Reducing Hallucinations in Entity Abstract Summarization with Facts-Template Decomposition
CoRR(2024)
摘要
Entity abstract summarization aims to generate a coherent description of a
given entity based on a set of relevant Internet documents. Pretrained language
models (PLMs) have achieved significant success in this task, but they may
suffer from hallucinations, i.e. generating non-factual information about the
entity. To address this issue, we decompose the summary into two components:
Facts that represent the factual information about the given entity, which PLMs
are prone to fabricate; and Template that comprises generic content with
designated slots for facts, which PLMs can generate competently. Based on the
facts-template decomposition, we propose SlotSum, an explainable framework for
entity abstract summarization. SlotSum first creates the template and then
predicts the fact for each template slot based on the input documents.
Benefiting from our facts-template decomposition, SlotSum can easily locate
errors and further rectify hallucinated predictions with external knowledge. We
construct a new dataset WikiFactSum to evaluate the performance of SlotSum.
Experimental results demonstrate that SlotSum could generate summaries that are
significantly more factual with credible external knowledge.
更多查看译文
AI 理解论文
溯源树
样例
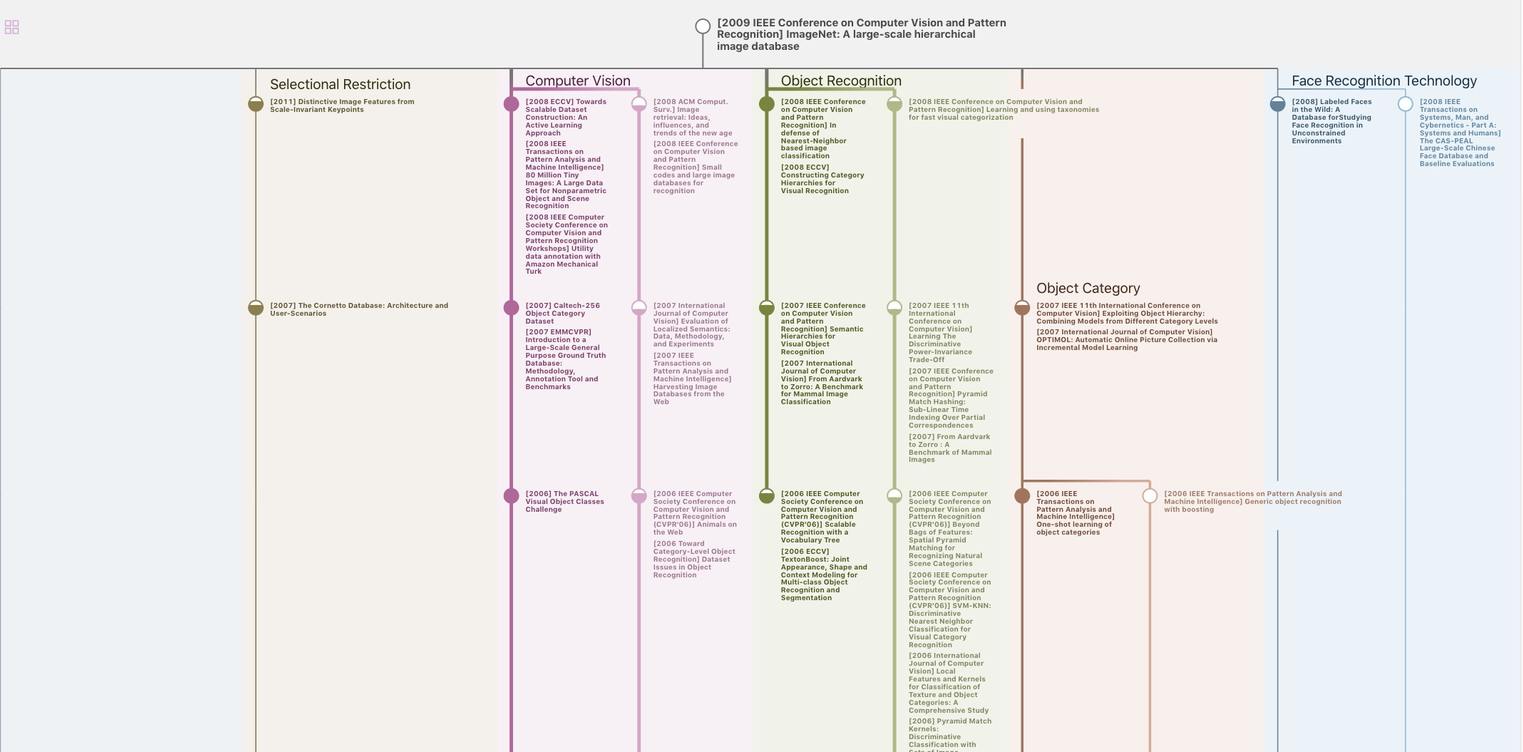
生成溯源树,研究论文发展脉络
Chat Paper
正在生成论文摘要