FHVAC: Feature-Level Hybrid Video Adaptive Configuration for Machine-Centric Live Streaming.
IEEE Trans. Parallel Distributed Syst.(2024)
摘要
With the widespread deployment of edge computing, the focus has shifted to machine-centric live video streaming, where endpoint-collected videos are transmitted over networks to edge servers for analysis. Unlike maximizing user's Quality of Experience (QoE), machine-centric video streaming optimizes the machine's Quality of Inference (QoI) by balancing the inference accuracy, inference delay, and transmission latency with video adaptive configuration. Traditional heuristic configuration adaption methods are reliable but unable to respond to erratic network fluctuations. Reinforcement learning (RL) based algorithms exhibit superior flexibility but suffer from exploration mechanisms, resulting in long-tail effects on upload latency. In this paper, we propose FHVAC, which dynamically selects video encoding parameters for live streaming by coherently fusing rule-based and RL-based agent at the feature level. We initially develop a robust rule-based approach for ensuring the low latency in transmission, and employ imitation learning to convert it into a neural network equivalently. Subsequently, we design a novel module to combine the two approaches and assess various fusion mechanisms. Our evaluation of FHVAC across two vision tasks (pose estimation and semantic segmentation) in two scenarios (trace-driven simulation and testbed-based experiment) shows that FHVAC enhances the average QoI, and reduces 10.61%-65.27% latency tail performance compared to prior work.
更多查看译文
关键词
Video Configuration Adaptation,Machine-centric,Live Video Streaming,Reinforcement Learnin
AI 理解论文
溯源树
样例
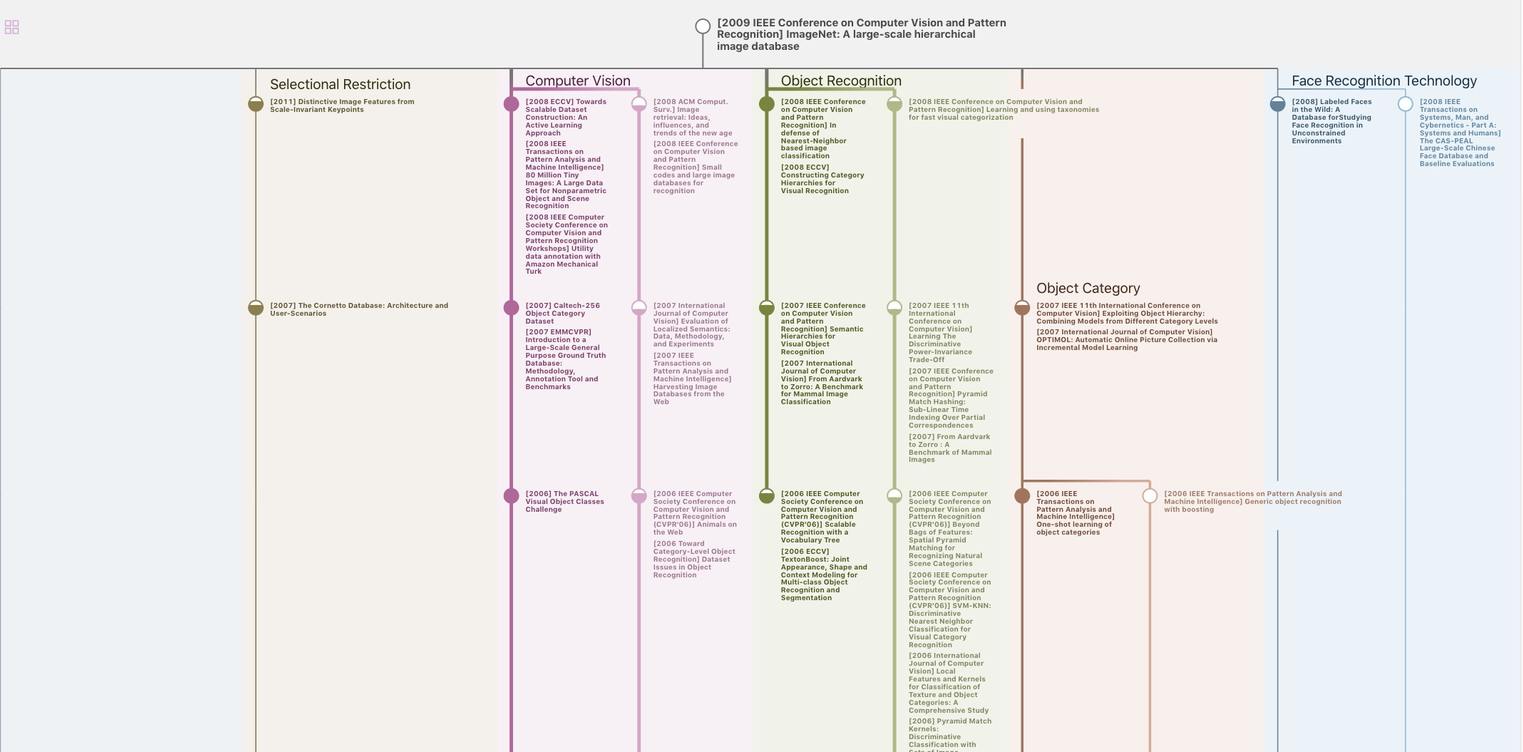
生成溯源树,研究论文发展脉络
Chat Paper
正在生成论文摘要