Distributed State Estimation for Sparse Stochastic Systems Based On Compressed Sensing
IEEE Transactions on Circuits and Systems II: Express Briefs(2024)
摘要
This brief proposes a compressed distributed Kalman filter to cooperatively estimate the sparse state vector of a dynamic system with general stochastic coefficients. Based on the compressed sensing theory and the diffusion strategy, each sensor first compresses the original high-dimensional and sparse coefficient matrices via the sensing matrix. Then, each sensor diffuses the local innovation pairs with neighbors to obtain a distributed Kalman estimate in the compressed low-dimensional space. Subsequently, the original high-dimensional sparse state vector can be well recovered by the reconstruction technique. Under the compressed collective stochastic observability condition, the upper bound for the estimation error is established. Note that our theoretical results are established without such stringent conditions as independence or stationarity of the coefficient matrices and are thus applicable to feedback systems. Finally, a simulation example is given to illustrate our theoretical results.
更多查看译文
关键词
Sparse state estimation,distributed Kalman filter,compressed sensing,stochastic dynamic system
AI 理解论文
溯源树
样例
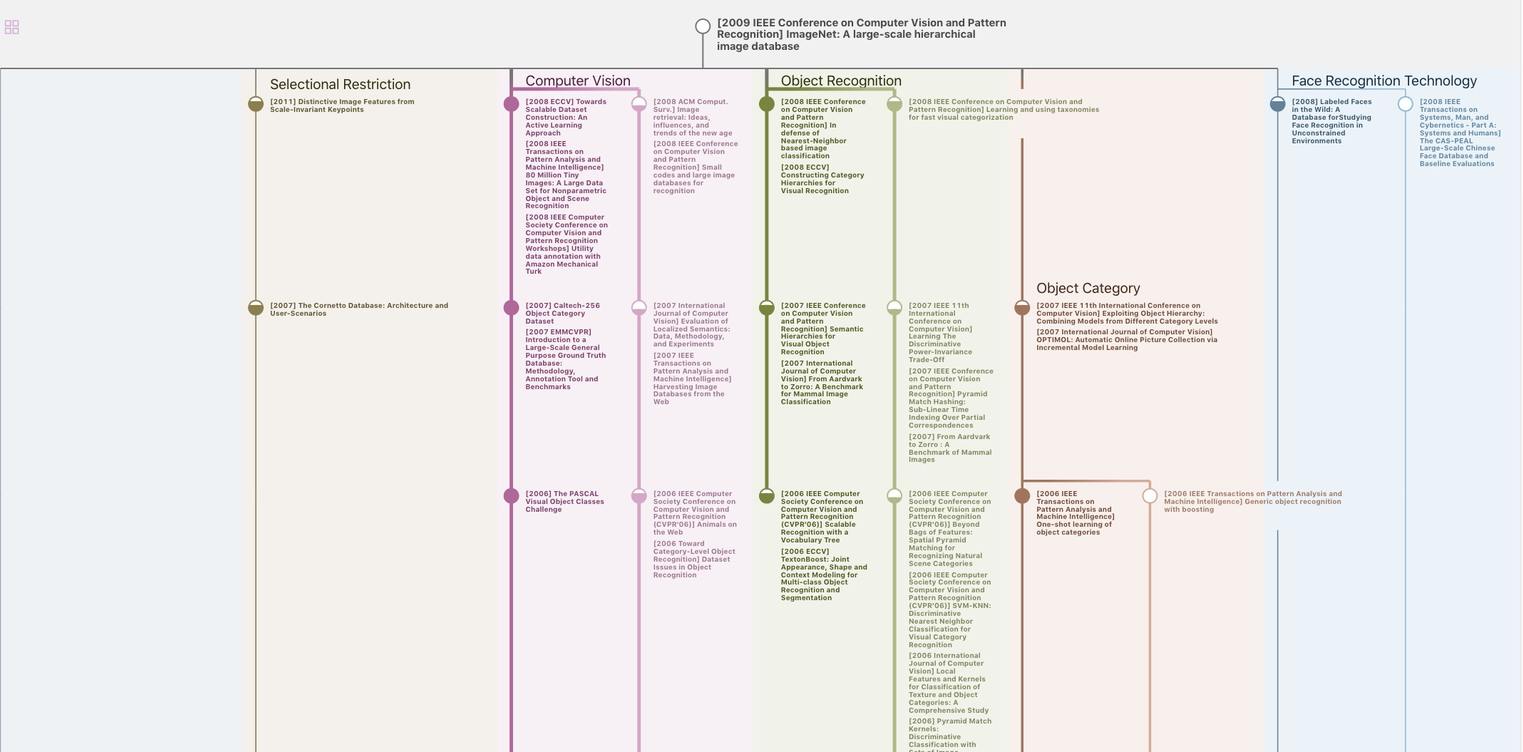
生成溯源树,研究论文发展脉络
Chat Paper
正在生成论文摘要