Graph Neural Network for Distributed Beamforming and Power Control in Massive URLLC Networks
IEEE Transactions on Wireless Communications(2024)
摘要
In this paper, we consider a massive ultrareliable and low-latency communication (mURLLC) network with multiple antennas at each transmitter. We formulate a distributed beamforming and power control problem by minimizing the logarithm-based average utility of decoding error probability for the worst link over different network topologies and channels, where the policy is represented by a graph neural network (GNN). To reduce signaling overhead and computation delay for distributed inference, we first develop a GNN for mURLLC (G4U) framework, where the graph embedding of each node is updated according to its previous graph embedding. In addition, we represent the local message of each node by the amplitude and phase of its pilot signal, such that the graph convolution can be accomplished efficiently by broadcasting the pilot signals. To further reduce the overall latency, we propose the pipeline G4U (PG4U), where each node determines its policy solely based on the channel state information acquired in the previous frames. The feedforward neural networks in PG4U for graph convolution can be executed efficiently during data transmission. To train the GNNs in mURLLC where the decoding error probability is small, we develop a novel loss function based on the asymptotic expression of the Gaussian
Q
-function. Simulation results show that G4U and PG4U are scalable to a different number of links. They can outperform the existing GNN and other policies significantly in terms of the QoS outage probability. Moreover, PG4U is suitable for mURLLC networks with short frame durations and highly correlated channels, while G4U is suitable for moderate frame durations with low channel correlation coefficients.
更多查看译文
关键词
Graph neural network,beamforming,power control,ultrareliable and low-latency communication,distributed algorithms
AI 理解论文
溯源树
样例
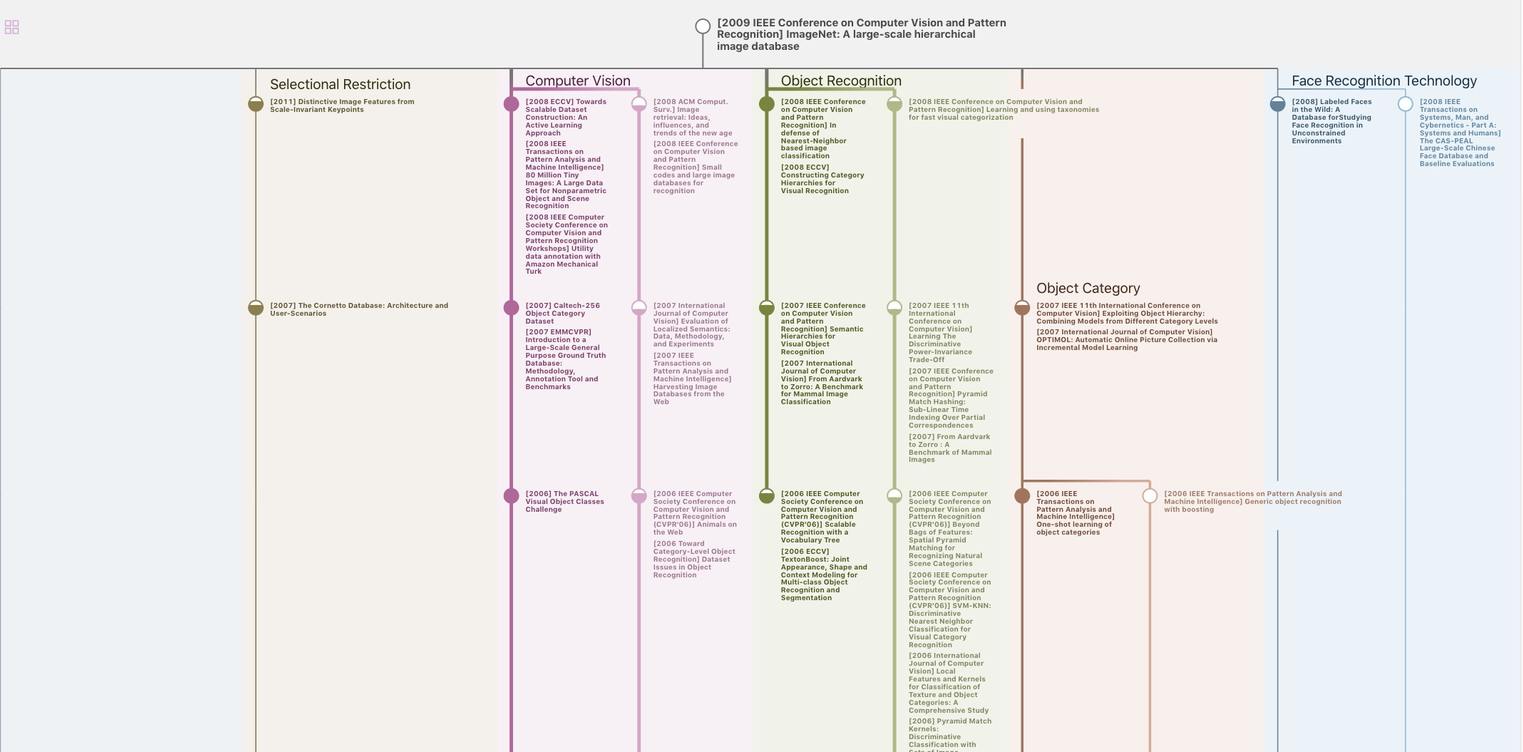
生成溯源树,研究论文发展脉络
Chat Paper
正在生成论文摘要