Unsupervised Transfer Aided Lifelong Regression For Learning New Tasks without Target Output
IEEE Transactions on Knowledge and Data Engineering(2024)
摘要
As an emerging learning paradigm, lifelong learning solves multiple consecutive tasks based upon previously accumulated knowledge. When facing with a new task, existing lifelong learning approaches need both input and desired output data to construct task models before knowledge transfer can succeed. However, labeling each task requires extensive labors and time, which can be prohibitive for real-world lifelong regression problems. To reduce this burden, we propose to incorporate unsupervised feature into lifelong regression via coupled dictionary learning, enabling to learn new tasks without target output data. Specifically, the input data for each task is encoded as unsupervised feature while both input and output data are used to construct task predictor. The unsupervised feature is linked with task predictor through two dictionaries that are coupled by a joint sparse representation. Because of the learned coupling between the two spaces, the task predictor for the new coming task can be recovered given only the input data. We further incorporate active task selection into this framework, enabling actively choosing tasks to learn in a task-efficient manner. Three case studies are used to evaluate the effectiveness of our method, in comparison with existing lifelong learning approaches. Results show that our method is able to accurately predict new tasks through unsupervised transfer, eliminating the need to label tasks before constructing the predictor.
更多查看译文
关键词
Lifelong regression,unsupervised feature,coupled dictionary learning,knowledge transfer,active task selection
AI 理解论文
溯源树
样例
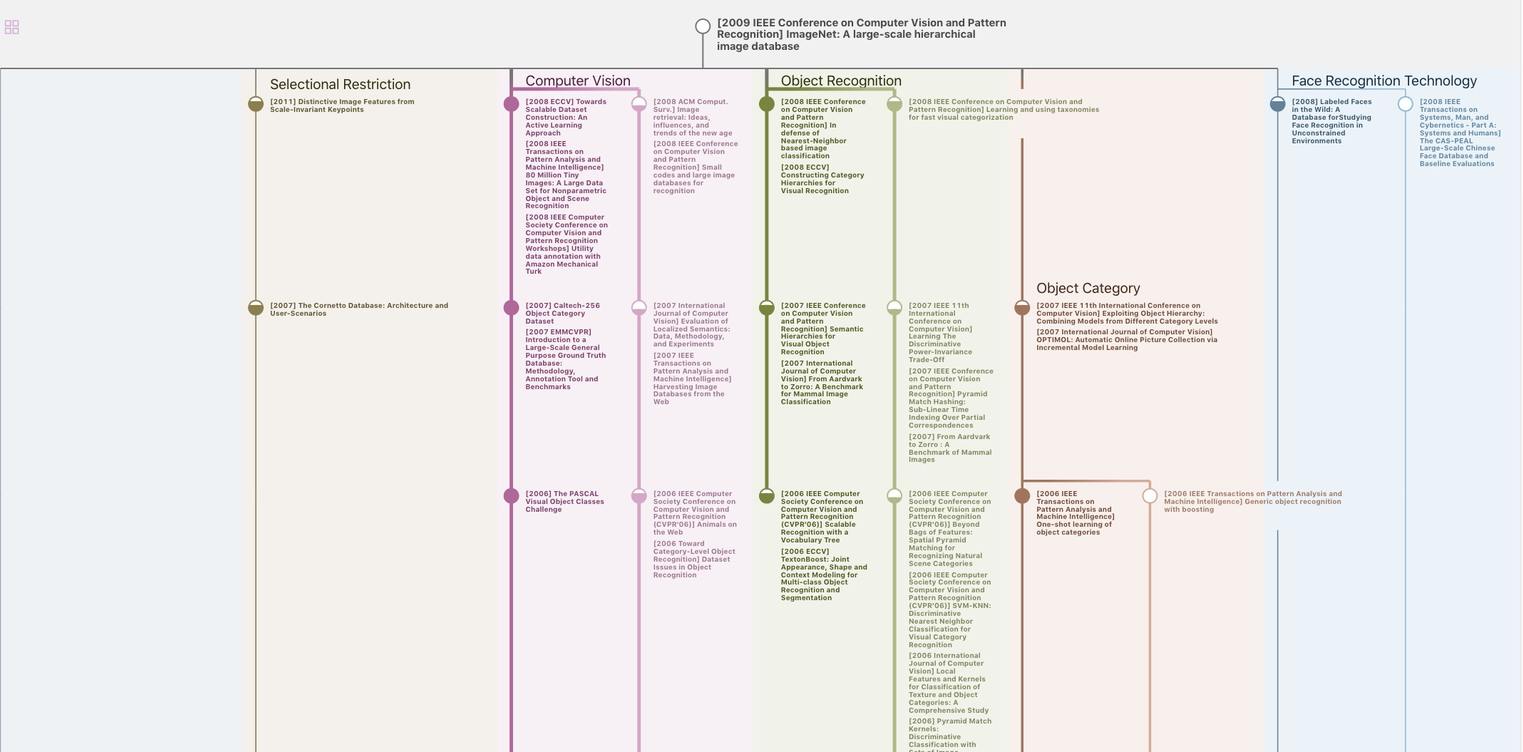
生成溯源树,研究论文发展脉络
Chat Paper
正在生成论文摘要