Privacy-Preserving Deep Reinforcement Learning Based On Adaptive Noise For Sepsis Treatment
2023 IEEE Smart World Congress (SWC)(2023)
摘要
In recent years, deep reinforcement learning has made amazing achievements in the field of personalized treatment plan recommendation, especially in sepsis treatment. But deep reinforcement learning has privacy leakage problem. Differential privacy is a reliable privacy-preserving technique that has been proven to be effective in protecting the privacy of machine learning from adversary attacks on sensitive data. However, existing methods add the same amount of noise in the model gradient, which dramatically reduces the usability of the model. In this paper, we propose a privacy-preserving deep reinforcement learning based on relevance analysis, that is used add noise adaptively. This approach aims to better balance the usability and privacy preservation of reinforcement learning models for sepsis treatment. Our experimental results shows that this mechanism can achieve higher model usability with the same privacy-preserving strength.
更多查看译文
关键词
deep reinforcement learning,sepsis,adaptive noise,differential privacy
AI 理解论文
溯源树
样例
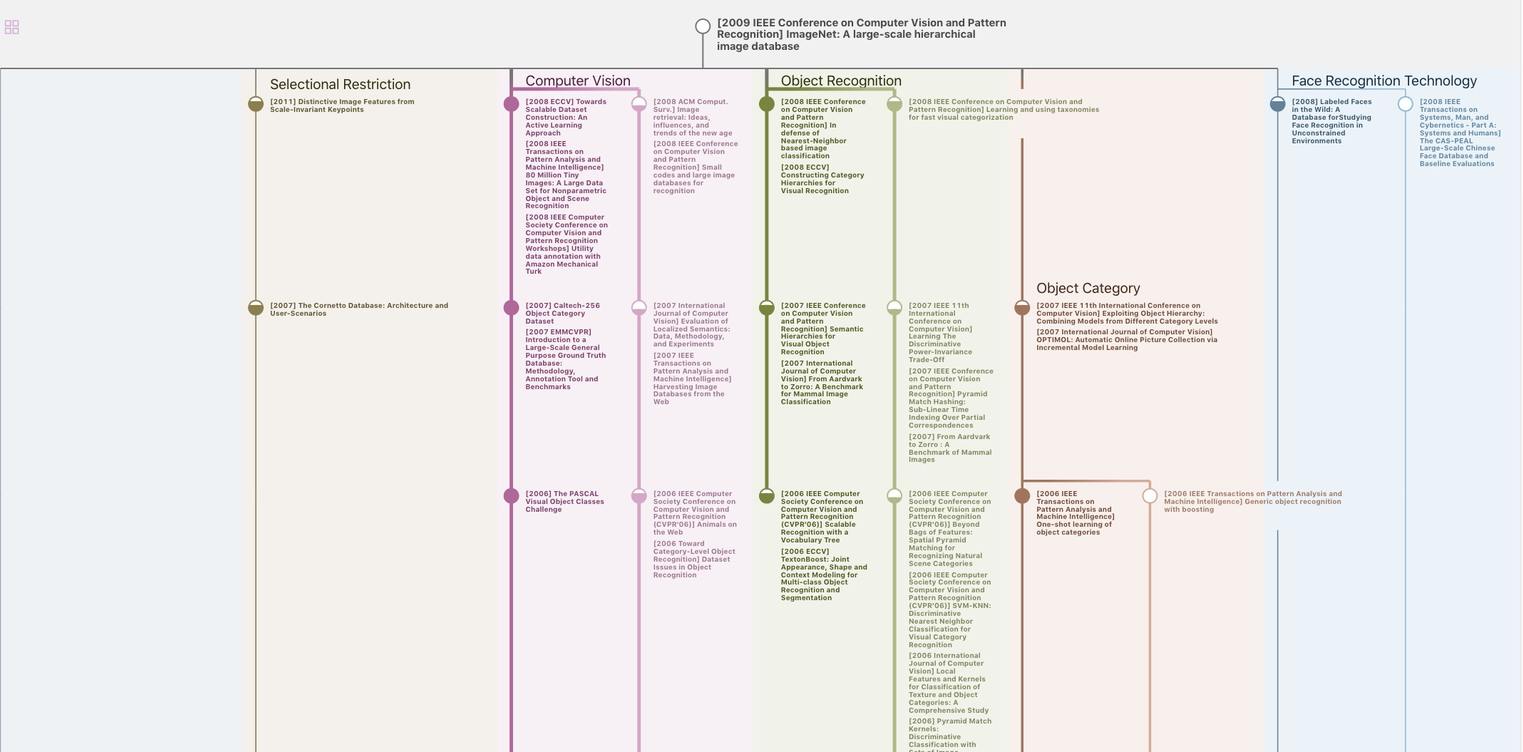
生成溯源树,研究论文发展脉络
Chat Paper
正在生成论文摘要