A knowledge guided deep learning framework for underground natural gas micro-leaks detection from hyperspectral imagery
ENERGY(2024)
摘要
Detecting natural gas (NG) micro-leaks is critical for NG transport and storage. In the event of underground NG leaks in vegetation covered areas, a priori knowledge derived from analyzing vegetation growth mechanisms can effectively describe the degree of vegetation response to NG stress, which is of theoretical importance for the quantitative detection of NG leaks. This study proposed a knowledge-guided deep learning framework and use it for underground NG leaks detection, in which an image and spectrum combined multi-branching deep learning (DL) network (Img-Spec-PGE model) was used to estimate the leaf area index (LAI) of NG-stressed plants, and an improved physical model for describing plant NG stress mechanisms was proposed, then assimilating the SAFY-NG model with LAI as an intermediate variable to obtain an accurate NG stress factor (Ka). The results showed that the Img-Spec-PGE model with the NIR-RE-R spectral bands' combination had the highest LAI estimation accuracy (R-2 = 0.867, RMSE = 0.312) among the three designed DL models. The assimilated SAFY-NG model can accurately simulate the wheat LAI growth curve under NG leaks, and the calibration results of environmental parameters for NG leaks (leak start time and leak point depth) were consistent with the realistic experimental settings. Ka is sensitive to NG leaks in all experimental periods (p < 0.05). Enabling distinction between leak and non-leak scenarios as early as 1 day after NG leaks. 30 days after NG leaks, Ka can distinguish between 1 L min(-1) and 1.5 L min(-1) gas flow. The proposed approach provides a reference for detecting micro-leaks in NG transit and storage.
更多查看译文
关键词
Hyperspectral image,Natural gas leaks,Physical model,Deep learning model
AI 理解论文
溯源树
样例
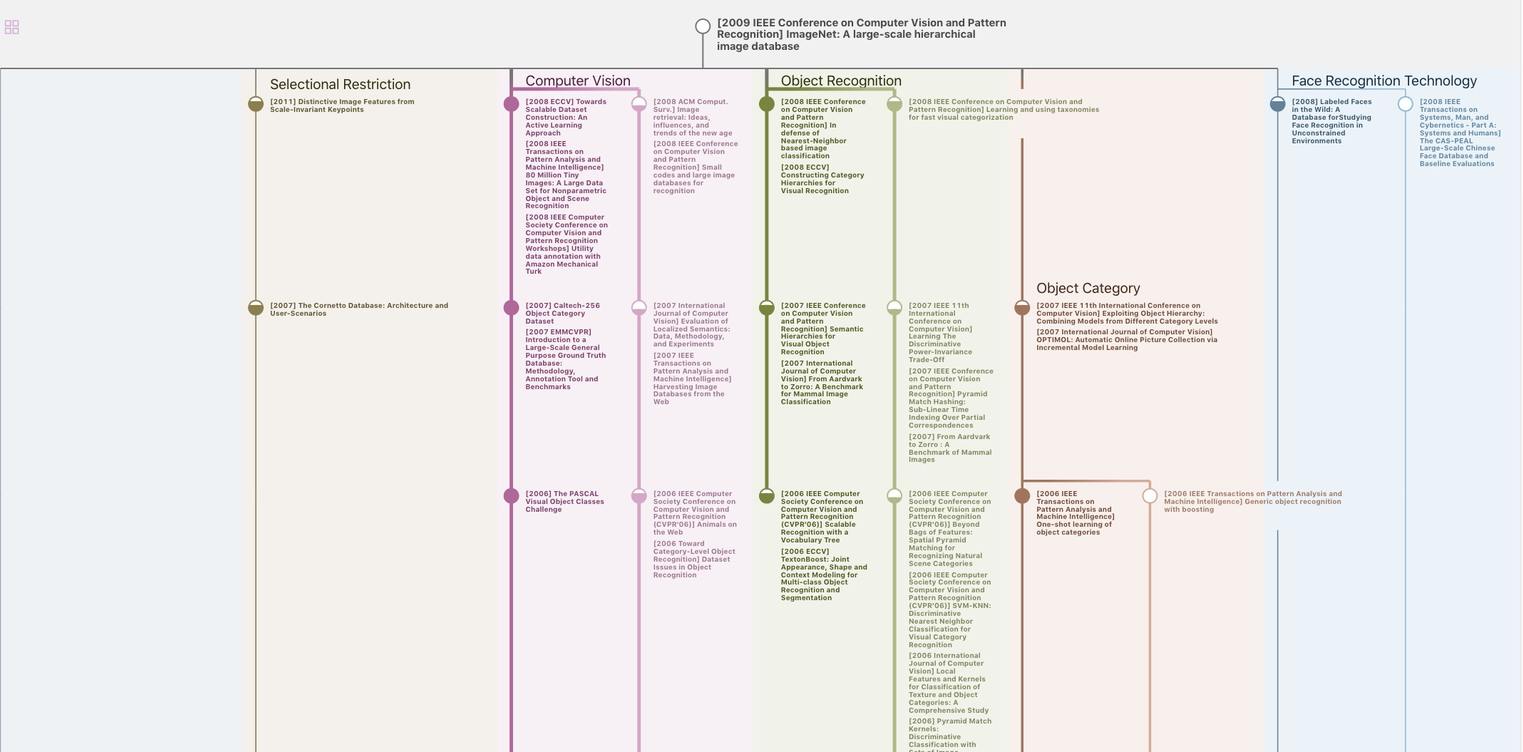
生成溯源树,研究论文发展脉络
Chat Paper
正在生成论文摘要