On the Understandability of MLOps System Architectures
IEEE Transactions on Software Engineering(2024)
We aimed to investigate informal textual descriptions and informal graphical MLOps system architecture representations and compare them with semi-formal MLOps system diagrams for those systems. We report on a controlled experiment with sixty-three participants investigating the understandability of MLOps system architecture descriptions based on informal and semiformal representations.
The results indicate that the understandability (quantified by task correctness) of MLOps system descriptions is significantly greater using supplementary semi-formal MLOps system diagrams, that using semi-formal MLOps system diagrams does not significantly increase task duration (and thus hinder understanding), and that task correctness is only significantly correlated with task duration when semi-formal MLOps system diagrams are provided.
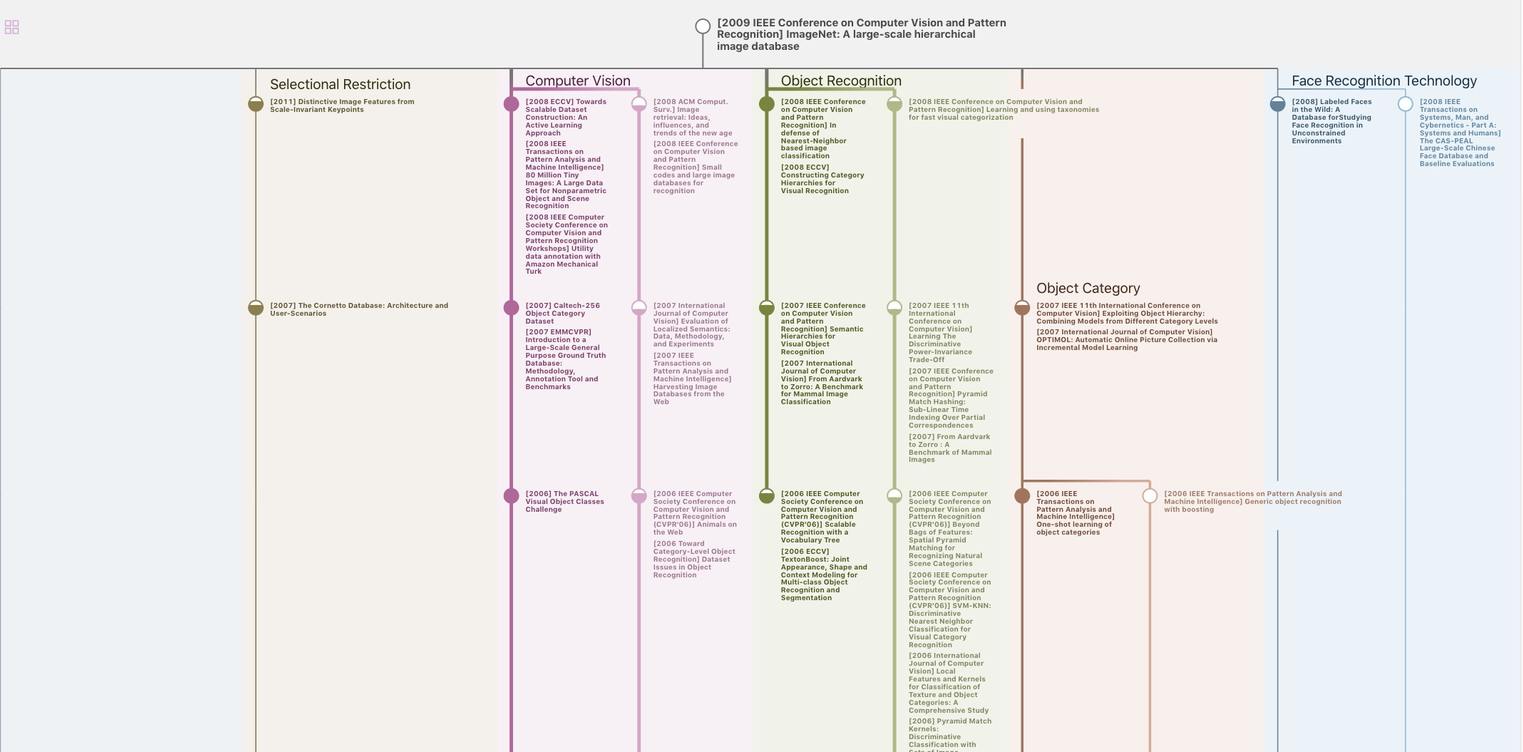