Like draws to like: A Multi-granularity Ball-Intra Fusion approach for fault diagnosis models to resists misleading by noisy labels
ADVANCED ENGINEERING INFORMATICS(2024)
摘要
Although data -driven fault diagnosis methods have achieved remarkable results, these achievements often rely on high -quality datasets without noisy labels, which can mislead the model into producing inaccurate or overfitting classification boundaries in the latent feature space, leading to a significant decrease in the accuracy or even complete failure. To address this challenge, this study proposes a label correction and sample selection approach named MgBIF. The aim is to enable models to robustly perform supervised learning under unknown ratios of noisy labels and achieve the performance of high accuracy and strong generalization. Specifically, MgBIF first creates an exclusive granular ball for each sample in the latent feature space, then fuses labels from other samples within the granular ball to generate pseudo -labels, which are used to correct the current labels. Subsequently, samples with high -confidence labels are selected from the training data with corrected labels to construct a clean dataset for supervised learning, thus minimizing the misguidance of noisy labels on the model during gradient -based parameter updates. A series of experiments validate the effectiveness and superiority of the MgBIF, which can effectively suppress the negative impact of noisy labels in lownoise environments (achieving an accuracy of over 96%) and significantly improve accuracy in high -noise environments (with a maximum improvement of 80%), outperforming the current state-of-the-art methods. MgBIF, therefore, provides a competitive and robust training strategy for uncertain label noise ratio datasets, which is expected to promote the process of automatic annotators replacing expert annotations to construct datasets in the era of large datasets, thereby helping many practical engineering projects reduce costs and increase efficiency.
更多查看译文
关键词
Industrial time series classification,Noisy labels,Information fusion,Label correction,Sample separation,Granular computing
AI 理解论文
溯源树
样例
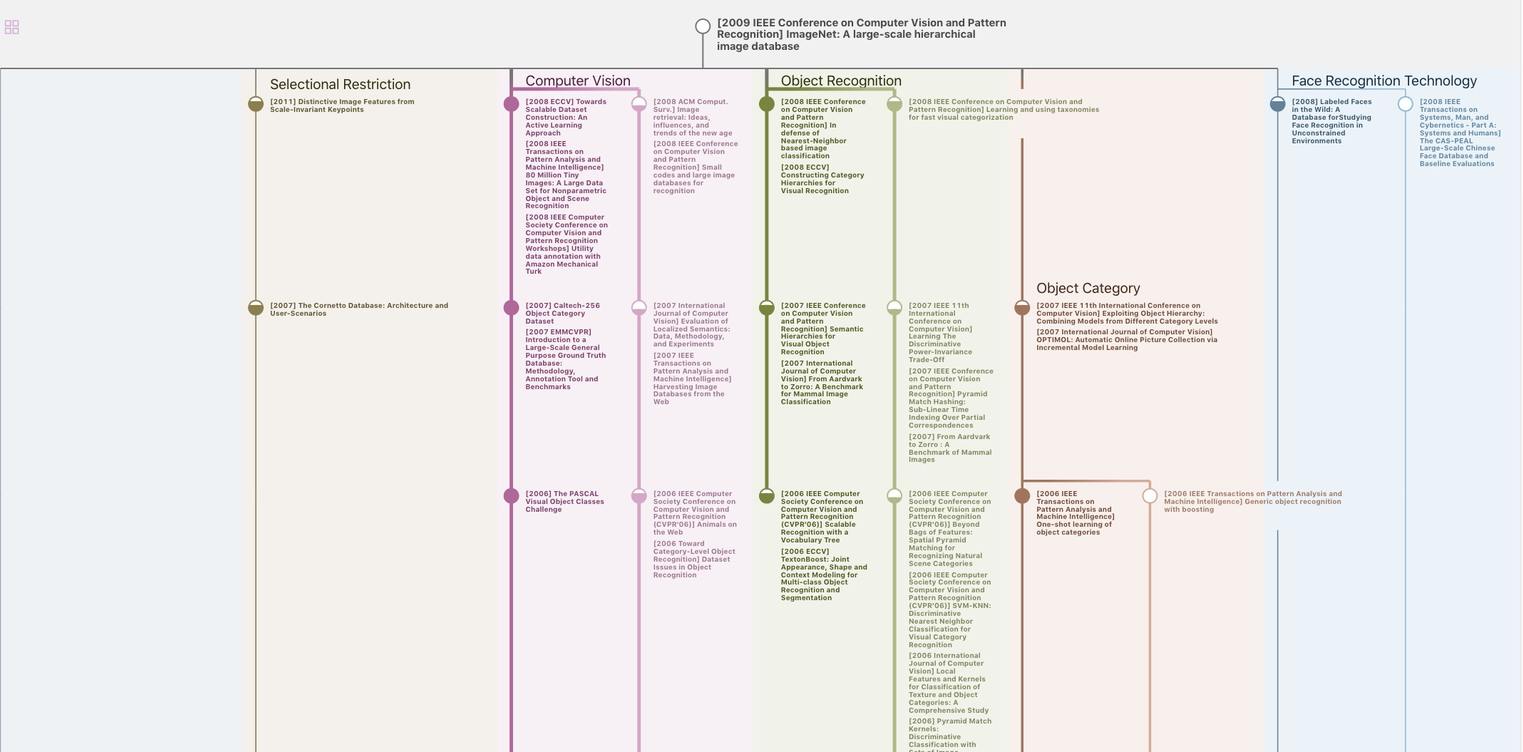
生成溯源树,研究论文发展脉络
Chat Paper
正在生成论文摘要