Unsupervised Pansharpening Based on Double-Cycle Consistency.
IEEE Trans. Geosci. Remote. Sens.(2024)
摘要
Multispectral (MS) pansharpening can improve the spatial resolution of MS images by fusing panchromatic (PAN) images, which have important applications in the fields of smart agriculture and environmental monitoring. However, existing supervised algorithms treat the original MS images as ground truth and generate training data under Wald’s protocol, resulting in a gap between the learned degradation process of the model and reality. This leads to the model having poor generalization and impractical. Unsupervised pansharpening methods often struggle to fully explore the rich information contained in images, leading to suboptimal pansharpening outcomes. In this work, we propose an unsupervised pansharpening algorithm based on double-cycle consistency that can learn directly from the original MS images without relying on artificially simulated degradation processes. Specifically, the network with cross-domain correlation information interaction is developed to achieve a deep fusion of spatial and spectral features. To address the inaccurate degradation mechanism representation of MS images, a spatial information extraction module based on scale invariance is developed to achieve an accurate representation. Meanwhile, double-cycle consistency loss is proposed to reduce the information loss caused by simulated degradation during the cycle process. Experimental results show that this method outperforms existing unsupervised pansharpening methods in both quantitative and qualitative evaluation of full-resolution images.
更多查看译文
关键词
Multispectral pansharpening,unsupervised learning,generative adversarial network (GAN),cycle consistency
AI 理解论文
溯源树
样例
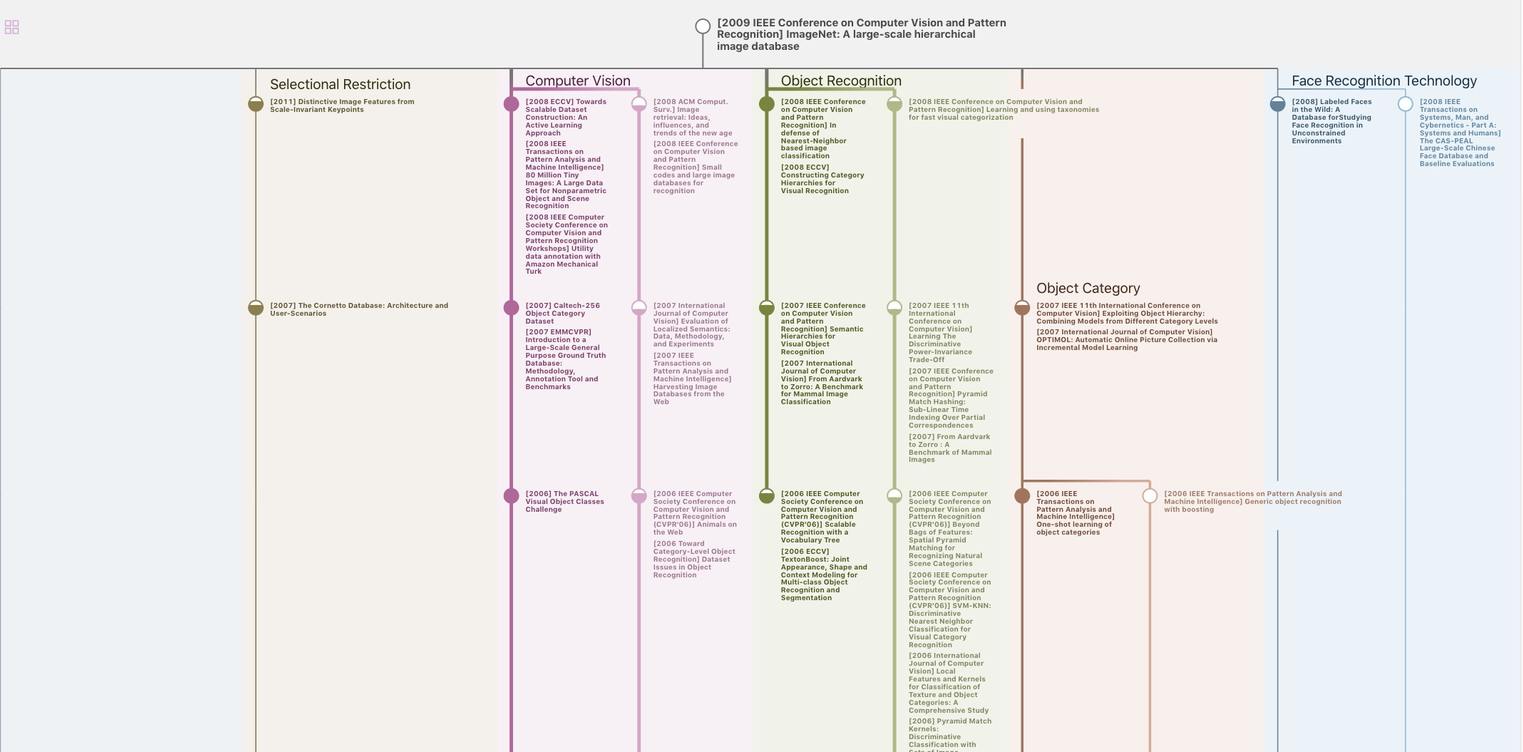
生成溯源树,研究论文发展脉络
Chat Paper
正在生成论文摘要