One model to use them all: training a segmentation model with complementary datasets
International Journal of Computer Assisted Radiology and Surgery(2024)
摘要
Understanding surgical scenes is crucial for computer-assisted surgery systems to provide intelligent assistance functionality. One way of achieving this is via scene segmentation using machine learning (ML). However, such ML models require large amounts of annotated training data, containing examples of all relevant object classes, which are rarely available. In this work, we propose a method to combine multiple partially annotated datasets, providing complementary annotations, into one model, enabling better scene segmentation and the use of multiple readily available datasets. Our method aims to combine available data with complementary labels by leveraging mutual exclusive properties to maximize information. Specifically, we propose to use positive annotations of other classes as negative samples and to exclude background pixels of these binary annotations, as we cannot tell if a positive prediction by the model is correct. We evaluate our method by training a DeepLabV3 model on the publicly available Dresden Surgical Anatomy Dataset, which provides multiple subsets of binary segmented anatomical structures. Our approach successfully combines 6 classes into one model, significantly increasing the overall Dice Score by 4.4
更多查看译文
关键词
Full scene segmentation,Multi-class segmentation,Surgical scene understanding,Dataset availability,Surgical data science,Computer-assisted surgery
AI 理解论文
溯源树
样例
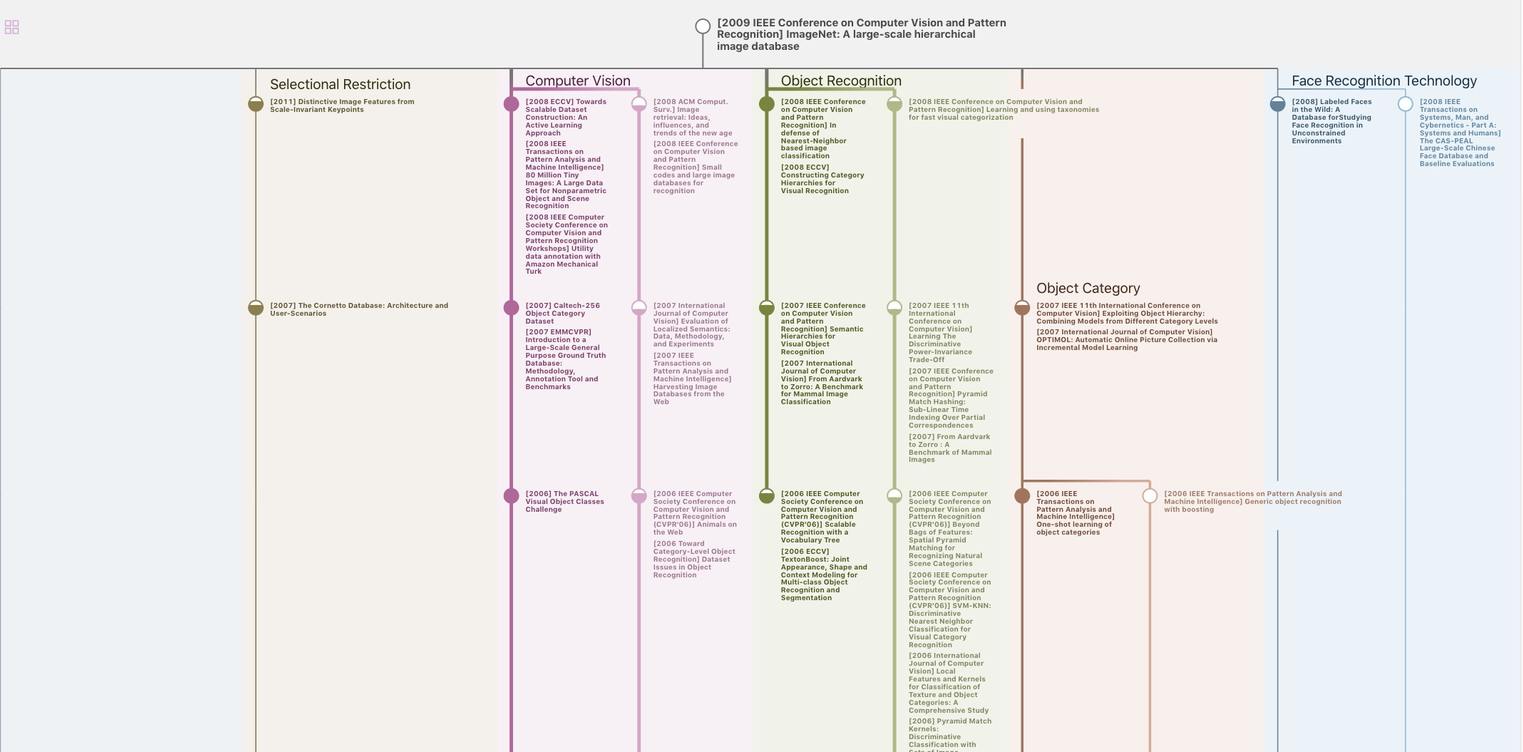
生成溯源树,研究论文发展脉络
Chat Paper
正在生成论文摘要