Fast-DAVAD : Domain Adaptation for Fast Video Anomaly Detection on Resource-Constrained Edge Devices
2023 IEEE Smart World Congress (SWC)(2023)
摘要
The development of artificial intelligence of things (AIoT) makes it possible to detect video anomalies on intelligent edge devices with low transmission delay and data privacy. However, the performance of these applications is constrained by the domain shift between the diverse real-world environment and the training data. Despite the existence of many advanced domain adaptation methods, it is difficult to deploy these methods on resource-constrained intelligent edge devices due to their model complexity. In addition, there is a lack of large amounts of labeled real-scenario data to complete model training. In this paper, we propose the Fast-DAVAD, an unsupervised end-to-end lightweight adaptive video anomaly detection framework. It solves the domain shift problem through multi-level adversarial domain adaptation. Furthermore, it achieves short training time and inference latency on resource-constrained edge devices with Residual Unet, and applies a memory module to guarantee detection accuracy. Extensive experiments on public datasets and multiple platforms, such as PC and NVIDIA Jetson, demonstrate that the effectiveness and superiority of Fast-DAVAD compared with state-of-the-art domain adaptation-based video anomaly detection solutions.
更多查看译文
关键词
domain adaptation,video anomaly detection,edge computing
AI 理解论文
溯源树
样例
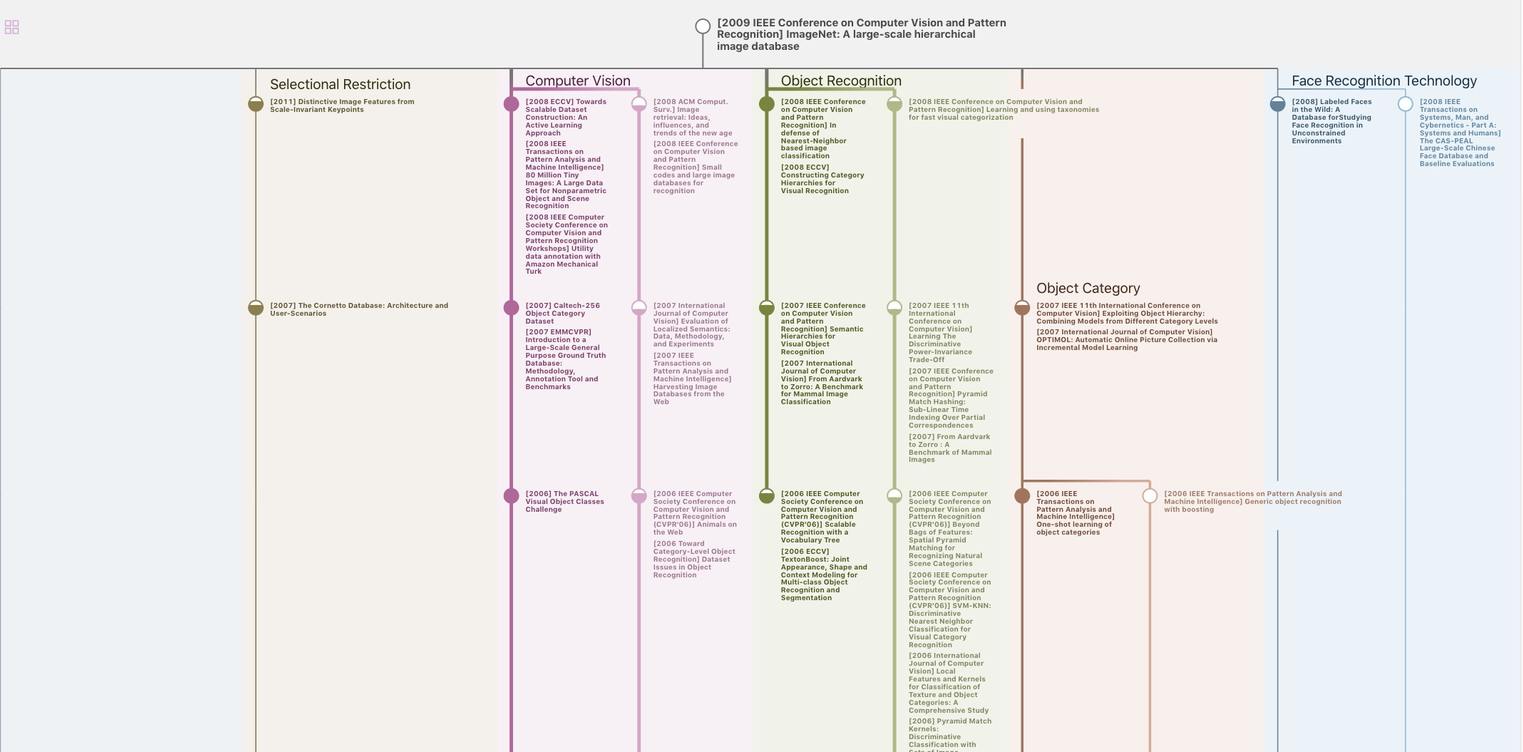
生成溯源树,研究论文发展脉络
Chat Paper
正在生成论文摘要