Decomposition and Meta-DRL based Multi-Objective Optimization for Asynchronous Federated Learning in 6G-Satellite Systems
IEEE Journal on Selected Areas in Communications(2024)
摘要
Wireless-based federated learning (FL), as an emerging distributed learning approach, has been widely studied for 6G systems. When the paradigm shifts from terrestrial to non-terrestrial networks (NTN), FL may need to address several open challenges, e.g., the limited service time of low earth orbit (LEO) satellites, the straggler issue in synchronous FL, and time-efficient uploading and aggregation for massive devices. In this work, we exploit the synergy of LEO and FL for future integrated 6G-satellite systems by taking advantage of ubiquitous wireless access provided by LEO and appealing characteristics of collaborative training and data privacy preservation in FL. The studied LEO-FL framework may need to improve multi-metric performance in practice. Different from most FL works, we simultaneously improve the communication-training efficiency and local training accuracy from a multi-objective optimization (MOO) perspective. To solve the problem, we propose a decomposition and meta-deep reinforcement learning based MOO algorithm for FL (DMMA-FL), aiming at adapting to the dynamic satellite-terrestrial environments, achieving efficient uploading and aggregation, and approaching Pareto optimal sets. Compared to single-objective optimization, heuristics-based, and learning-based MOO algorithms, the effectiveness and advantages of the proposed LEO-FL framework and DMMA-FL algorithm are assessed on MNIST and CIFAR-10 datasets.
更多查看译文
关键词
LEO satellite,asynchronous federated learning,multi-objective optimization,meta-reinforcement learning
AI 理解论文
溯源树
样例
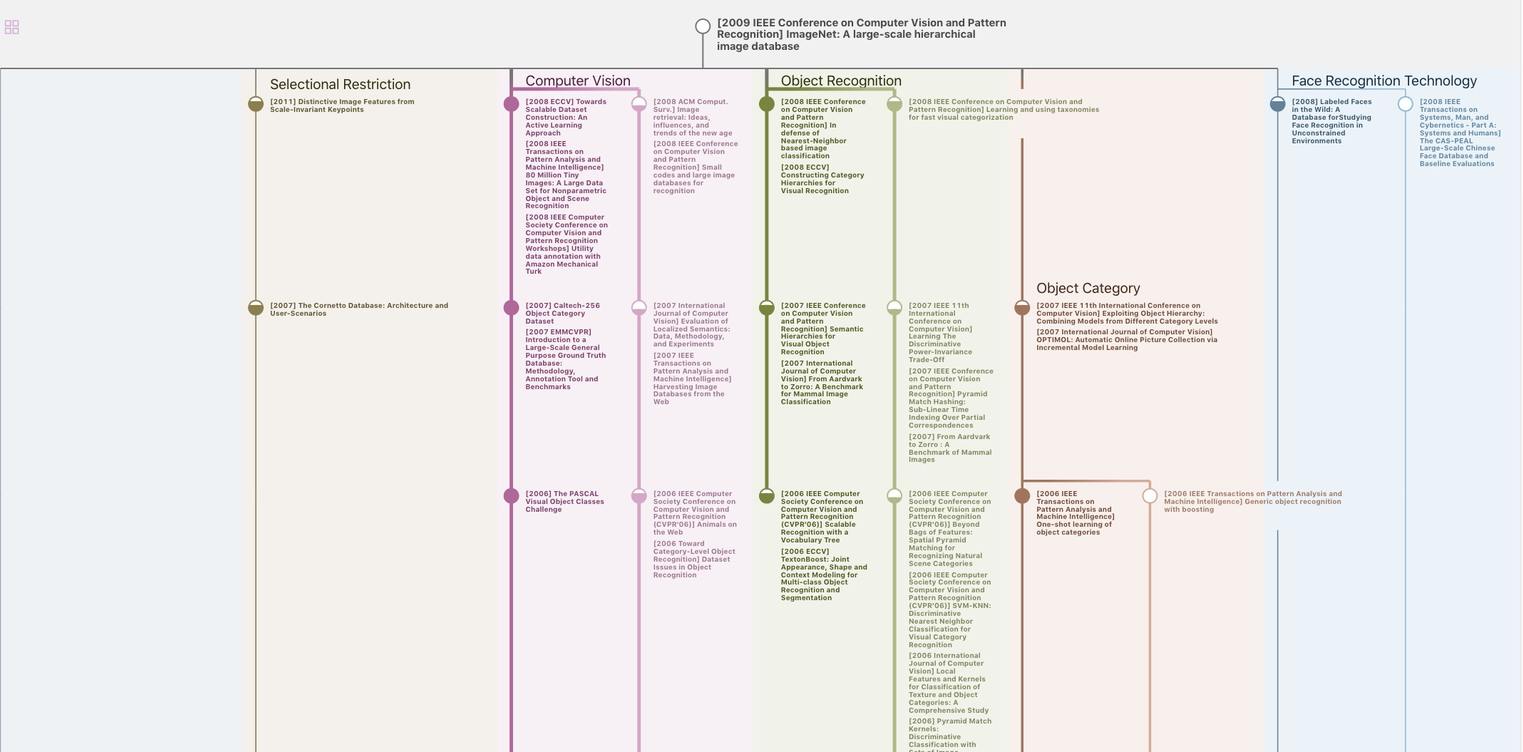
生成溯源树,研究论文发展脉络
Chat Paper
正在生成论文摘要