Detection of Cyber Attacks to Mitigate Their Impacts on the Manipulated EV Charging Prices
IEEE Transactions on Transportation Electrification(2024)
摘要
Electric vehicle (EV) ecosystem is a multi-actor cyberspace. Thus, interactions among the relevant actors should be considered to model the impacts of cyber-attacks on EV charging prices. In response, this paper copes with this issue through a novel two-step framework which is reinforced by employing a machine learning (ML)-based attack detector. In the first step, the interactions between the cyber attackers and EV management center (EVMC) are modeled as a bi-level optimization problem. In this modeling, the perspectives of both attackers and EVMC are considered. In addition, a new method using machine learning is developed to detect the cyber attacks aiming at manipulating EV charging prices. For this purpose, a self-generated dataset is created by modifying the EV charging price in the California state. This dataset is supplied into the developed ML algorithm, to analyze and detect any malicious data instantly. Support vector machine (SVM), Random Forest (RF), and multi-layer perceptron (MULP) algorithms are exploited in this work to improve the detection accuracy to 98%. In the second step, an optimization method is used to address the distribution system operator’s (DSO’s) concerns by power grid reconfiguration to minimize the grid’s power losses during the intervention. The IEEE-123 bus distribution system is employed to verify the performance of the proposed framework. The results have confirmed the effectiveness and the high performance of the proposed method. Application of this method resulted in considerable reduction in the financial losses of attacked EV charging stations.
更多查看译文
关键词
Cyber-attack,Electric vehicles (EVs),extreme fast chargers (XFCs),FDIA,ML algorithm
AI 理解论文
溯源树
样例
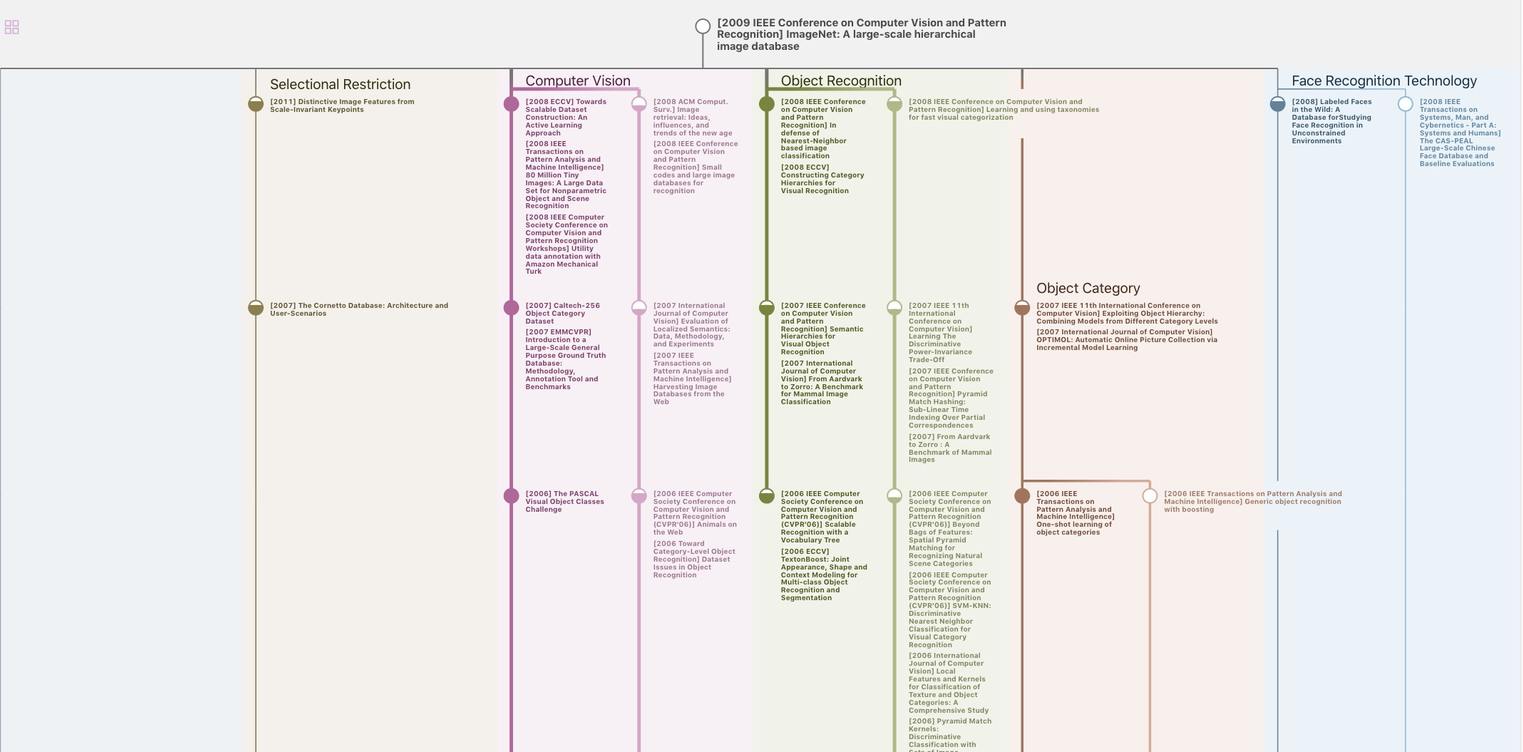
生成溯源树,研究论文发展脉络
Chat Paper
正在生成论文摘要