A Q-learning memetic algorithm for energy-efficient heterogeneous distributed assembly permutation flowshop scheduling considering priorities
SWARM AND EVOLUTIONARY COMPUTATION(2024)
摘要
Most studies on distributed assembly permutation flowshop scheduling do not consider product priorities and factory heterogeneity. This causes delays in critical products and cannot reflect the real -world production situation. This paper focuses on the energy -efficient heterogeneous distributed assembly permutation flowshop scheduling considering priorities (EHDAPFS-P) to minimize total tardiness and total energy consumption simultaneously. Unlike traditional models, factory heterogeneity and product priorities are considered to better reflect the production environment and customer satisfaction in real -world situations. Then, a Qlearning memetic algorithm (QLMA) is proposed to solve this problem: (i) a high -quality initial population is obtained using a hybrid initialization strategy that combines four problem -specific heuristics; (ii) six efficient neighborhood structures are tailored to guide the population to converge to the promising areas; (iii) the most useful neighborhood structure is selected among the six structures using the Q -learning algorithm to accelerate the convergence, thus maximizing the cumulative and future improvements according to the population state; and (iv) an energy -saving strategy is developed to optimize the total energy consumption without deteriorating the total tardiness. The proposed QLMA is compared with seven state-of-the-art algorithms on 261 benchmark instances to demonstrate its superiority or at least competitiveness.
更多查看译文
关键词
Assembly permutation flowshop scheduling,Distributed scheduling,Heterogeneous factories,Product priorities,Q-learning,Energy-saving
AI 理解论文
溯源树
样例
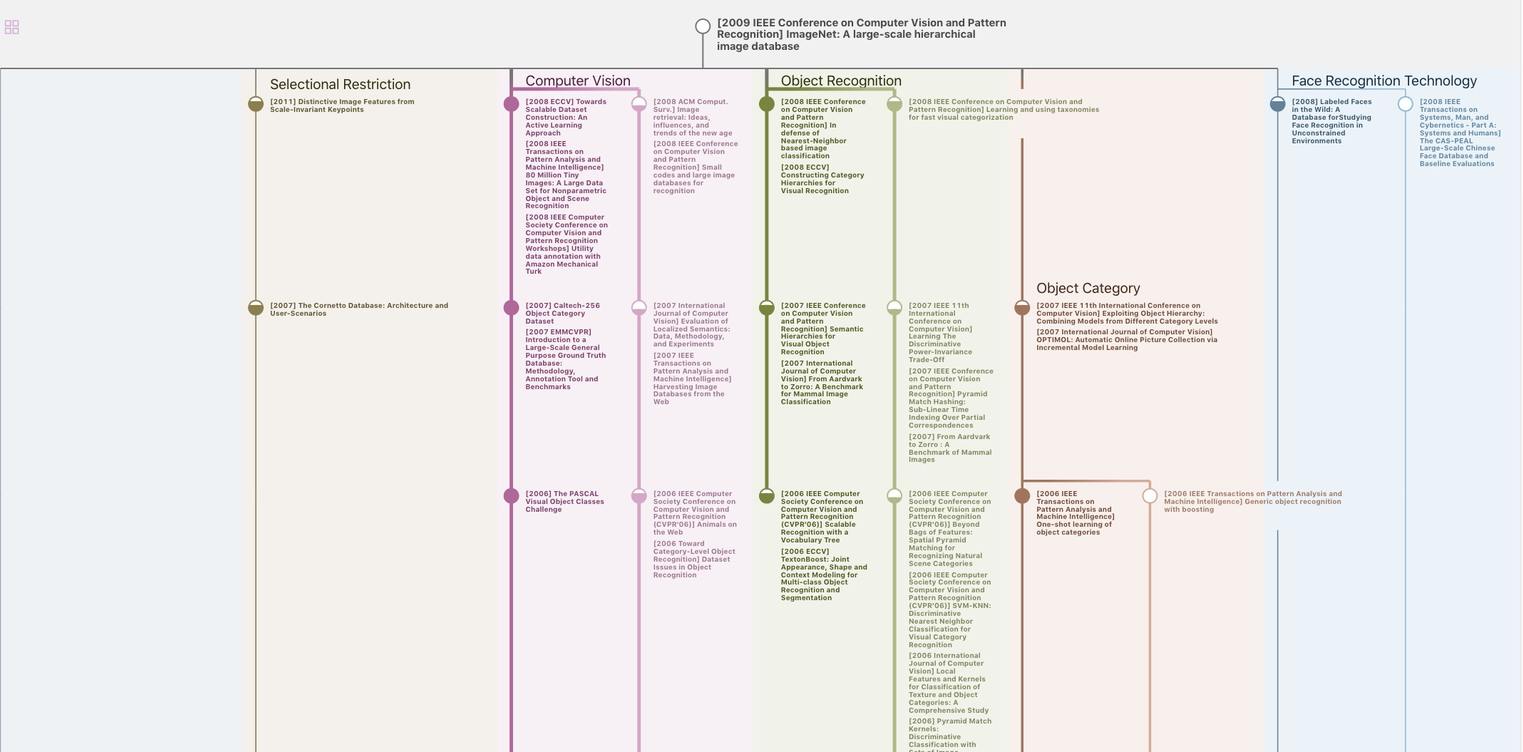
生成溯源树,研究论文发展脉络
Chat Paper
正在生成论文摘要