Enhanced Water Leakage Detection in Shield Tunnels Based on Laser Scanning Intensity Images Using RDES-Net.
IEEE J. Sel. Top. Appl. Earth Obs. Remote. Sens.(2024)
摘要
Tunnel water leakage detection is the difficulty and bottleneck in shield tunnel operation monitoring. This paper introduces the RDES method for detecting water leakage in shield tunnels. Firstly, to enhance the model's information extraction capabilities, we introduce a detection-efficient Efficient 3 Normalized Conv1ds of Attention (E3NCA) module by combining the characteristics of the attention mechanisms Efficient Channel Attention (ECA) and Coordinate Attention (CA). This module aims to focus the model's backbone network on capturing the spatial features of water leakage data and facilitating meaningful interaction between different channels. Additionally, we design the residual deformable convolution (RD2) to provide the convolutional energy with the flexibility to detect deformed targets, thereby enhancing the model's recognition ability. Lastly, we incorporate a Non-Maximum Suppression technique during prediction to improve detection accuracy. This technique retains more bounding boxes by applying Soft-NMS weighted averaging. The results of measured and publicly available data show that the RDES algorithm outperforms all the compared algorithms and is advanced and efficient. The results show that compared to the baseline, the RDES algorithm improves the F1 metrics, mAP0.5, mAP0.75, and mAP by 2.2%, 4.4%, 9.2%, and 5.2% on the laser intensity image dataset, and by 3.1%, 4.6%, 7.9%, and 5.9% on the optical image dataset.
更多查看译文
关键词
Water leakage detection,Laser intensity image,E3NCA,object detection,RD2
AI 理解论文
溯源树
样例
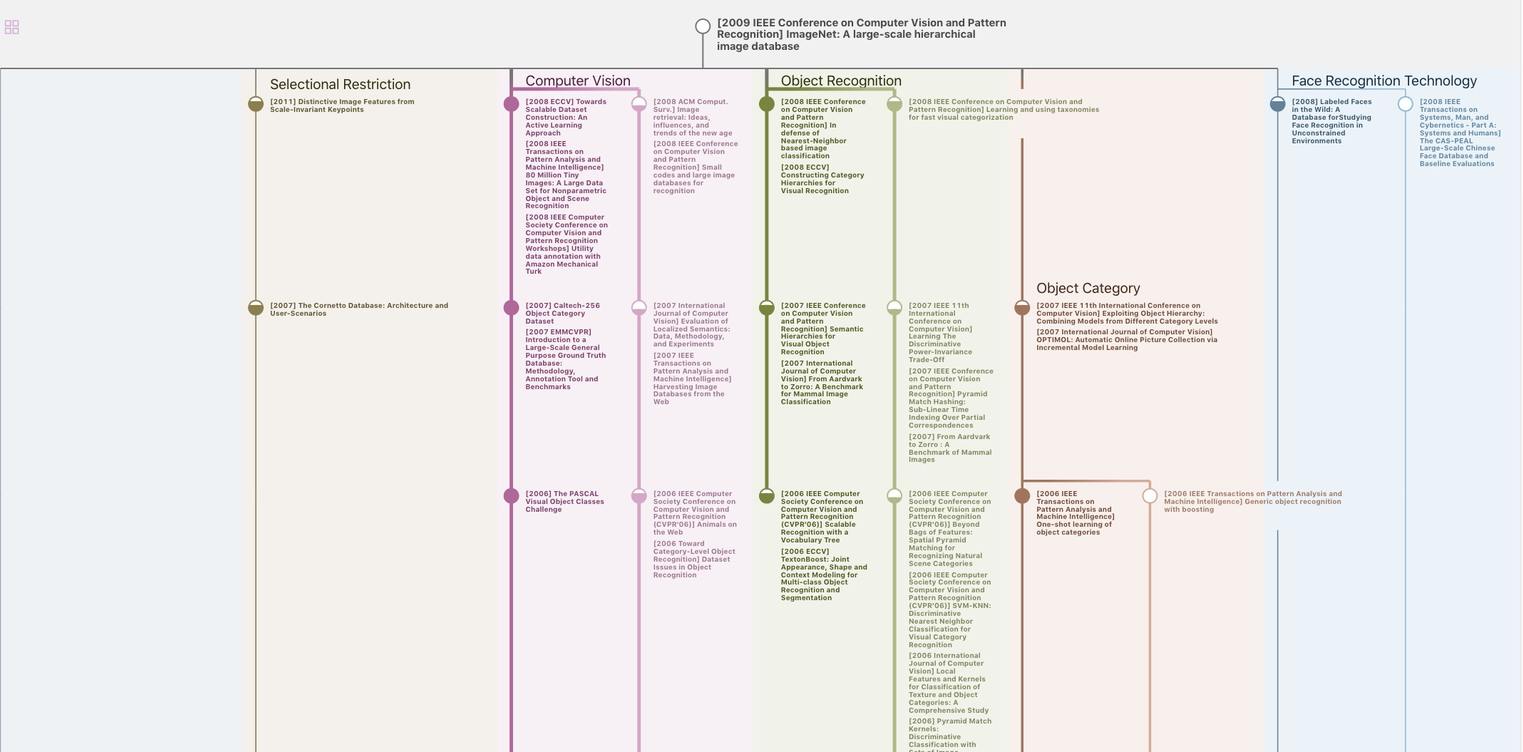
生成溯源树,研究论文发展脉络
Chat Paper
正在生成论文摘要