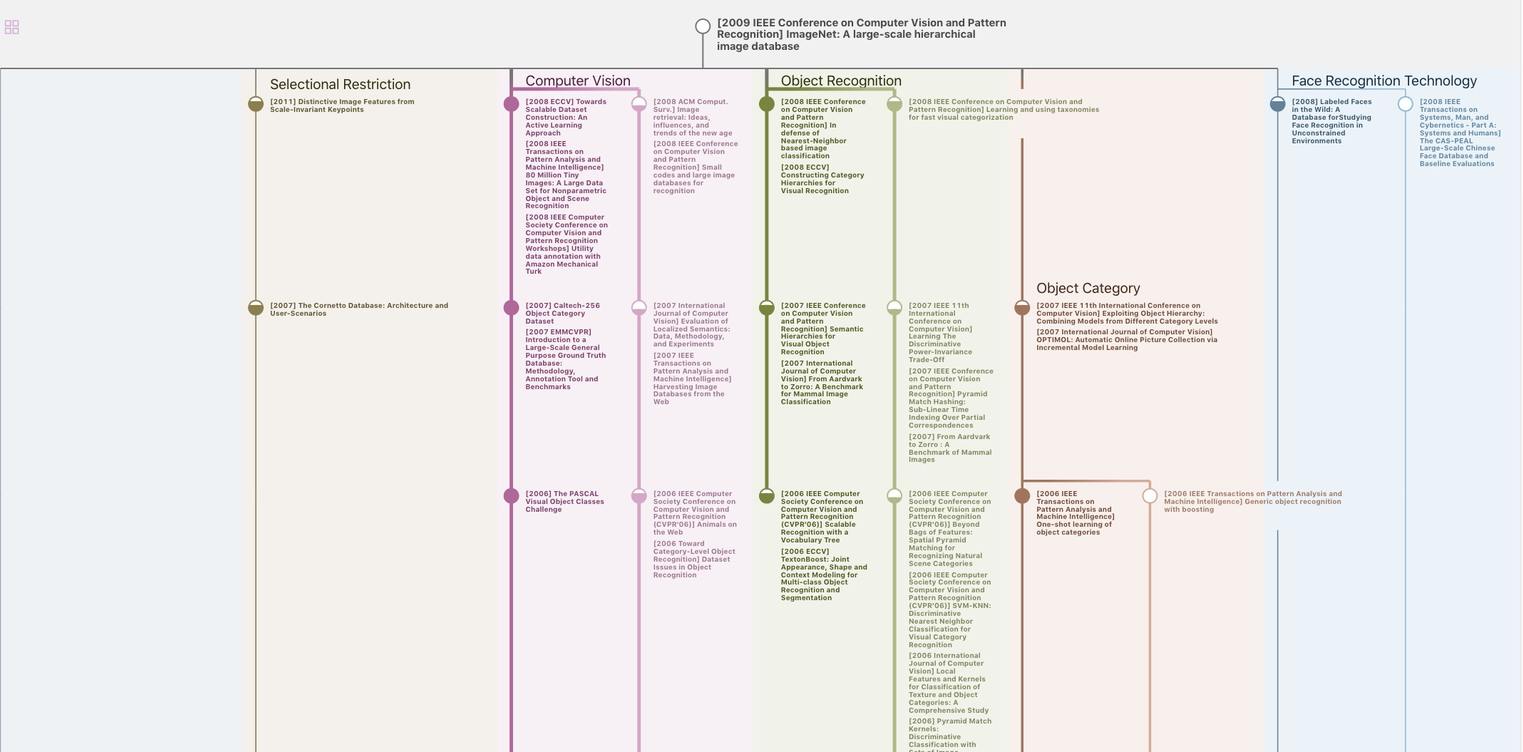
生成溯源树,研究论文发展脉络
Chat Paper
正在生成论文摘要