Indicative Vision Transformer for end-to-end zero-shot sketch-based image retrieval
ADVANCED ENGINEERING INFORMATICS(2024)
摘要
Zero -shot sketch -based image retrieval (ZS-SBIR) has garnered attention for overcoming inconvenience and impracticality of Traditional Image Retrieval (TIR) in the engineering domain. ZS-SBIR can retrieve neverbefore -seen images with sketches, solving the dilemmas of insufficient samples and model retraining. However, existing ZS-SBIR approaches have the following remaining limitations: Firstly, CNN -based methods struggle to capture global features effectively. Secondly, hybrid networks treat sketch and image modalities separately, ignoring the implied feature consistency. Thirdly, non -end -to -end Vision Transformer (ViT) models incur expensive training costs. To solve the above problem, we present an end -to -end retrieval approach, which first extends the ViT through indicative information. The key core of the algorithm is that we propose a feature picker with indicative multi -layer perception. It collectively processes images and sketches with relatively economical consumption, while yielding surprising benefits. To tackle the inherent modal and semantic gaps in ZS-SBIR, we propose a parallel feature adapter. In this adapter, the features are modulated by an identification learning module to generate discriminative information. Next the feature -level smooth alignment is utilized to focus on enhancing the learning of inter -class relationships. In addition, we employ logit-level auxiliary signal to direct the model to capture additional semantic knowledge. Extensive experiments show that the proposed approach significantly outperforms state-of-the-art retrieval methods on Sketchy, Sketchy -No, QuickDraw, and the Tuberlin datasets.
更多查看译文
关键词
Zero-shot sketch-based image retrieval,End-to-end,Feature picker,Feature adapter,Parallel architecture
AI 理解论文
溯源树
样例
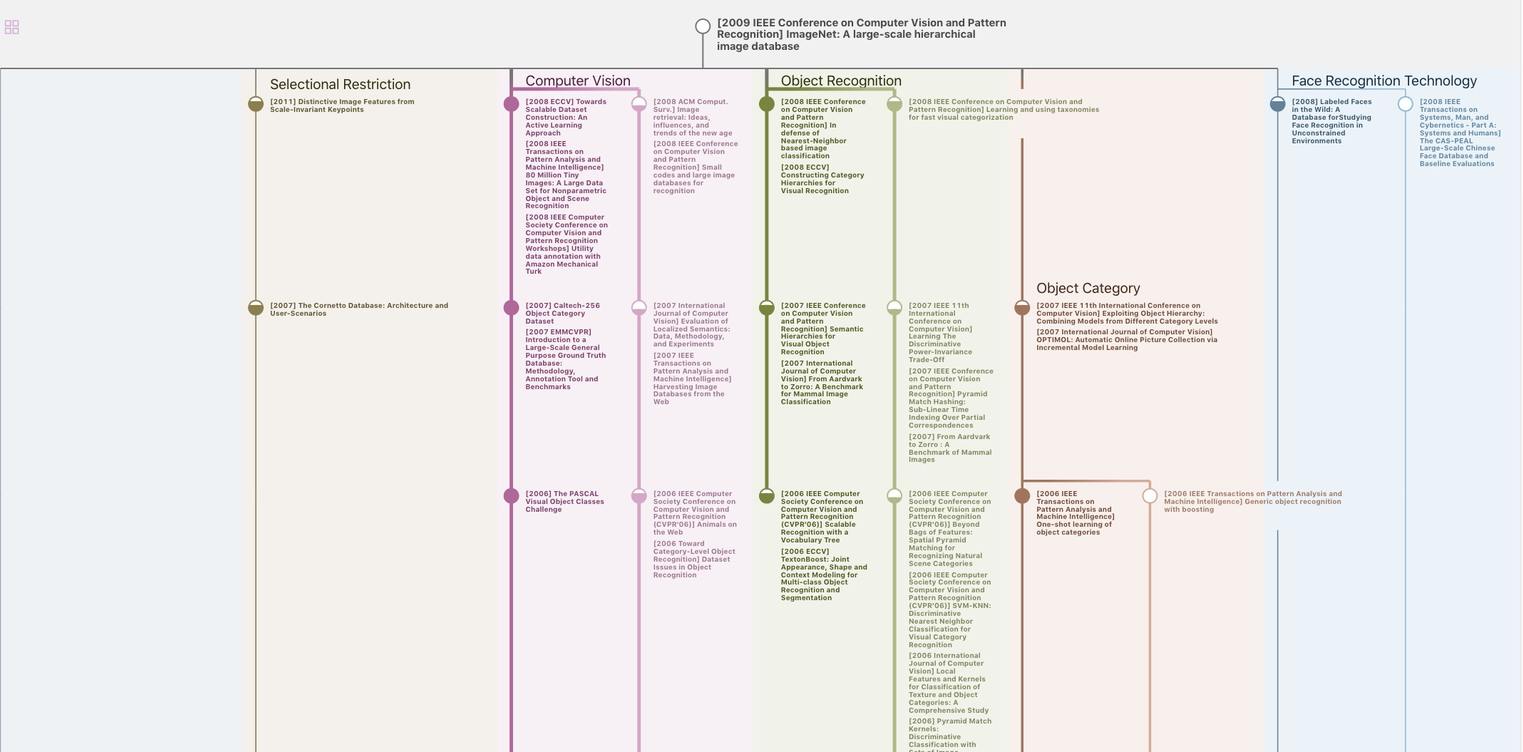
生成溯源树,研究论文发展脉络
Chat Paper
正在生成论文摘要