OptiTrack-aided Supervised Learning for Neural Network Based Ultra-wideband Ranging Bias Correction
IEEE Sensors Journal(2024)
摘要
Time-of-flight ranging using ultra-wideband (UWB) signals is of interest in many localization applications. However, non-line-of-sight (NLoS) and multipath radio propagation often lead to biased measurements. Even line-of-sight (LOS) UWB range measurements can exhibit spatially varying biases due to the relative pose and orientation of the UWB sensors or their antenna radiation patterns. This paper proposes a learning-based bias correction method to improve the measurement accuracy of UWB ranging measurements. Our contributions span across the architecture design of artificial neural networks (ANNs), identifying the informative features of the UWB signal to train the ANNs, and employing an OptiTrack motion capture camera system to collect a diverse set of training data in various relative poses between the sensors. Our neural network architecture consists of multiple ANNs to discriminate between LoS and NLoS measurements and to remove bias. To train ANNs, we focus on the signal features that are available on low-cost UWB ranging sensors and identify a novel set of features that enables our first ANN tier to distinguish between LoS and NLoS measurements with high accuracy. Consequently, subsequent ANNs can perform high-precision bias removal based on the identified measurement mode. Training data was collected using an OptiTrack system that measures the distances between a UWB transceiver mounted on a walking pedestrian and a set of stationary UWB transceivers in a continuum of various relative poses. A set of pedestrian location experiments showed that our bias removal method resulted in significantly improved localization accuracy.
更多查看译文
关键词
UWB ranging,OptiTrack,supervised learning,bias correction,neural network,localization
AI 理解论文
溯源树
样例
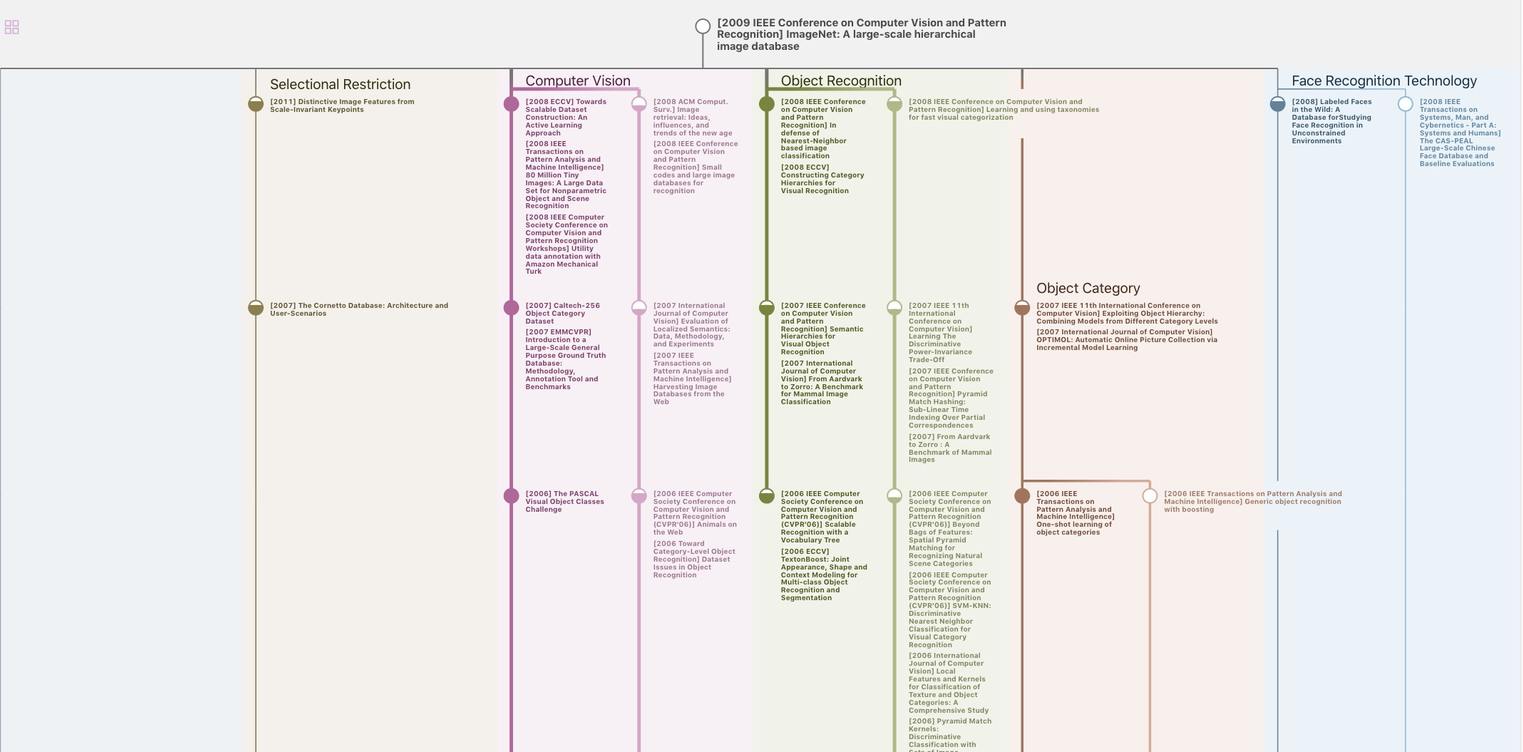
生成溯源树,研究论文发展脉络
Chat Paper
正在生成论文摘要