Privacy-Preserving Outsourcing Learning for Connected Autonomous Vehicles: Challenges, Solutions and Perspectives
IEEE Network(2024)
摘要
Although data sharing and fusion between connected autonomous vehicles (CAVs) can effectively enhance environment awareness and improve driving safety, it has to face severe challenges of privacy disclosure. Outsourcing encrypted data to edge servers for data analysis and model learning can alleviate this issue without imposing additional computing load on CAVs. In this article, we propose a privacy-preserving outsourcing learning (PPOL) framework based on lightweight additive secret sharing (ASS). Firstly, we propose a privacy-preserving outsourcing object detection method over secretly shared image and point cloud data. Secondly, we construct a privacy-preserving outsourcing depth estimation model over fused stereo image shares, aiming to provide a feasible solution for outsourcing data fusion and learning. Finally, we point out open issues under the PPOL framework, and give research perspectives in aspects of privacy-preserving data fusion for multi-frame, multi-modal and multi-view data, as well as privacy-preserving model optimization.
更多查看译文
关键词
Connected autonomous vehicles,privacy-preserving,outsourcing learning,data fusion,additive secret sharing
AI 理解论文
溯源树
样例
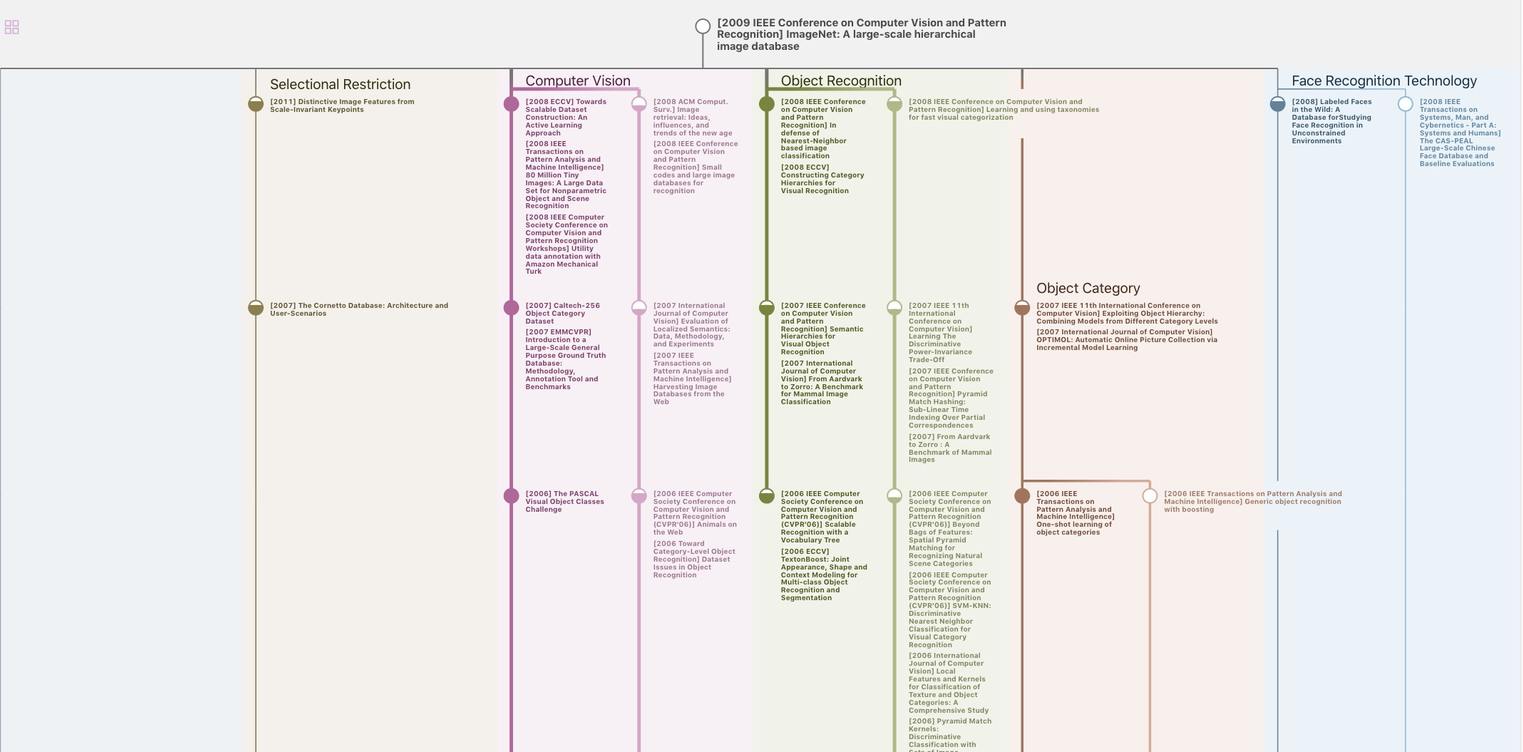
生成溯源树,研究论文发展脉络
Chat Paper
正在生成论文摘要