Improving Text-Based Person Retrieval by Excavating All-Round Information Beyond Color
IEEE TRANSACTIONS ON NEURAL NETWORKS AND LEARNING SYSTEMS(2024)
摘要
Text-based person retrieval is the process of searching a massive visual resource library for images of a particular pedestrian, based on a textual query. Existing approaches often suffer from a problem of color (CLR) over-reliance, which can result in a suboptimal person retrieval performance by distracting the model from other important visual cues such as texture and structure information. To handle this problem, we propose a novel framework to Excavate All-round Information Beyond Color for the task of text-based person retrieval, which is therefore termed EAIBC. The EAIBC architecture includes four branches, namely an RGB branch, a grayscale (GRS) branch, a high-frequency (HFQ) branch, and a CLR branch. Furthermore, we introduce a mutual learning (ML) mechanism to facilitate communication and learning among the branches, enabling them to take full advantage of all-round information in an effective and balanced manner. We evaluate the proposed method on three benchmark datasets, including CUHK-PEDES, ICFG-PEDES, and RSTPReid. The experimental results demonstrate that EAIBC significantly outperforms existing methods and achieves state-of-the-art (SOTA) performance in supervised, weakly supervised, and cross-domain settings.
更多查看译文
关键词
Task analysis,Image color analysis,Visualization,Semantics,Data models,Pedestrians,Learning systems,Color (CLR) information,cross-modal retrieval,frequency,person reidentification (ReID),text-based person retrieval
AI 理解论文
溯源树
样例
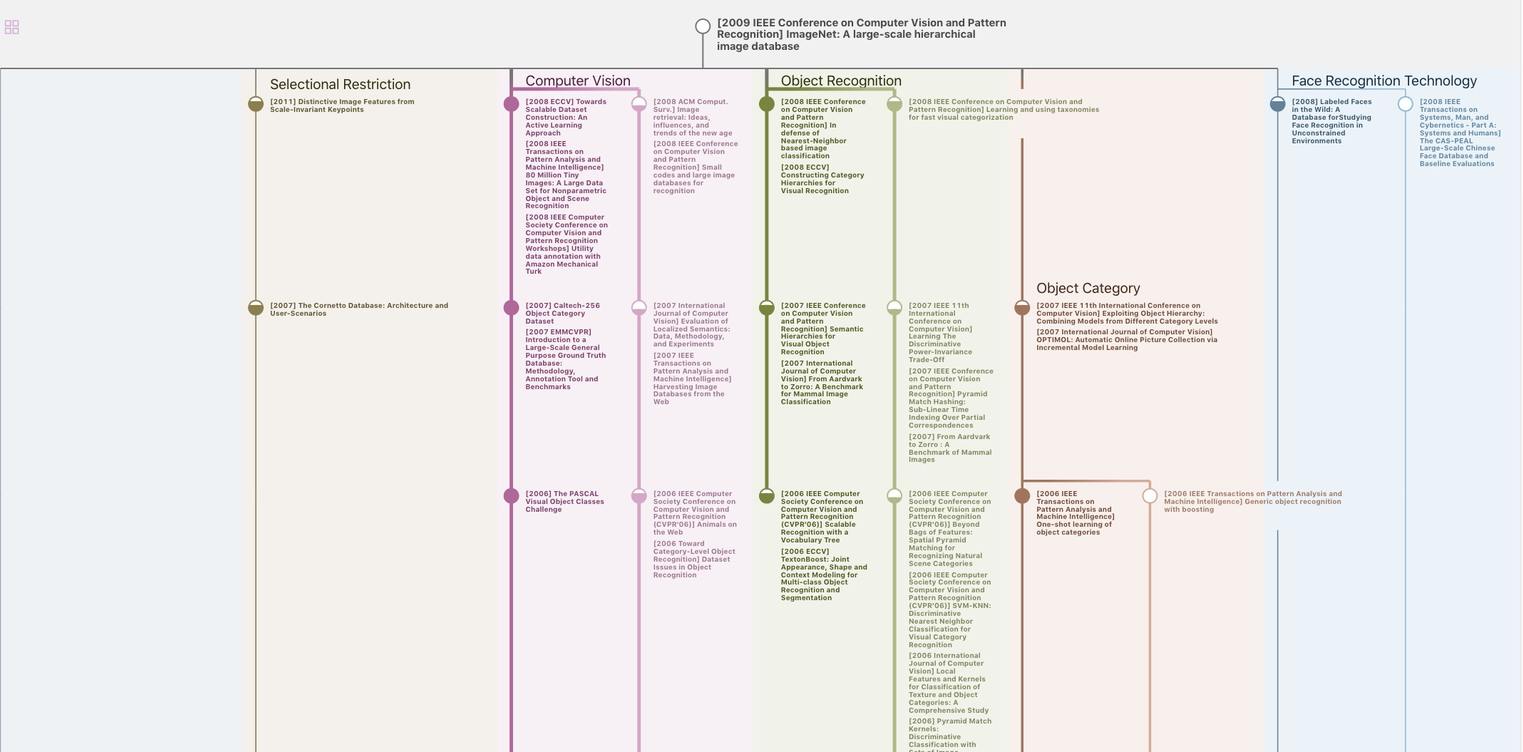
生成溯源树,研究论文发展脉络
Chat Paper
正在生成论文摘要