ForTune: Running Offline Scenarios to Estimate Impact on Business Metrics
arxiv(2024)
摘要
Making ideal decisions as a product leader in a web-facing company is
extremely difficult. In addition to navigating the ambiguity of customer
satisfaction and achieving business goals, one must also pave a path forward
for ones' products and services to remain relevant, desirable, and profitable.
Data and experimentation to test product hypotheses are key to informing
product decisions. Online controlled experiments by A/B testing may provide the
best data to support such decisions with high confidence, but can be
time-consuming and expensive, especially when one wants to understand impact to
key business metrics such as retention or long-term value. Offline
experimentation allows one to rapidly iterate and test, but often cannot
provide the same level of confidence, and cannot easily shine a light on impact
on business metrics. We introduce a novel, lightweight, and flexible approach
to investigating hypotheses, called scenario analysis, that aims to support
product leaders' decisions using data about users and estimates of business
metrics. Its strengths are that it can provide guidance on trade-offs that are
incurred by growing or shifting consumption, estimate trends in long-term
outcomes like retention and other important business metrics, and can generate
hypotheses about relationships between metrics at scale.
更多查看译文
AI 理解论文
溯源树
样例
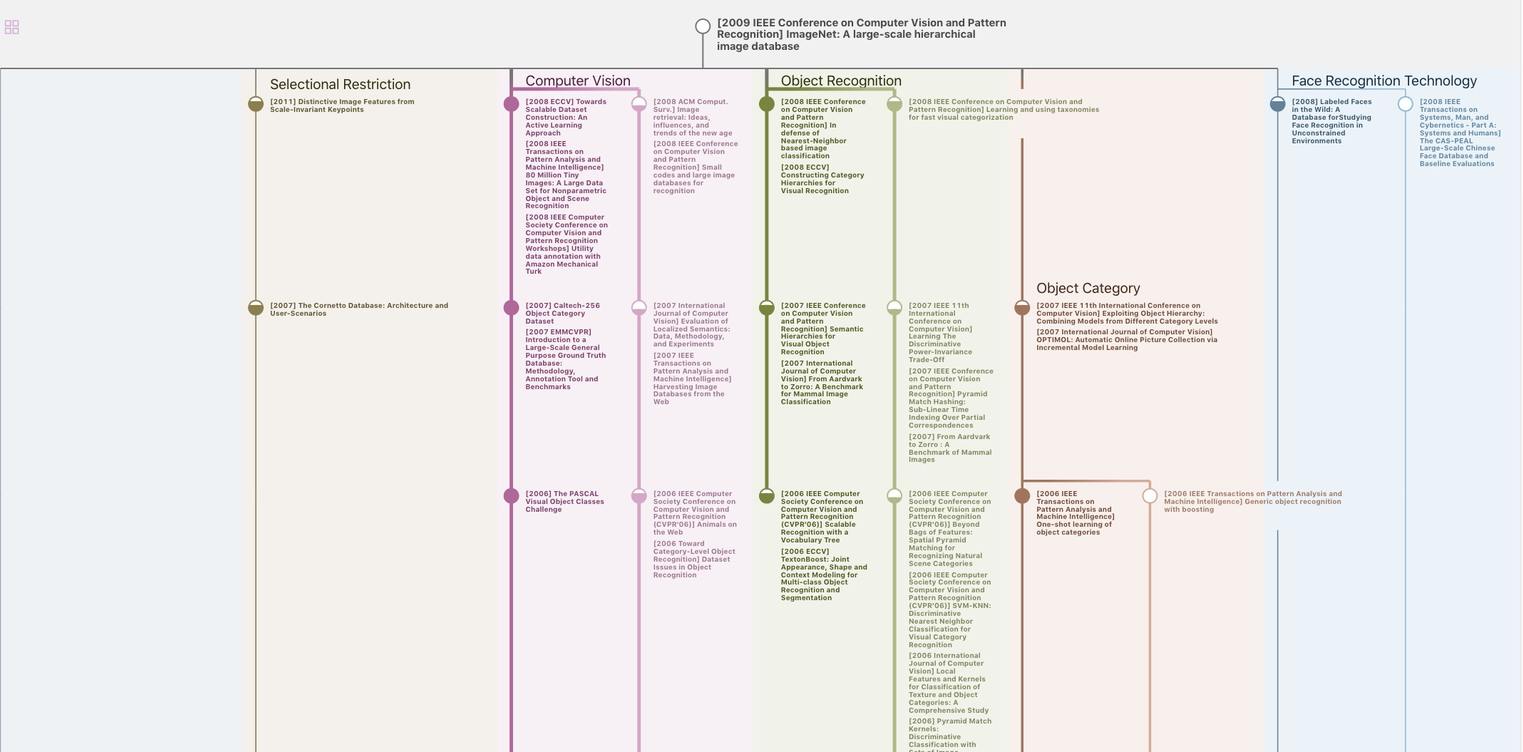
生成溯源树,研究论文发展脉络
Chat Paper
正在生成论文摘要