High-frequency channel attention and contrastive learning for image super-resolution
The Visual Computer(2024)
摘要
Over the last decade, convolutional neural networks (CNNs) have allowed remarkable advances in single image super-resolution (SISR). In general, recovering high-frequency features is crucial for high-performance models. High-frequency features suffer more serious damages than low-frequency features during downscaling, making it hard to recover edges and textures. In this paper, we attempt to guide the network to focus more on high-frequency features in restoration from both channel and spatial perspectives. Specifically, we propose a high-frequency channel attention (HFCA) module and a frequency contrastive learning (FCL) loss to aid the process. For the channel-wise perspective, the HFCA module rescales channels by predicting statistical similarity metrics of the feature maps and their high-frequency components. For the spatial perspective, the FCL loss introduces contrastive learning to train a spatial mask that adaptively assigns high-frequency areas with large scaling factors. We incorporate the proposed HFCA module and FCL loss into an EDSR baseline model to construct the proposed lightweight high-frequency channel contrastive network (HFCCN). Extensive experimental results show that it can yield markedly improved or competitive performances compared to the state-of-the-art networks of similar model parameters.
更多查看译文
关键词
Image super-resolution,Attention mechanism,Contrastive learning,Deep learning
AI 理解论文
溯源树
样例
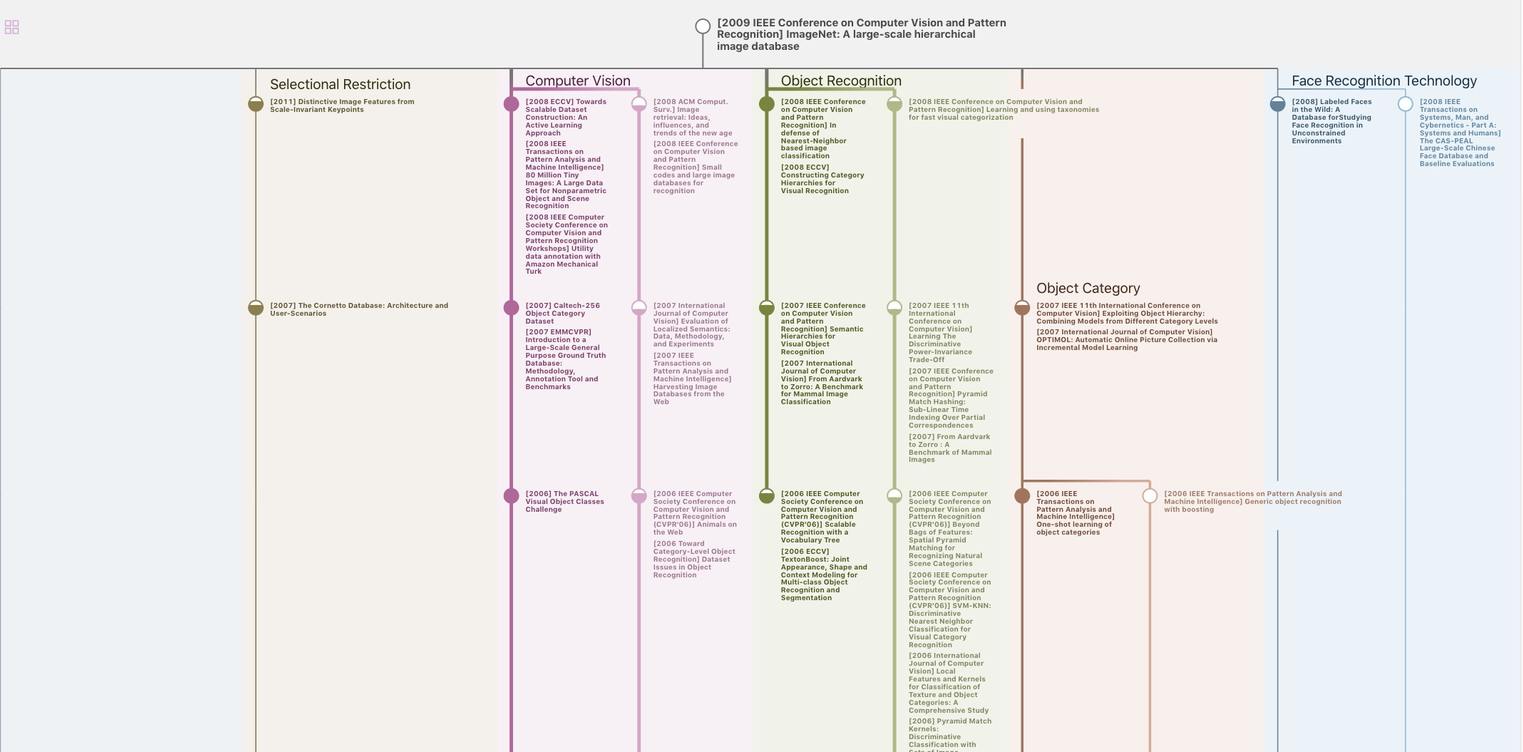
生成溯源树,研究论文发展脉络
Chat Paper
正在生成论文摘要