Refiner: a reliable and efficient incentive-driven federated learning system powered by blockchain
The VLDB Journal(2024)
摘要
Federated learning (FL) enables learning a model from data distributed across numerous workers while preserving data privacy. However, the classical FL technique is designed for Web2 applications where participants are trusted to produce correct computation results. Moreover, classical FL workers are assumed to voluntarily contribute their computational resources and have the same learning speed. Therefore, the classical FL technique is not applicable to Web3 applications, where participants are untrusted and self-interested players with potentially malicious behaviors and heterogeneous learning speeds. This paper proposes Refiner, a novel blockchain-powered decentralized FL system for Web3 applications. Refiner addresses the challenges introduced by Web3 participants by extending the classical FL technique with three interoperative extensions: (1) an incentive scheme for attracting self-interested participants, (2) a two-stage audit scheme for preventing malicious behavior, and (3) an incentive-aware semi-synchronous learning scheme for handling heterogeneous workers. We provide theoretical analyses of the security and efficiency of Refiner. Extensive experimental results on the CIFAR-10 and Shakespeare datasets confirm the effectiveness, security, and efficiency of Refiner.
更多查看译文
关键词
Federated learning,Blockchain
AI 理解论文
溯源树
样例
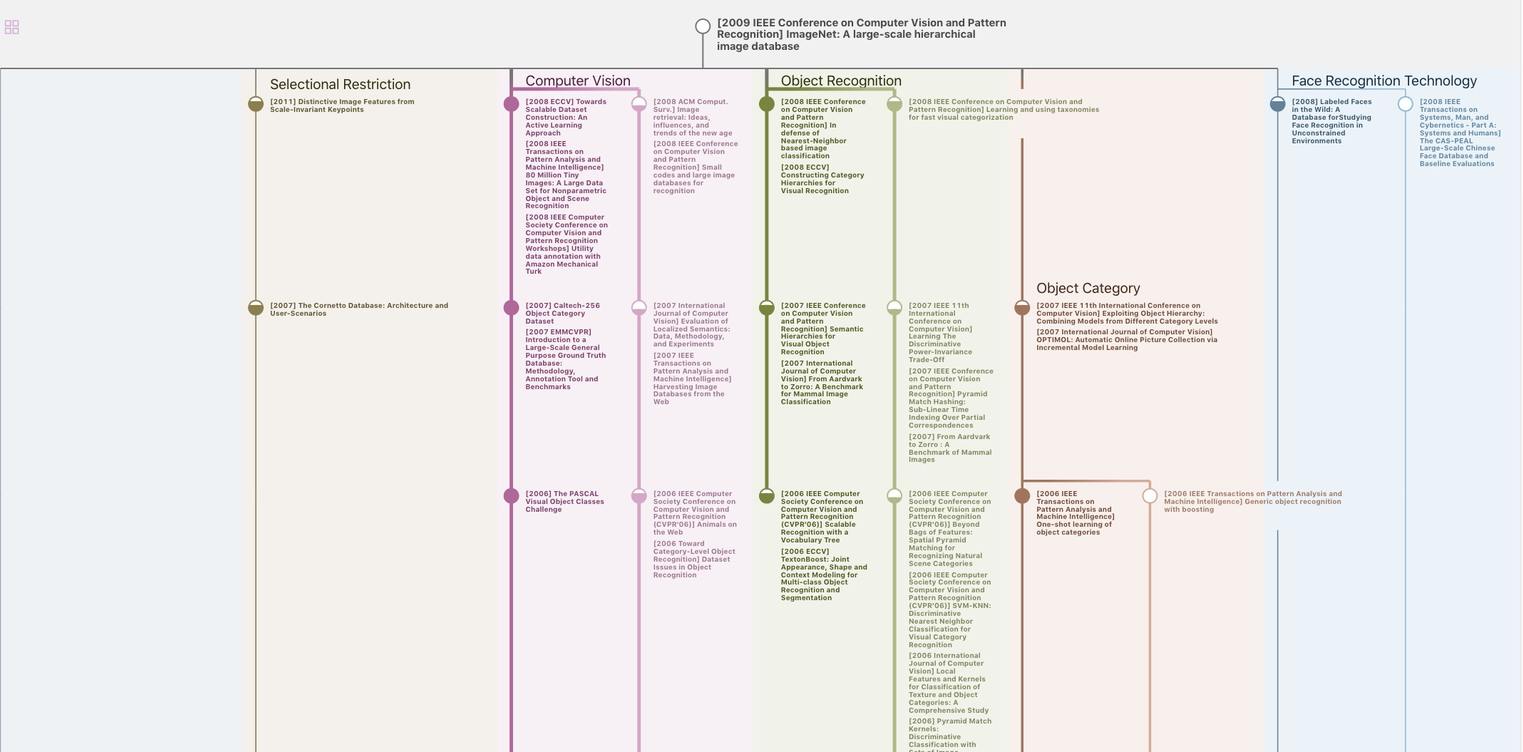
生成溯源树,研究论文发展脉络
Chat Paper
正在生成论文摘要