Synthesizing study-specific controls using generative models on open access datasets for harmonized multi-study analyses
arxiv(2024)
摘要
Neuroimaging consortia can enhance reliability and generalizability of
findings by pooling data across studies to achieve larger sample sizes. To
adjust for site and MRI protocol effects, imaging datasets are often harmonized
based on healthy controls. When data from a control group were not collected,
statistical harmonization options are limited as patient characteristics and
acquisition-related variables may be confounded. Here, in a multi-study
neuroimaging analysis of Alzheimer's patients and controls, we tested whether
it is possible to generate synthetic control MRIs. For one case-control study,
we used a generative adversarial model for style-based harmonization to
generate site-specific controls. Downstream feature extraction, statistical
harmonization and group-level multi-study case-control and case-only analyses
were performed twice, using either true or synthetic controls. All effect sizes
using synthetic controls overlapped with those based on true study controls.
This line of work may facilitate wider inclusion of case-only studies in
multi-study consortia.
更多查看译文
AI 理解论文
溯源树
样例
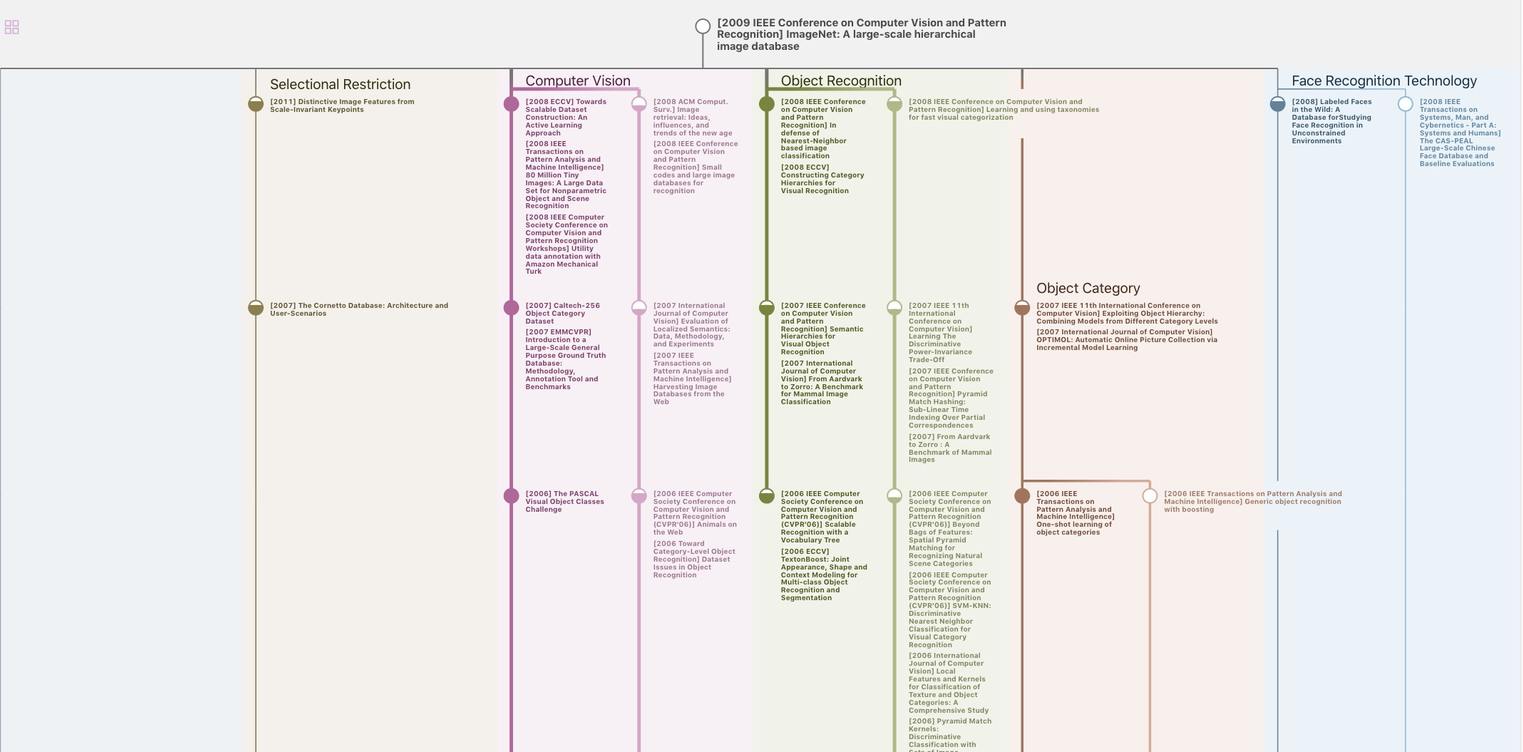
生成溯源树,研究论文发展脉络
Chat Paper
正在生成论文摘要