EMCNet: Ensemble Multiscale Convolutional Neural Network for Single-Lead ECG Classification in Wearable Devices
IEEE Sensors Journal(2024)
摘要
The rapid advancements in wearable devices have enabled continuous and real-time monitoring and analysis of physiological parameters. Owing to the benefits of detecting cardiovascular diseases, arrhythmia recognition from the wearable ECG (Electrocardiogram) signals has drawn much attention. In this paper, we present a novel method of using an ensemble of multiscale convolutional neural networks, the so-called EMCNet, for classifying the single-lead ECG waves acquired by wearable devices. The EMCNet includes two primary modules. Two component classifiers are first developed, which input the filtered ECG recordings and time-frequency spectrograms, and output the prediction probabilities of corresponding categories. These prediction probabilities are then sent to an improved weight matrix based on soft voting to produce the final classification result. Numerous experiments have been conducted, with results clearly demonstrating the efficacy and benefits of the EMCNet.
更多查看译文
关键词
ECG classification,Single-lead ECG,Ensemble learning,Internet of Medical Things
AI 理解论文
溯源树
样例
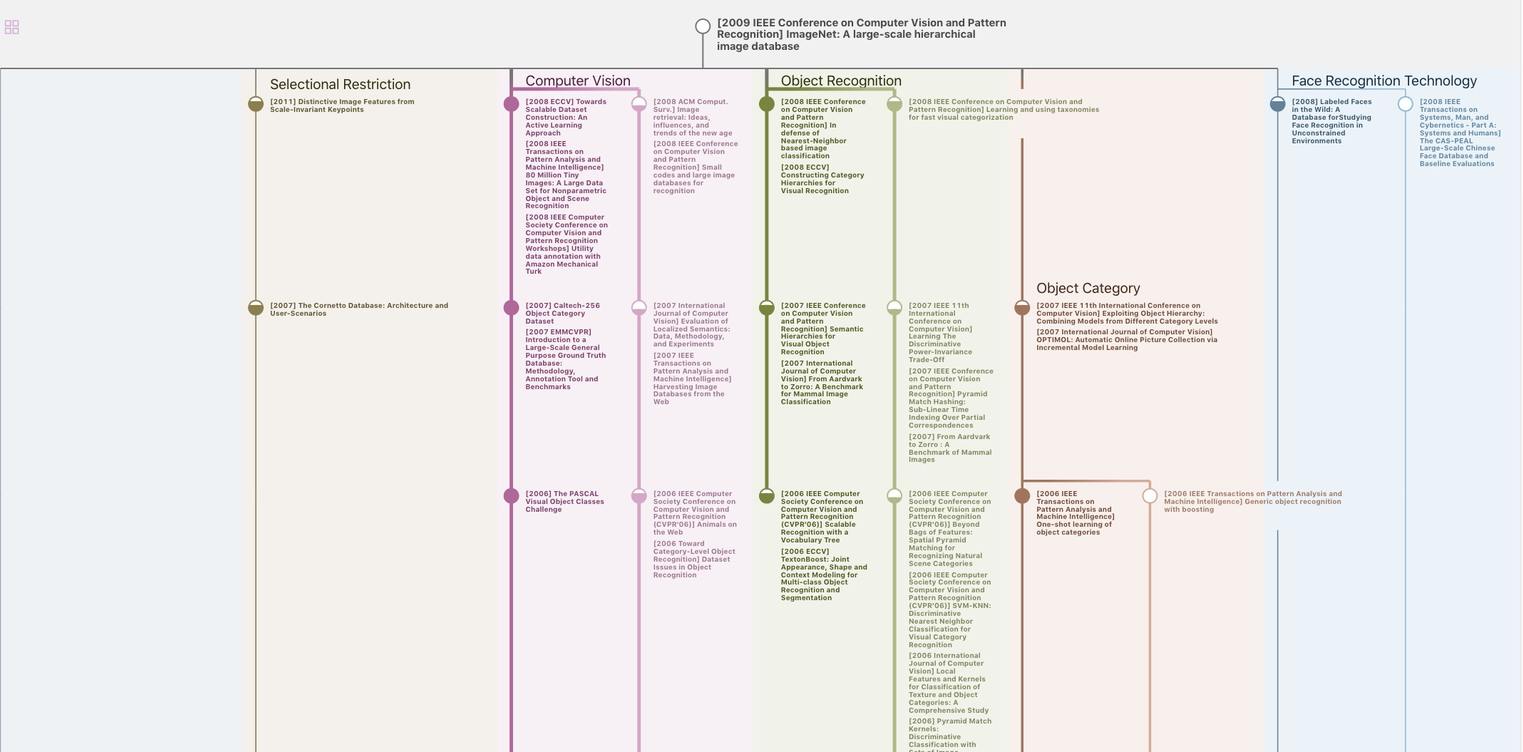
生成溯源树,研究论文发展脉络
Chat Paper
正在生成论文摘要