An engagement-aware predictive model to evaluate problem-solving performance from the study of adult skills' (PIAAC 2012) process data
Large-scale Assessments in Education(2024)
摘要
The benefits of incorporating process information in a large-scale assessment with the complex micro-level evidence from the examinees (i.e., process log data) are well documented in the research across large-scale assessments and learning analytics. This study introduces a deep-learning-based approach to predictive modeling of the examinee’s performance in sequential, interactive problem-solving tasks from a large-scale assessment of adults' educational competencies. The current methods disambiguate problem-solving behaviors using network analysis to inform the examinee's performance in a series of problem-solving tasks. The unique contribution of this framework lies in the introduction of an “effort-aware” system. The system considers the information regarding the examinee’s task-engagement level to accurately predict their task performance. The study demonstrates the potential to introduce a high-performing deep learning model to learning analytics and examinee performance modeling in a large-scale problem-solving task environment collected from the OECD Programme for the International Assessment of Adult Competencies (PIAAC 2012) test in multiple countries, including the United States, South Korea, and the United Kingdom. Our findings indicated a close relationship between the examinee's engagement level and their problem-solving skills as well as the importance of modeling them together to have a better measure of students’ problem-solving performance.
更多查看译文
关键词
PIAAC,PS-TRE,Long-short-term-memory (LSTM),Attention,Engagement,Problem-solving performance,Log information
AI 理解论文
溯源树
样例
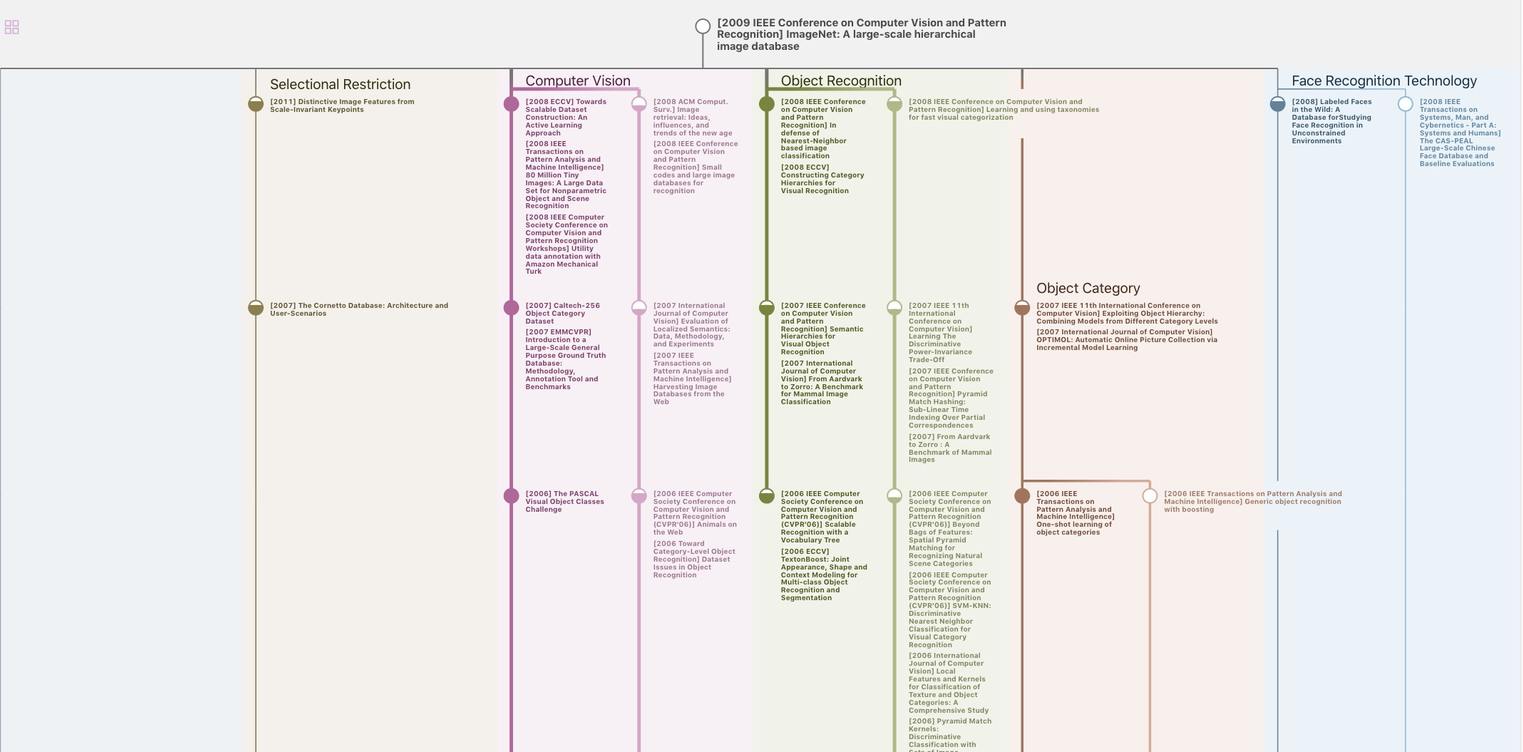
生成溯源树,研究论文发展脉络
Chat Paper
正在生成论文摘要