Semantically-Disentangled Progressive Image Compression for Deep Space Communications: Exploring the Ultra-low Rate Regime
IEEE Journal on Selected Areas in Communications(2024)
摘要
While sensing imagery in space missions has broad applications, the growing image resolution and data volume have caused a major challenge due to limited deep space channel capacities. To address this challenge, semantics-aware image compression becomes a promising direction. This paper is motivated to explore lossy compression at the ultra-low rate regime, which is a deviation from the high-fidelity- oriented tradition. Specifically, we propose an ultra-low rate deep image compression (DIC) codec by synthesizing multiple neural computing techniques such as style generative adversarial network (GAN), inverse GAN mapping, and contrastive disentangled representation learning. In addition, a residual-based progressive encoding framework is proposed to enable smooth transitions from the ultra-low rate regime to near- lossless regime. Experiments on the FFHQ and DOTA dataset demonstrate that compared with existing DICs, the proposed DIC can push the minimum rate boundary by about one order of magnitude while preserving the semantic attributes and maintaining a high perception quality. We further elaborate the design considerations for cross-rate-regime progressive DIC. Our study confirm that a semantically disentangled DIC holds the promise to bridge multiple rate regimes.
更多查看译文
关键词
Image compression,image semantics,deep neural network,progressive coding,deep space communication,generative adversarial network
AI 理解论文
溯源树
样例
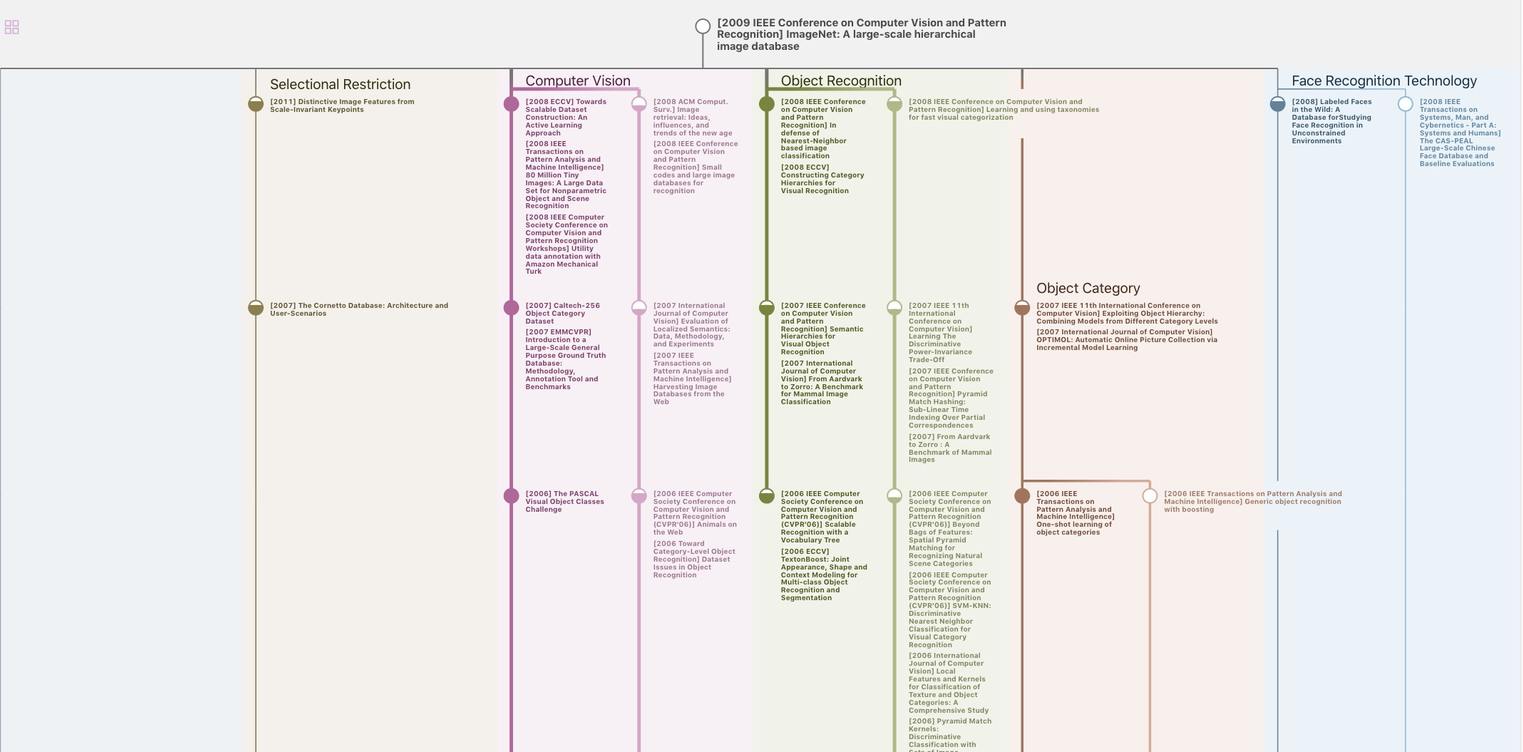
生成溯源树,研究论文发展脉络
Chat Paper
正在生成论文摘要