Boosting Adversarial Training with Hardness-Guided Attack Strategy
IEEE Transactions on Multimedia(2024)
摘要
The susceptibility of deep neural networks (DNNs) to adversarial examples has raised significant concerns regarding the security and reliability of artificial intelligence systems. These examples contain maliciously crafted perturbations not perceptible to the human eye but can cause the model to make wrong predictions. Adversarial training (AT) is the de facto standard method for enhancing adversarial robustness. However, the improved robustness is often at the cost of a significant drop in standard accuracy for clean samples. Numerous works have attempted to alleviate this trade-off by identifying its causes. A key factor lies in the variability of clean samples, which leads to different adversarial examples being generated using the same attack strategy. The other factor is the disruption of the underlying data structure caused by adversarial perturbations. To overcome these challenges, we propose a novel adversarial training framework named Hardness-Guided Sample-Dependent Adversarial Training (HGSD-AT), which dynamically adjusts the attack strategy based on the hardness of the current adversarial sample to further improve the robustness of the model. By utilizing the two types of constraints which construct from a temporal perspective and spatial distribution perspective, our method directly learns the impact of attack methods on the model, rather than the indirect effects associated with sample distribution. This approach aims to improve the generation of adversarial examples while simultaneously enhancing the robustness and accuracy of DNNs. Our approach exhibits superior performance in terms of both robustness and natural accuracy compared to state-of-the-art defense methods, as validated through comprehensive experiments conducted on three benchmark datasets.
更多查看译文
关键词
Model Robustness,Adversarial Defense,Adversarial Training
AI 理解论文
溯源树
样例
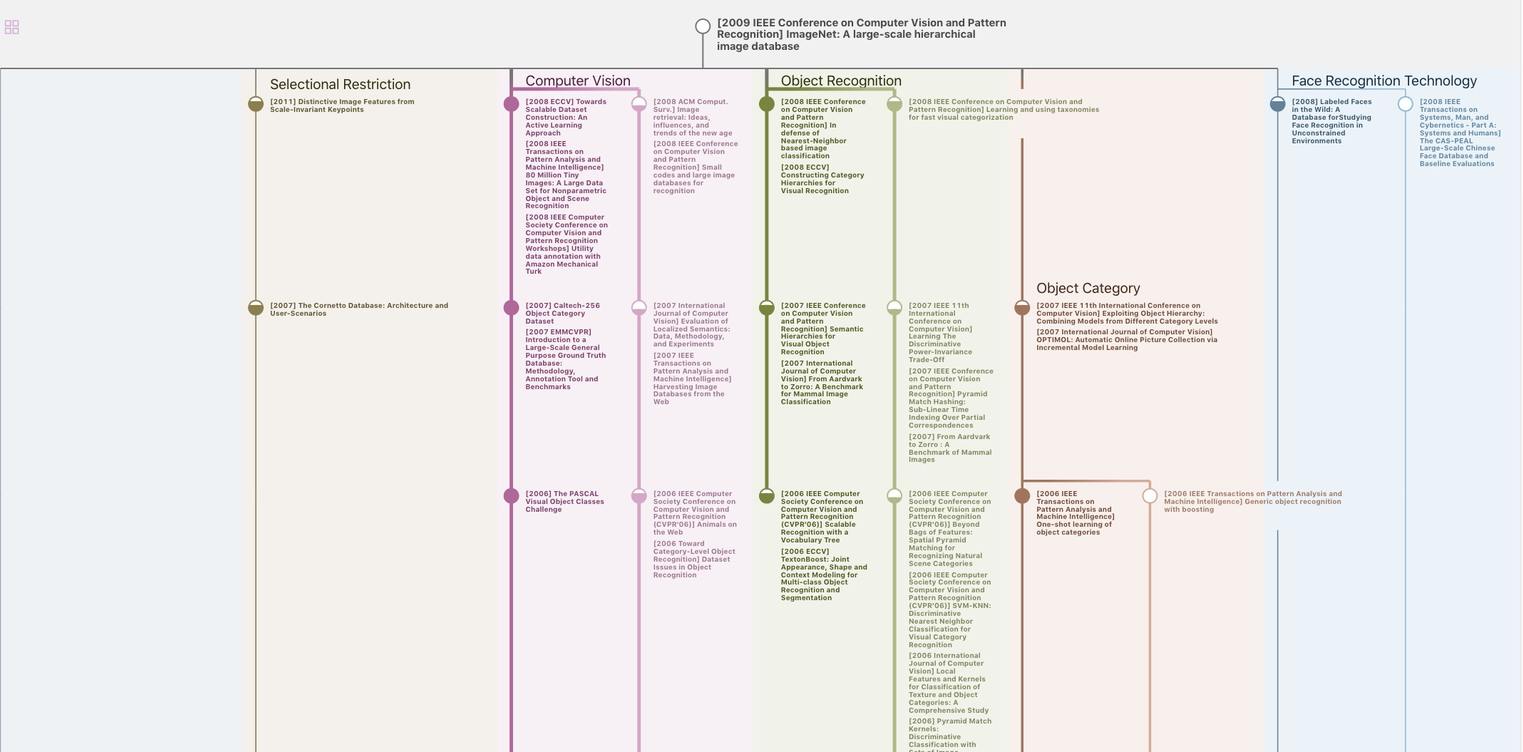
生成溯源树,研究论文发展脉络
Chat Paper
正在生成论文摘要