Battery calendar degradation trajectory prediction: Data-driven implementation and knowledge inspiration
Energy(2024)
摘要
—Batteries are acknowledged as an important carrier for next-generation energy and future electrification. Capacity degradation is an inevitable process that will eventually lead to a decline in battery performance. This paper presents a battery degradation trajectory prognostics approach under storage conditions based on the data-driven method with necessary knowledge inspiration. This data-driven approach is based on Support Vector Regression (SVR), incorporating mechanisms and empirical knowledge of battery calendar aging as the kernel. The developed data-driven approach is evaluated using two real but different capacity degradation datasets: Dataset A has two storage temperatures (25 °C and 45 °C) and storage SoCs (50% and 90%), while Dataset B has the same storage temperatures but four different storage SoCs (20%, 50%, 70% and 95%). The illustrative results show that the developed SVR-based data-driven approach with the battery knowledge-motivated kernel can make accurate future capacity prognostics under different storage conditions. Due to the superiority of its flexibility and knowledge-data-motivation, the developed approach could be extended and help engineers monitor or capture the future capacity degradation trajectory of other types of batteries when their relevant data become available, which in turn will benefit the life management of battery-based energy storage applications.
更多查看译文
关键词
Energy storage,Battery management,Health management,Data science
AI 理解论文
溯源树
样例
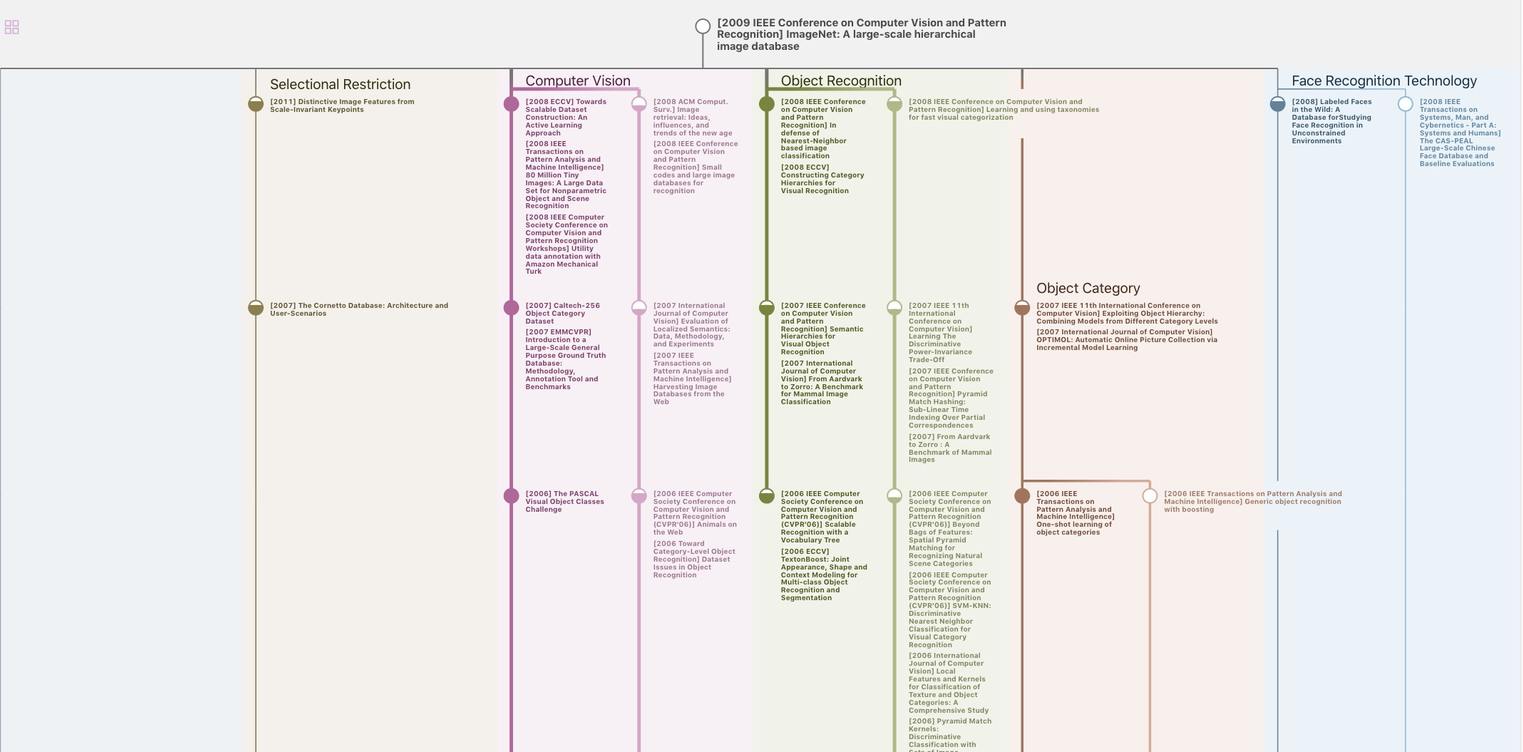
生成溯源树,研究论文发展脉络
Chat Paper
正在生成论文摘要