Synthesis of gadolinium-enhanced glioma images on multisequence magnetic resonance images using contrastive learning
MEDICAL PHYSICS(2024)
摘要
BackgroundGadolinium-based contrast agents are commonly used in brain magnetic resonance imaging (MRI), however, they cannot be used by patients with allergic reactions or poor renal function. For long-term follow-up patients, gadolinium deposition in the body can cause nephrogenic systemic fibrosis and other potential risks.PurposeDeveloping a new method of enhanced image synthesis based on the advantages of multisequence MRI has important clinical value for these patients. In this paper, an end-to-end synthesis model structure similarity index measure (SSIM)-based Dual Constrastive Learning with Attention (SDACL) based on contrastive learning is proposed to synthesize contrast-enhanced T1 (T1ce) using three unenhanced MRI images of T1, T2, and Flair in patients with glioma.MethodsThe model uses the attention-dilation generator to enlarge the receptive field by expanding the residual blocks and to strengthen the feature representation and context learning of multisequence MRI. To enhance the detail and texture performance of the imaged tumor area, a comprehensive loss function combining patch-level contrast loss and structural similarity loss is created, which can effectively suppress noise and ensure the consistency of synthesized images and real images.ResultsThe normalized root-mean-square error (NRMSE), peak signal-to-noise ratio (PSNR), and SSIM of the model on the independent test set are 0.307 +/-$\pm$ 0.12, 23.337 +/-$\pm$ 3.21, and 0.881 +/-$\pm$ 0.05, respectively.ConclusionsResults show this method can be used for the multisequence synthesis of T1ce images, which can provide valuable information for clinical diagnosis.
更多查看译文
关键词
attention mechanism,contrast enhanced MRI synthesis,contrastive learning,GBCAs,generative adversarial networks
AI 理解论文
溯源树
样例
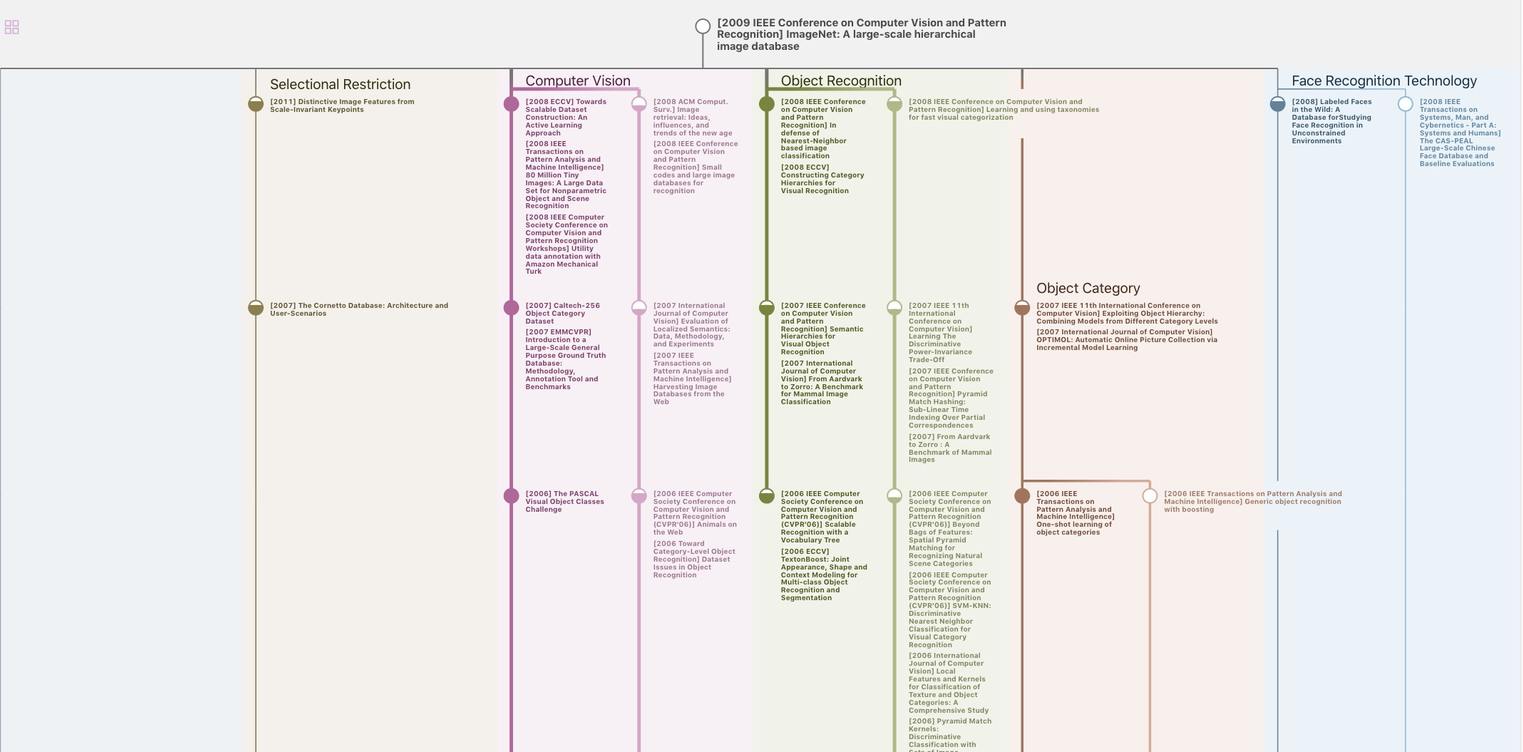
生成溯源树,研究论文发展脉络
Chat Paper
正在生成论文摘要