Deep Reinforcement Learning Assisted Hybrid Precoding for V2I Communications with Doppler Shift and Delay Spread
IEEE Transactions on Vehicular Technology(2024)
摘要
As a fundamental catalyst for intelligent transportation systems, the utilization of beyond-fifth-generation (B5G) technology in vehicle-to-everything (V2X) communication has garnered global attention. To tackle the performance challenge, the 3rd generation partnership project (3GPP) has stipulated in its Release 17 standard that the 5G new radio (NR) frequency range 2 (FR2, from 24GHz to 71GHz) can be employed for V2X communication. However, V2X communication, especially vehicle-to-Infrastructure (V2I) communication, is affected by the Doppler shift and delay spread, which becomes more pronounced as the transmission frequency rises. This presents additional obstacles for vehicle communication in the FR2. In this paper, we propose a deep reinforcement learning (DRL)-assisted hybrid precoding method for V2I communication on the 5G NR FR2 band, aming to strike a balance between complexity, reliability, and data rate, while considering the impact of strong Doppler shift and delay spread. Specifically, we present a downlink transmission model using massive multi-input multi-output (mMIMO) and hybrid precoding to assess the influence of these variables, leading to the evaluation of mismatch probability. Subsequently, a DRL-assisted hybrid precoding method is introduced, leveraging a multi-level codebook to optimize spectral efficiency without excessive complexity. Through rigorous data analysis, we highlight the benefit of incorporating RNNs within the DRL framework, enhancing training efficiency. A comparative study reveals the twin delayed deep deterministic policy gradient (TD3) model coupled with RNN outperforms other methods in terms of spectral performance.
更多查看译文
关键词
5G,vehicular communication network,precoding,Doppler shift,delay spread
AI 理解论文
溯源树
样例
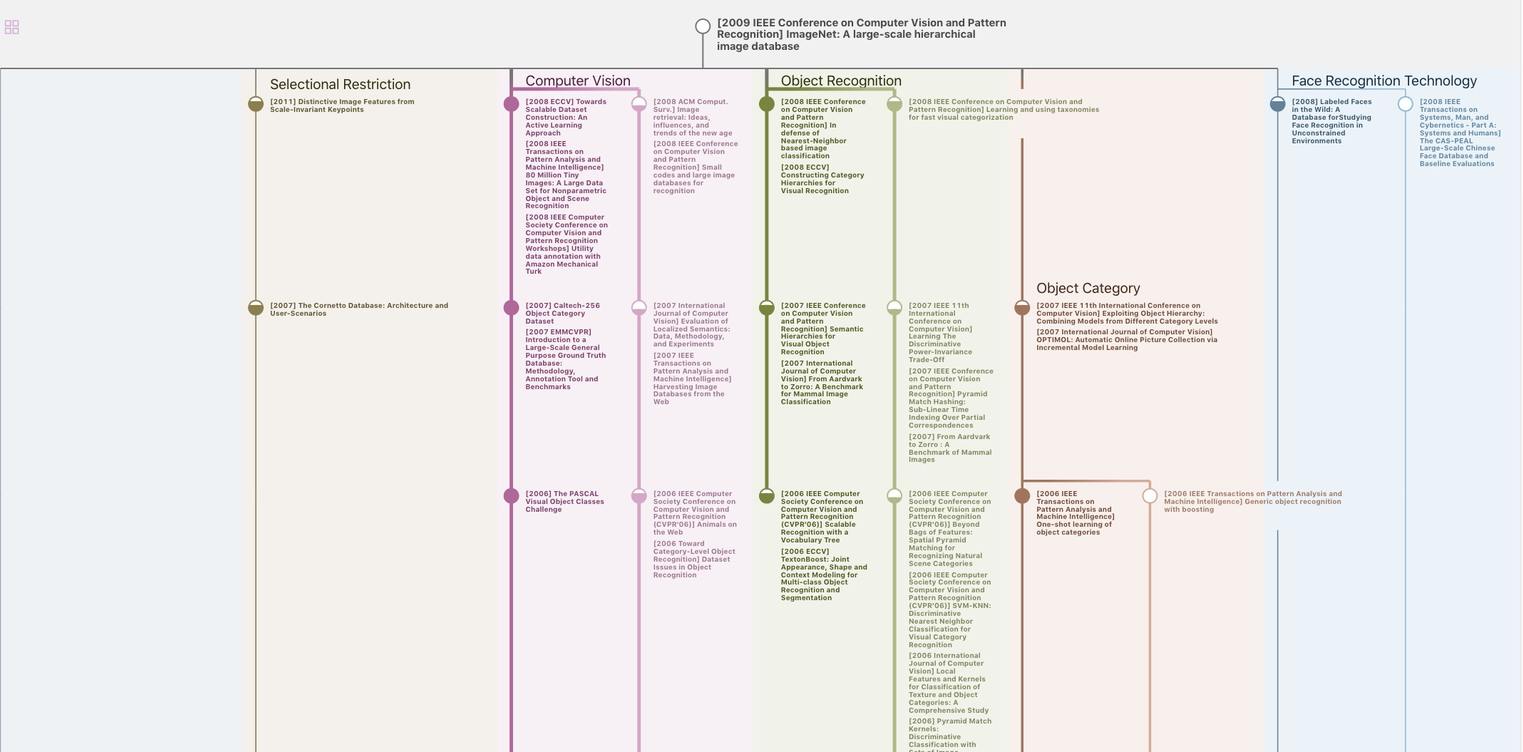
生成溯源树,研究论文发展脉络
Chat Paper
正在生成论文摘要