Detecting Anomalies in Time Series Using Kernel Density Approaches
IEEE ACCESS(2024)
摘要
This paper introduces a novel anomaly detection approach tailored for time series data with exclusive reliance on normal events during training. Our key innovation lies in the application of kernel-density estimation (KDE) to scrutinize reconstruction errors, providing an empirically derived probability distribution for normal events post-reconstruction. This non-parametric density estimation technique offers a nuanced understanding of anomaly detection, differentiating it from prevalent threshold-based mechanisms in existing methodologies. In post-training, events are encoded, decoded, and evaluated against the estimated density, providing a comprehensive notion of normality. In addition, we propose a data augmentation strategy involving variational autoencoder-generated events and a smoothing step for enhanced model robustness. The significance of our autoencoder-based approach is evident in its capacity to learn normal representation without prior anomaly knowledge. Through the KDE step on reconstruction errors, our method addresses the versatility of anomalies, departing from assumptions tied to larger reconstruction errors for anomalous events. Our proposed likelihood measure then distinguishes normal from anomalous events, providing a concise yet comprehensive anomaly detection solution. The extensive experimental results support the feasibility of our proposed method, yielding significantly improved classification performance by nearly 10% on the UCR benchmark data.
更多查看译文
关键词
Time series anomaly detection,machine learning,neural networks,autoencoder,kernel density estimation
AI 理解论文
溯源树
样例
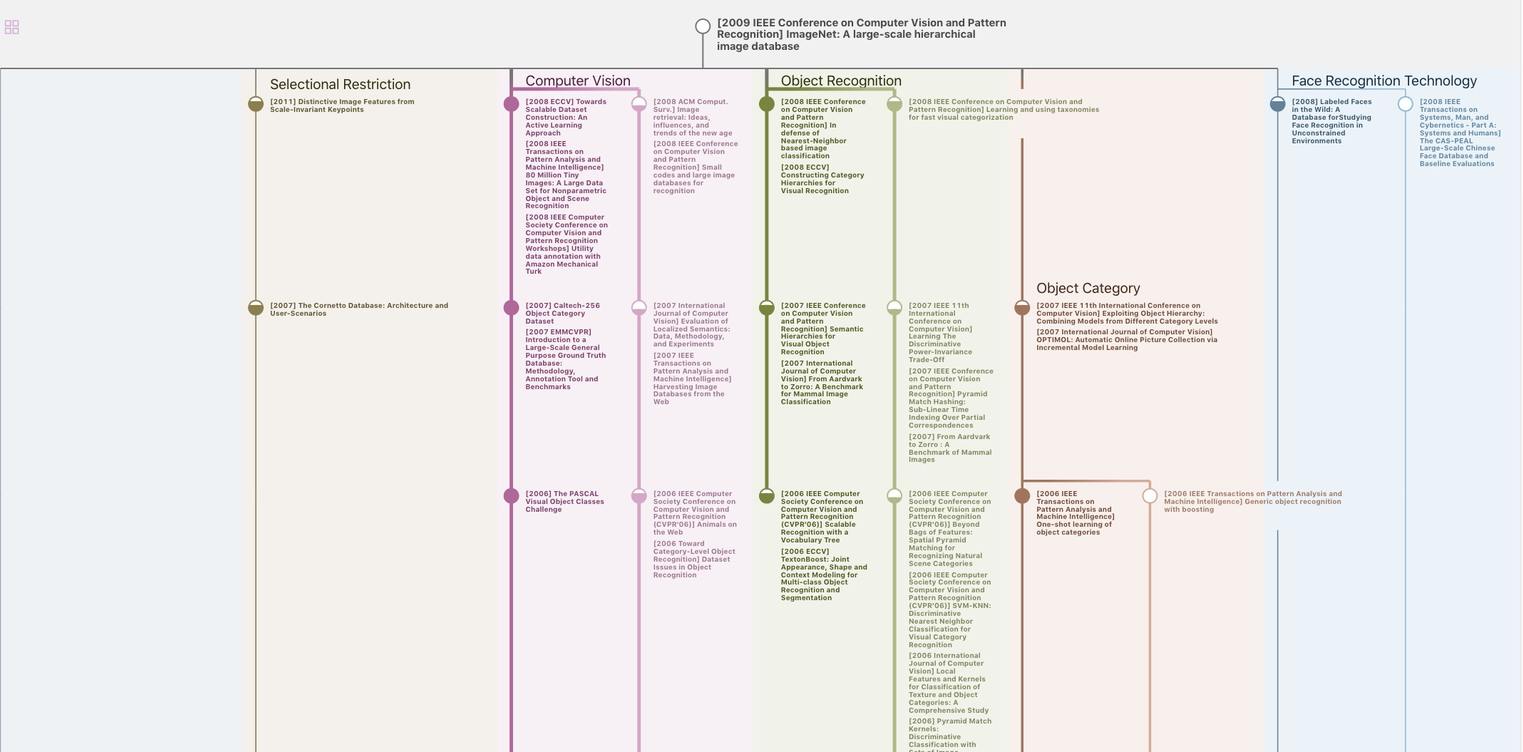
生成溯源树,研究论文发展脉络
Chat Paper
正在生成论文摘要