Human-Aware Dynamic Hierarchical Network Control for Distributed Metaverse Services.
IEEE J. Sel. Areas Commun.(2024)
摘要
Metaverse has emerged as a revolutionary technique for transforming the way people interact with digital content, which relies on a distributed computing and communication infrastructure, encompassing terminal users, edge servers, and cloud servers. However, the rapid evolution of the Metaverse presents challenges that surpass the capabilities of existing communication and network infrastructures, particularly on network bandwidth and latency. Additionally, human experience becomes a critical factor in this domain. Therefore, we introduce a human-aware hierarchical software defined network (SDN) architecture consisting of a Metaverse cloud layer, a mobile edge computing (MEC) server empowered edge layer, and a distributed terminal layer. Each MEC server dynamically controls a multi-antenna base station (BS) and several reconfigurable intelligent surfaces (RISs) according to the terminal immersive experience requirements in real-time. To overcome the bandwidth limitation, we propose a novel smart reconfigurable spatial reuse new radio in unlicensed spectrum (NR-U) framework, which can realize customizable communications through flexibly and coordinately reconfiguring beams among the coordination between BSs and RISs. The objective function is formulated as a Lyapunov optimization based decentralized partially-observable Markov decision process (Dec-POMDP) problem to maximize the spectral efficiency while guaranteeing the latency and reliability requirements in Metaverse, via a joint user selection, phase-shift control, and beam coordination strategy. To solve the above non-convex, strongly coupled, and mixed integer nonlinear programming (MINLP), we propose a novel multi-agent hierarchical deep reinforcement learning (MAHDRL) algorithm that integrates deep Q-network (DQN) to solve discrete problems, deep deterministic policy gradient (DDPG) to solve continuous problems, and mixing network to capture complex interactions between multiple agents. Numerical results demonstrate the effectiveness of the proposed algorithm and verify the performance improvements compared to traditional multi-agent deep reinforcement learning (MADRL) algorithms.
更多查看译文
关键词
Human-aware,dynamic network control,multi-agent,distributed coordination,NR-U,RIS,Metaverse
AI 理解论文
溯源树
样例
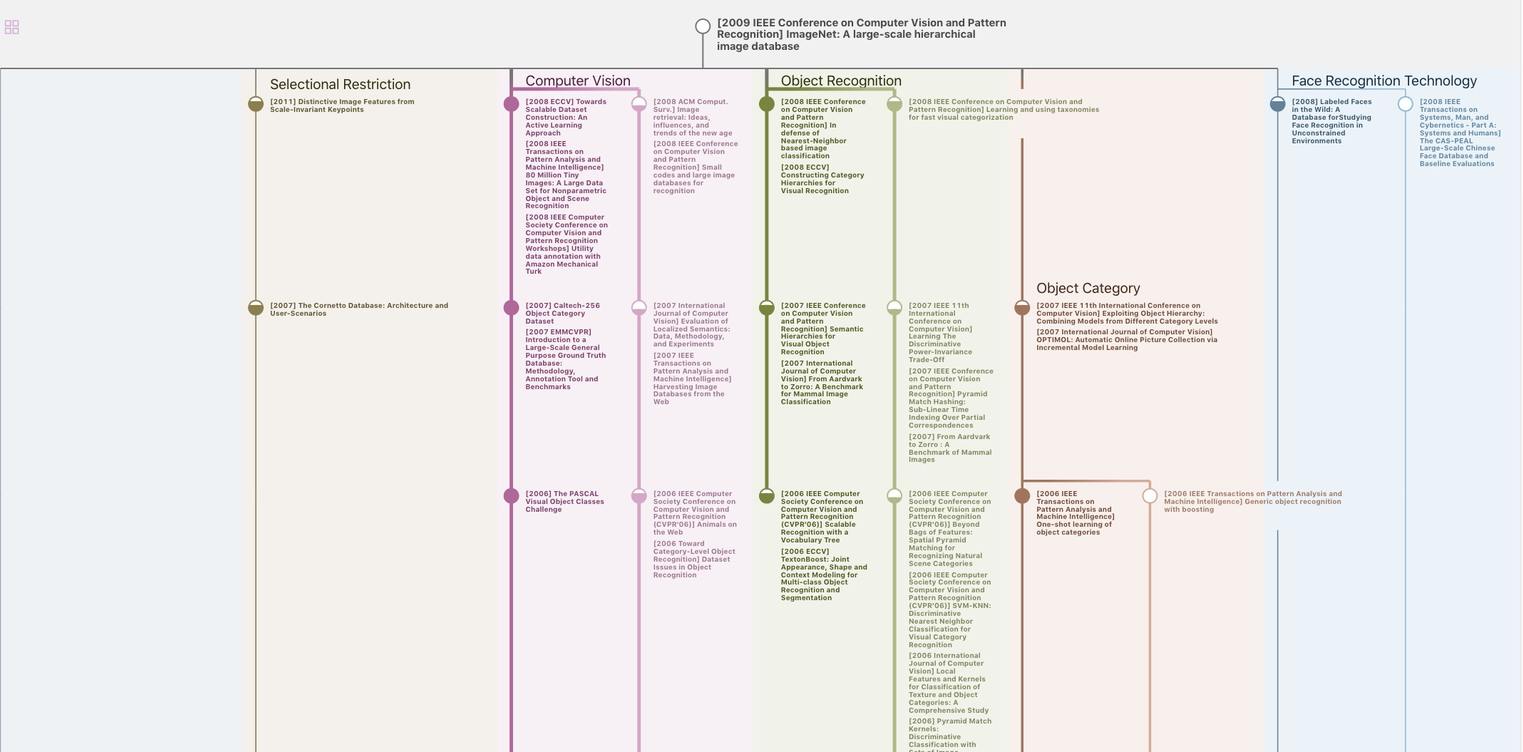
生成溯源树,研究论文发展脉络
Chat Paper
正在生成论文摘要