Aware Distribute and Sparse Network for Infrared Small Target Detection
IEEE ACCESS(2024)
摘要
Deep learning has achieved tremendous success in the field of object detection. The efficient detection of infrared small targets using deep learning methods remains a challenging task. Infrared small targets are often detected in high-resolution features. Extracting high-level semantic features layer by layer in the network may lead to the loss of deep-layer targets. However, performing global detection on high-resolution feature maps results in high computational costs. To address this issue, we propose the aware distribute and sparse network (ADSNet) to preserve deep-layer small target features while accelerating inference speed. Specifically, we design the aware fusion distribute module (AFD) to aggregate global features and enhance the representation capability of deep-layer features. Subsequently, the aware cascaded sparse module (ACS) is utilized to guide step-by-step high-resolution feature sparsification. Experimental results demonstrate that the proposed method achieves accurate segmentation in various detection scenarios and for diverse target morphologies, effectively suppressing false alarms while controlling computational expenses. Ablation experiments further validate the effectiveness of each component.
更多查看译文
关键词
Object detection,infrared imaging,infrared small target detection,feature fusion
AI 理解论文
溯源树
样例
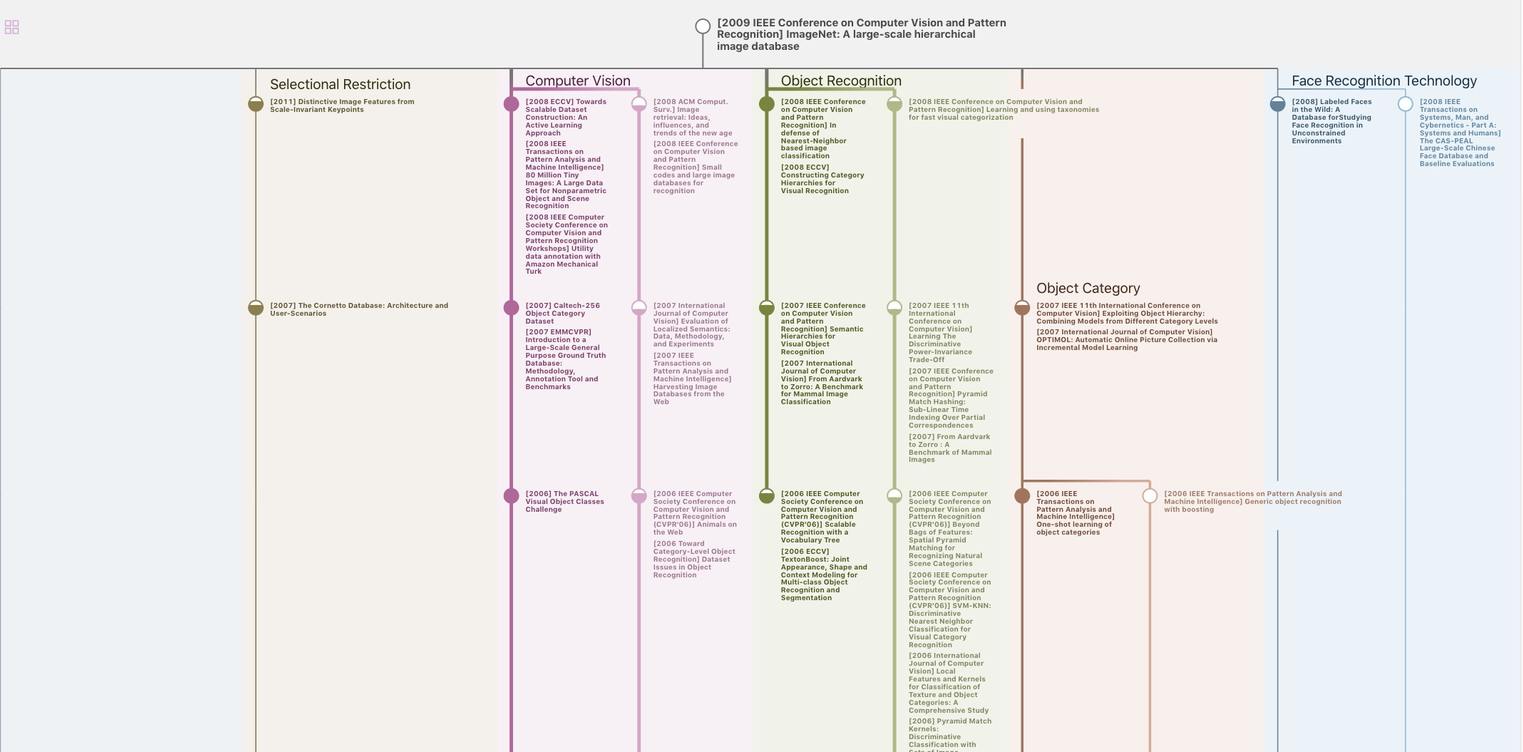
生成溯源树,研究论文发展脉络
Chat Paper
正在生成论文摘要