Joint Learning of Channel Estimation and Beamforming for Cell-Free Massive MIMO Systems
IEEE Wireless Communications Letters(2024)
摘要
In cell-free massive multiple-input multiple-output (MIMO) systems, beamforming is a crucial technique to enhance system performance. Nevertheless, practical challenges arise in obtaining accurate downlink channel state information (CSI), which plays a crucial role in effective beamforming design. Moreover, the limited fronthaul capacity hinders system performance improvement. In this letter, we propose a model-driven deep learning-based framework to obtain the optimal beamforming solution with unknown downlink CSI, comprising the neural network (NN) module and the beamforming recovery (BR) module. Specifically, the NN module jointly trains two neural networks with different functions to obtain downlink CSI and key features extracted from the beamforming matrix. According to the output of the NN module, the BR module recovers the beamforming matrix during the deployment phase. The simulation results demonstrate that the performance of the proposed method is close to that of the numerical algorithm and outperforms benchmark approaches, but the computational complexity is significantly reduced.
更多查看译文
关键词
Cell-free massive MIMO,beamforming,channel estimation,model-driven learning,joint training
AI 理解论文
溯源树
样例
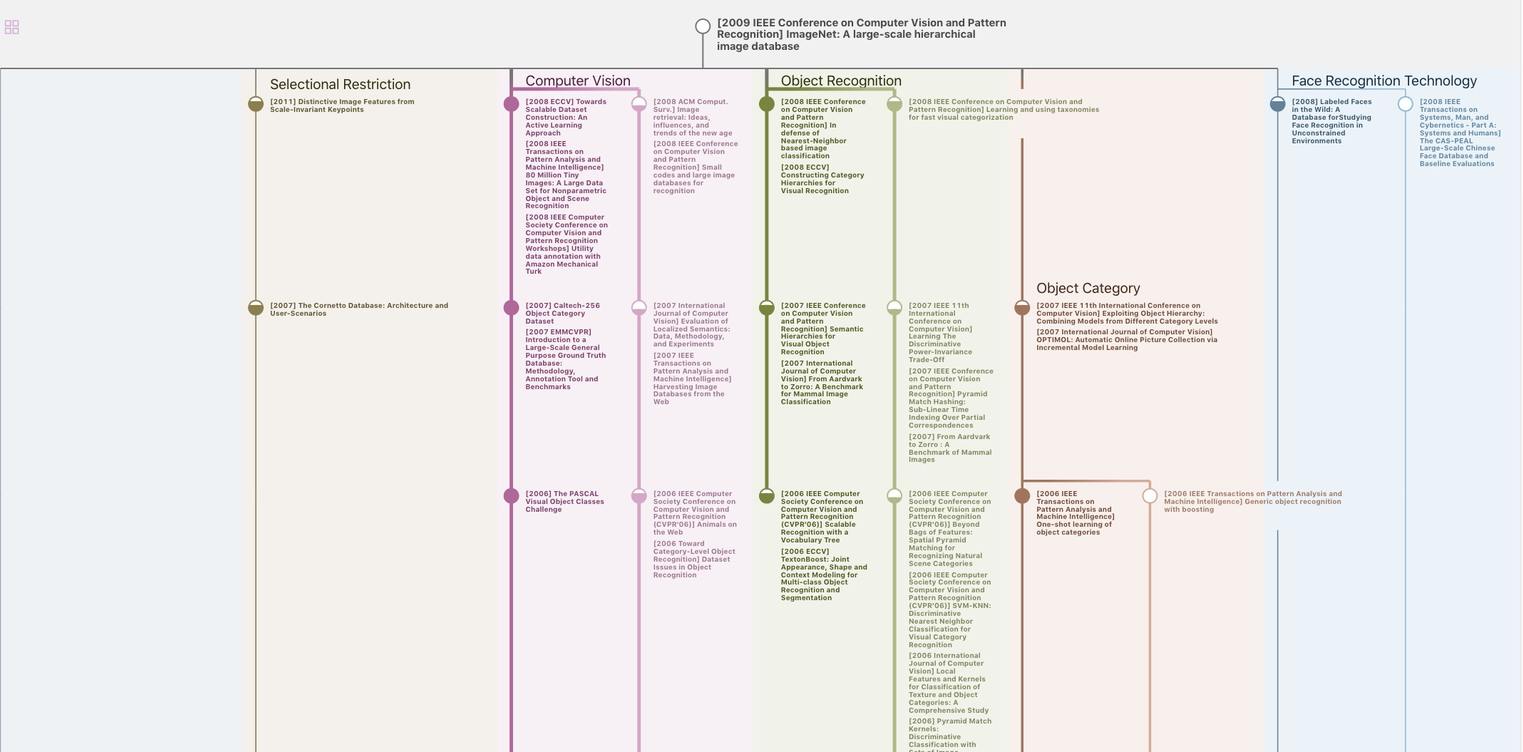
生成溯源树,研究论文发展脉络
Chat Paper
正在生成论文摘要