A knowledge-driven spatial-temporal graph neural network for quality-related fault detection
Process Safety and Environmental Protection(2024)
摘要
The majority of quality-related fault detection methods focused on process statistics, neglecting the spatial-temporal characteristics of variables and the physical information of the process. This research presents a Knowledge-driven Spatial-Temporal Graph Attention Neural Network (K-STGAT) to address the instability of quality-related fault detection due to the existing dynamic and spatial-temporal correlations. First, the measurable variables in the process are transformed into topological graphs based on prior knowledge. Second, construct a spatial-temporal adjacency matrix and spatial-temporal sequence. Third, the sign attention mechanism is introduced into the graph attention networks to capture the propagation patterns of variables in both temporal and spatial dimensions. Finally, the model is trained by reconstructing the variables, and the quality-related variables are selected to construct a quality regression model to obtain the quality-related space and construct the detection statistic. The effectiveness and soundness of the proposed approach are demonstrated through its application to the Tennessee Eastman process.
更多查看译文
关键词
Quality indicator,Process monitoring,Graph attention networks,Spatial-temporal characteristics
AI 理解论文
溯源树
样例
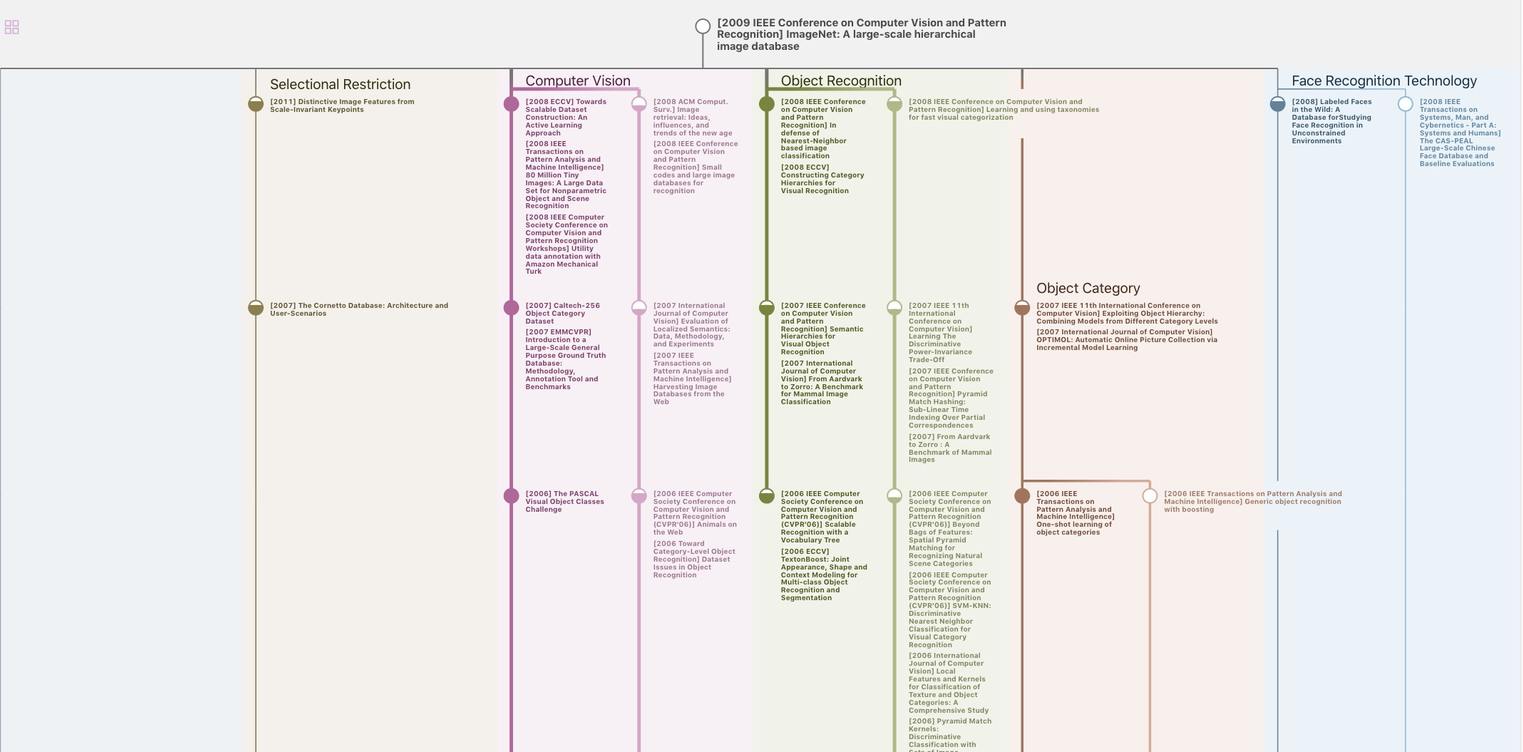
生成溯源树,研究论文发展脉络
Chat Paper
正在生成论文摘要