Domain-Aware Graph Network for Bridging Multi-Source Domain Adaptation
IEEE Transactions on Multimedia(2024)
摘要
Domain adaptation (DA) addresses the challenge of distribution discrepancy between the training and test data, while multi-source domain adaptation (MSDA) is particularly appealing for realistic scenarios. With the emergence of extensive unlabeled datasets, self-supervised learning has gained significant popularity in deep learning. It is noteworthy that multi-source domain adaptation and self-supervised learning share a common objective: leveraging unlabeled data to acquire more informative representations. However, conventional self-supervised learning encounters two main limitations. Firstly, the traditional pretext task falls to transfer fine-grained knowledge to downstream task with general representation learning. Secondly, the scheme of the same feature extractor with distinct prediction heads makes the cross-task knowledge exchange and information sharing ineffective. In order to tackle these challenges, we introduce a novel approach called Domain-Aware Graph Network (DAGNet). DAGNet utilizes a graph neural network as a bridge to facilitate efficient cross-task knowledge exchange. By employing a mask token strategy, we enhance the robustness of representations by selectively masking certain domain or self-supervised information. In terms of datasets, the uneven and style-based domain shifts in current datasets make it challenging to measure the model's domain adaptation performance in real-world applications. To address this issue, we introduce a benchmark dataset DomainVerse with continuous spatio-temporal domain shifts encountered in the real world. Our extensive experiments demonstrate that DAGNet achieves state-of-the-art performance not only on mainstream multi-source domain adaptation datasets but also on different settings within DomainVerse. Code is available at
https://github.com/a791702141/SSG
.
更多查看译文
关键词
Multi-source domain adaptation,self-supervised learning,graph neural network,real-world applications
AI 理解论文
溯源树
样例
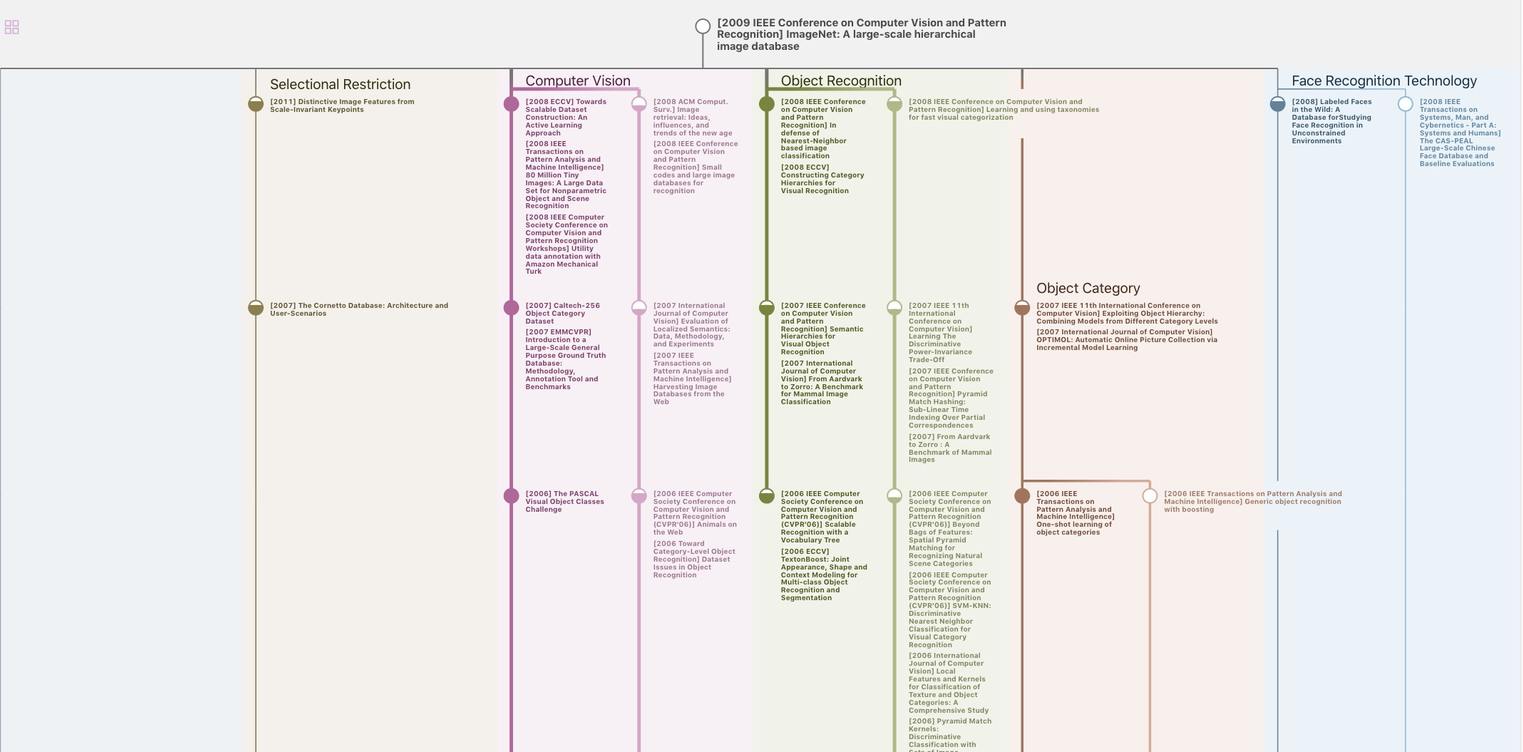
生成溯源树,研究论文发展脉络
Chat Paper
正在生成论文摘要