MASE: Multi-Attribute Source Estimator for Epidemic Transmission in Complex Networks
IEEE TRANSACTIONS ON SYSTEMS MAN CYBERNETICS-SYSTEMS(2024)
摘要
Identifying the transmission source based on network topology is of great significance for timely blocking migration from epidemic areas. To date, several sensor-based works have effectively focused on knowledge related to a single aspect, such as correlating the deterministic delay and observational delay or calculating the infection density. In this work, we study this problem from a new direction and develop a multi-attribute source estimator (MASE) and its extension Fuzzy-MASE. The proposed framework allows us to transform source localization into a multi-attribute decision-making (MADM) problem, ensuring better flexibility and extensibility. In addition, we propose a novel method, i.e., source dimension which can be taken as a supplementary attribute to MASE or used as an independent estimator called source dimension estimator (SDE). Crucially, we also study a previously underappreciated yet vital issue-how to effectively manage the influence of noisy observation sources. To broaden the generality and applicability of our research, we examine arbitrary graph structures rather than any specific configurations. As part of our validation, we have simulated diffusion on networks of different scales. The experimental results demonstrate the strengths of our proposed method over other existing methods in localization accuracy.
更多查看译文
关键词
Observers,Location awareness,Adaptation models,Noise measurement,Knowledge engineering,Diseases,Delays,Complex network,fuzzy theory,multi-attribute decision-making (MADM),source localization
AI 理解论文
溯源树
样例
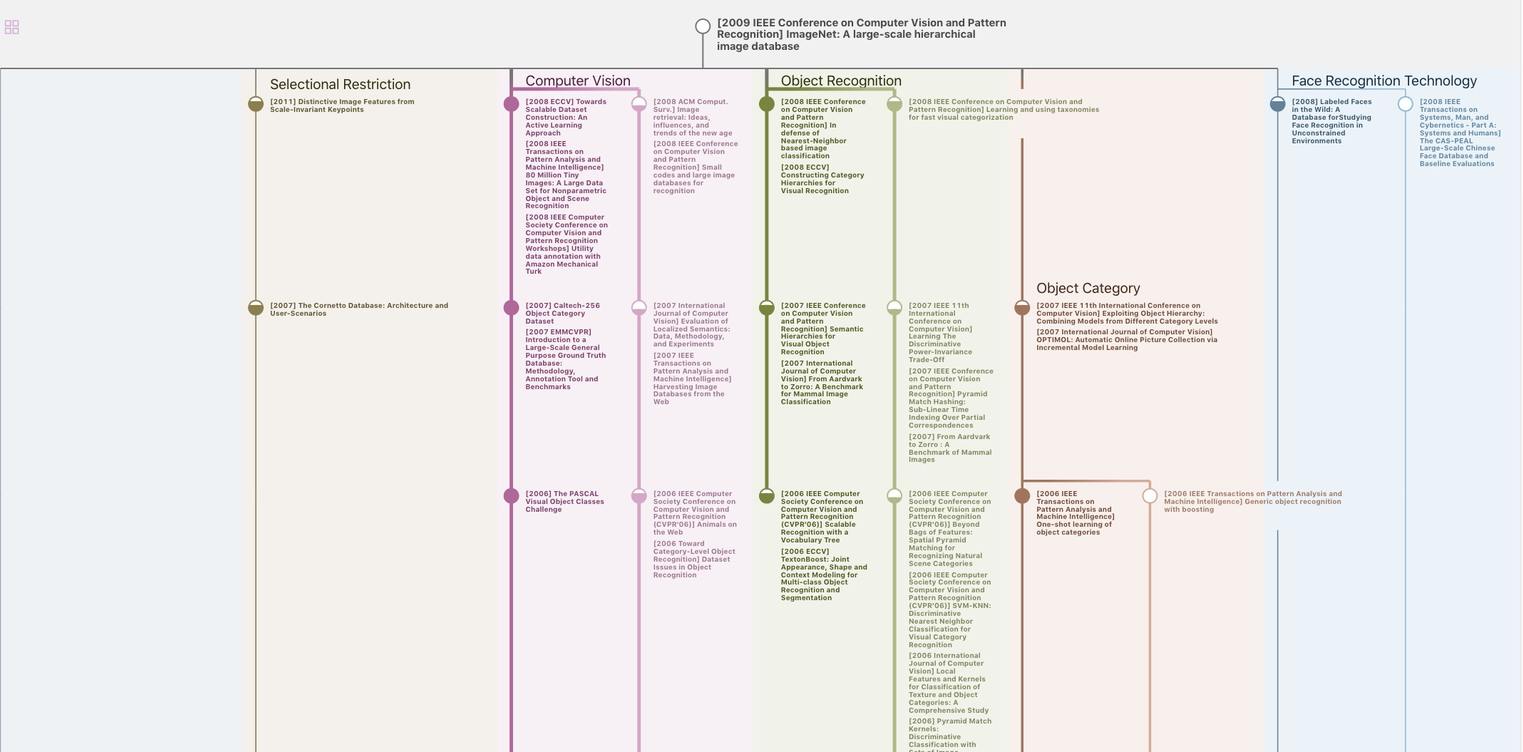
生成溯源树,研究论文发展脉络
Chat Paper
正在生成论文摘要